Think about peering into the intricate world of biomolecules, those complex assemblies that direct the symphony of life. Scientists have long relied on molecular dynamics (MD) simulations to watch them dance, but these often require costly quantum-mechanical calculations to get accurate results. However, a groundbreaking new technique called GEMS (general approach for constructing accurate machine-learned force fields for large-scale molecular simulations) developed by researchers from Google DeepMind, Germany, and collaborators may soon usher in a revolution in biomolecular research.
GEMS was designed with machine learning (ML), which gives it an unprecedentedly high level of accuracy when simulating huge biomolecules. This blog post delves into some of the nuances surrounding GEMS, its potential impact on understanding biomolecular dynamics, and its prospects for biological research and drug discovery going forward.
The Bottleneck of Biomolecular Simulation: Traditional Force Field
MD simulations are like a movie of the movements of biological molecules. It is necessary to have a set of rules known as force fields (FFs) that define how atoms interact with each other. Yet, traditional FFs often present simple approximations of these interactions at the cost of precision and speed. This inadequacy becomes more apparent when dealing with large and complex biomolecules such as proteins. In most cases, conventional FFs fail to capture the subtle intricacies in these delicate systems, leading to incorrect results that can be misleading.
GEMS: A Paradigm Shift with Machine Learning
GEMS provides an excellent alternative by combining machine learning expertise. The methodology is based on training an ML model using vast quantities of data from highly precise electronic-structure computations for fragments of small molecules. These fragments can be constituent units or related structures within the biomolecule, including water or solvent molecules, which are vital in determining the behavior.
The trick is in how GEMS uses this data. By studying these minute constituents, the machine learning model acquires an advanced understanding of the fundamental physics that governs their behavior. This consequently enables it to predict the potential energy surface (PES) of the whole biomolecule. PES shows an energy landscape for a molecule, which gives information about the most stable structures and the energetic transition cost between them. Then, with the correct PES, GEMS can simulate the dynamics of biomolecules to incredible precision.
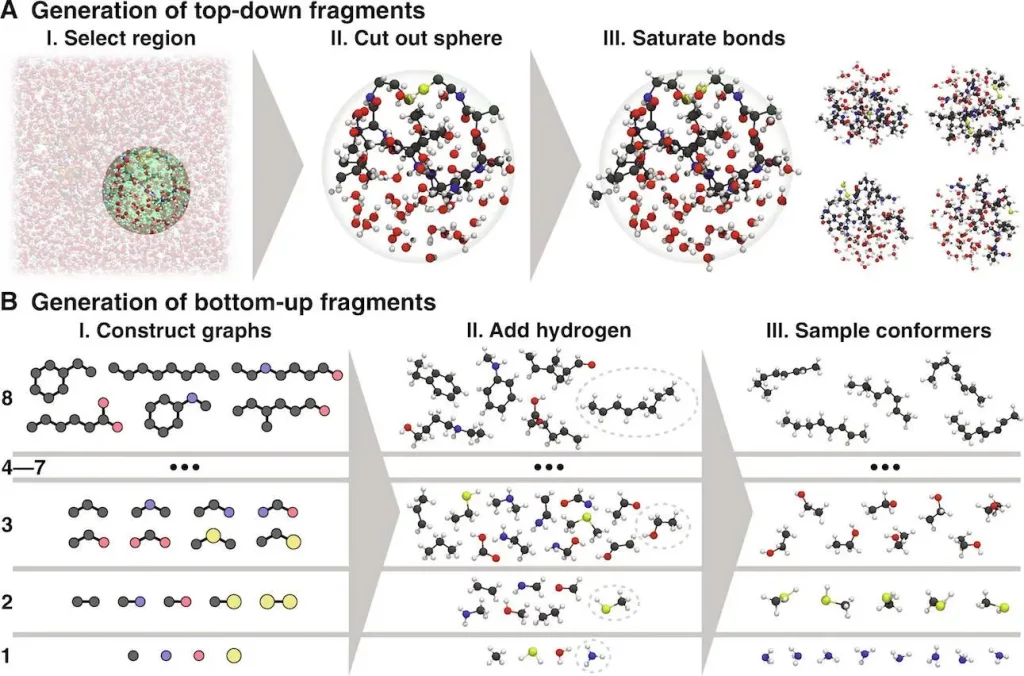
Image Source: https://doi.org/10.1126/sciadv.adn4397
GEMS Unmasked: From Peptides to Proteins
According to research by Unke et al., this application has been used successfully in simulating various biological systems. For example, in model peptides, simple amino acid chains constituting proteins’ building blocks, GEMS provides critical information about their folding process that was unknown. The work showed there are intermediate states during folding, which has important implications for how such peptides attain their final shapes.
However, peptides are not the only interest of GEMS. Researchers used crambin, a 46-residue protein dissolved in water molecules, as the test subject for GEMS. Simulations run by GEMS resulted in a different Feig-Grossman’s conventional FF protein landscape. In contrast to the slow, stiff motion, and jerky movements associated with traditional methods, which highlighted rigid structures and inflexible behaviors, GEMS brought out a more fluid yet smoother protein that vibrated gently. This implies that capturing the biomolecule’s dynamics with ab initio accuracy, the holy grail of MD simulations, might be crucial for a complete understanding of its function. GEMS has also been proven to be effective in reproducing experimental results on polyalanine helix stability and solvated crambin terahertz infrared vibrational spectrum. One thing that can be observed from this is how accurate and reliable GEMS is as it aligns with its real-world observations.
GEMS: Unlocking Life’s Hidden Mysteries
GEMS is a tool for precise biomolecular dynamics simulation, allowing unlimited possibilities in drug discovery. In the olden days, this method took a long time to complete because there was a need for repeated trials and errors involved in it. Virtual screening of potential drug molecules using GEMS can reveal how they interact with target biomolecules at the atomic level. Thus, predicting binding affinities and mechanisms of action will hasten the pace of discovering drugs, hence enhancing targeted therapies.
In addition to its applications in drug discovery, GEMS could potentially change our understanding of basic biological processes. The symphony of life must have something to do with biomolecular dynamics: protein folding or enzymatic catalysis, signal transduction, immune response, etc. As such, GEMS offers researchers a glimpse into this intricate ballet, enabling them to unravel nature’s secrets and spur the development of new biotechnologies.
The Road Ahead: Challenges and Opportunities with GEMS
Despite marking a milestone in biomolecular simulations, GEMS has its challenges. Here are some areas to focus on.
- Data Acquisition and Training: The training of the GEMS model demands a large amount of electronic-structure calculation results. However, generating this data for increasingly complex biomolecules still faces difficulties despite improvements in computational power. Better methods to acquire data more efficiently and optimize training processes will be instrumental for wider availability.
- Explainability and Interpretability: The inner workings of complex ML models such as GEMS can be difficult to discern; thus, how the model comes up with its predictions is unclear. Increasing the understandability and interpretability of GEMS will help researchers trust its findings and gain deeper insights from these simulations.
- Integration with Existing Workflows: Biomolecular research is highly dependent on well-established tools and workflows. Hence, it will be necessary to make sure that there is a seamless integration between these existing computational biology toolkits and databases with GEMS so that they can be adopted across science disciplines.
- Scalability and Computational Cost: Even though GEMS is a massive leap from what it was before, it is still computationally expensive to do really big simulations of thousands or millions of atoms. As technology develops rapidly, better algorithms will be developed to exploit GEMS’ full potential for simulating complex biological systems.
A Glimpse into the Future: The Advent of Bioinformatics
Despite this, there are good prospects ahead for GEMS and other machine-learning-based methods for biomolecular simulations. This way, scientists would eliminate the limitations and make GEMS one of the pillars of knowledge concerning life processes at the molecular level. Here are several possible ways forward:
- Personalized Medicine: To pave the way for tailoring medicine toward individual needs, GEMS may allow the modeling of biomolecule personalization. One can mimic how specific proteins or enzymes in a patient might interact with drugs to help prescribe medication that could be most effective while having minimum side effects.
- De Novo Protein Design: GEMS could enable researchers to design brand-new proteins with pre-determined functions. This could change the game in many diverse areas, such as biocatalysis, bioremediation, and developing novel biosensors and materials.
- Understanding Complex Diseases: Most diseases arise from non-functional protein-protein interactions. The GEMS may be a powerful tool for scientists to explore these interactions at an atomic level, which can help them understand disease mechanisms better and develop better therapies specific to diseases.
GEMS is, therefore, a major landmark in biomolecular simulations. It brings together mechanistic modeling with machine learning to provide unrivaled accuracy and details for understanding molecular-level life processes. By addressing the remaining challenges and improving the technology further, GEMS could reshape how we think about biological systems, hasten drug discovery efforts, and eventually offer us hope for a healthier future in medical fields.
Article Source: Reference Paper | All data used for analysis are present in the paper and/or the Supplementary Materials. DFT data are available at Zenodo.
Follow Us!
Learn More:
Anchal is a consulting scientific writing intern at CBIRT with a passion for bioinformatics and its miracles. She is pursuing an MTech in Bioinformatics from Delhi Technological University, Delhi. Through engaging prose, she invites readers to explore the captivating world of bioinformatics, showcasing its groundbreaking contributions to understanding the mysteries of life. Besides science, she enjoys reading and painting.