Transcriptomics has revolutionized our understanding of gene expression and its impact on biological processes. High-dimensional transcriptomics data provides researchers with much information, but analyzing such datasets can be challenging. However, an innovative method called hdWGCNA (high-dimensional weighted gene co-expression network analysis), developed by scientists from the University of California, Irvine, has emerged as a powerful tool for unraveling co-expression networks within these complex datasets. Their innovative technique involves generating “meta-cells” and holds promise for exploring a wide range of diseases throughout the body. The research findings were published in the journal Cell Press.
Understanding Co-expression Networks
Co-expression networks represent relationships between genes based on their expression patterns across conditions or tissues. Genes with similar expression profiles are likely involved in the same biological processes or regulatory pathways. Traditionally, co-expression networks were constructed using correlation-based approaches like Pearson correlation. However, these methods have limitations when applied to high-dimensional transcriptomics data. The new process, named hdWGCNA, improves on a technique called RNA bulk sequencing that’s widely used but does not address single-cell genomes.
hdWGCNA: An Overview
hdWGCNA overcomes challenges associated with traditional co-expression network analysis in high-dimensional transcriptomics data. It extends the weighted gene co-expression network analysis (WGCNA) framework to handle large-scale datasets efficiently.
The key innovation of hdWGCNA lies in its ability to handle high-dimensional data by leveraging advanced statistical techniques. It incorporates regularization methods like the elastic net to improve network construction accuracy and robustness. Additionally, hdWGCNA integrates feature selection algorithms to identify the most relevant genes contributing to the co-expression network.
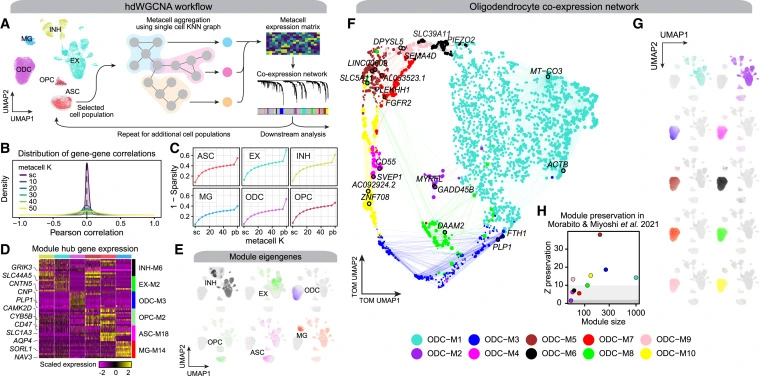
Advantages of hdWGCNA
- hdWGCNA excels at processing large-scale transcriptomics datasets with tens of thousands of genes. It reduces data dimensionality while preserving biologically meaningful relationships between genes.
- By incorporating regularization methods, hdWGCNA enhances the accuracy and robustness of co-expression network construction. It reduces noise introduced by high-dimensional data, resulting in more reliable network inference.
- hdWGCNA identifies gene modules—groups of co-expressed genes—that are functionally related. This information uncovers vital biological pathways and regulatory mechanisms underlying complex biological processes.
- Hub genes, highly connected within the co-expression network, play crucial roles in gene regulation and biological processes. hdWGCNA helps identify these hub genes, providing insights into potential targets for further investigation.
- hdWGCNA can identify condition-specific co-expression networks, revealing gene interactions that are specific to particular biological states or disease conditions. This knowledge enhances our understanding of context-dependent gene regulation.
- High-dimensional transcriptomics data often contains factors such as batch effects or technical variations. hdWGCNA incorporates methods to account for these factors, reducing their impact on co-expression network analysis and increasing the reliability of results.
- hdWGCNA can integrate transcriptomics data with other omics datasets, such as genomics, proteomics, or epigenomics. Integrative analyses allow researchers to uncover complex interactions between different molecular layers and identify key regulatory nodes that drive biological processes.
Applications of hdWGCNA
The versatility of hdWGCNA makes it a valuable tool in various biological research areas:
- Disease studies: hdWGCNA enables the identification of gene modules associated with specific diseases or conditions. Integrating clinical data can reveal potential biomarkers and therapeutic targets, facilitating precision medicine approaches. For example, in cancer research, hdWGCNA has been used to identify dysregulated gene modules that drive tumor progression and metastasis, leading to the discovery of novel therapeutic targets.
- Drug discovery: To develop effective therapeutics, it is essential to comprehend the complex interactions between genes and their response to drugs. hdWGCNA can identify genes involved in drug responses, unraveling molecular mechanisms and guiding the discovery of novel drug targets. Researchers can identify genes that show coordinated expression changes by analyzing co-expression networks in drug-treated cells or patient samples, potentially representing key players in drug response or resistance.
- Functional annotation: hdWGCNA aids in the functional annotation of genes by associating co-expressed gene modules with known biological processes or pathways. This information provides valuable insights into gene function and interaction networks. Researchers can prioritize genes for functional validation based on their membership in modules related to specific biological functions, accelerating our understanding of gene function in different contexts.
- Comparative genomics: Comparing co-expression networks across different species or experimental conditions can shed light on evolutionary conservation, uncover species-specific regulatory mechanisms, and identify conserved functional modules. hdWGCNA facilitates cross-species comparisons, allowing researchers to understand gene regulatory networks’ evolutionary dynamics and gain insights into the functional consequences of gene expression changes in different organisms.
- Integration of multi-omics data: hdWGCNA can integrate transcriptomics data with other omics datasets, such as genomics, proteomics, or epigenomics, to provide a comprehensive view of biological systems. Integrative analyses allow researchers to uncover complex interactions between different molecular layers and identify key regulatory nodes that drive biological processes.
- Biomarker discovery: hdWGCNA can identify gene modules associated with specific clinical outcomes or phenotypes. By analyzing co-expression networks in patient samples, researchers can identify gene modules that are differentially expressed between groups, potentially serving as biomarkers for diagnosis, prognosis, or treatment response.
Conclusion
hdWGCNA has emerged as a powerful method for unraveling co-expression networks in high-dimensional transcriptomics data. Its ability to handle large-scale datasets, construct robust networks, identify gene modules and hub genes, account for confounding factors, integrate different omics data types, and facilitate biomarker discovery has made it an invaluable tool in various areas of biological research. By deciphering the complex relationships within gene expression data, hdWGCNA helps uncover the underlying mechanisms driving biological processes, identify potential therapeutic targets, and advance our understanding of gene regulation. As high-throughput transcriptomics technologies continue to evolve, hdWGCNA will remain at the forefront, enabling researchers to unlock the hidden secrets within high-dimensional transcriptomics datasets and accelerate discoveries in molecular biology.
Article Source: Reference Paper | hdWGCNA Availability: R Package
Learn More:
Dr. Tamanna Anwar is a Scientist and Co-founder of the Centre of Bioinformatics Research and Technology (CBIRT). She is a passionate bioinformatics scientist and a visionary entrepreneur. Dr. Tamanna has worked as a Young Scientist at Jawaharlal Nehru University, New Delhi. She has also worked as a Postdoctoral Fellow at the University of Saskatchewan, Canada. She has several scientific research publications in high-impact research journals. Her latest endeavor is the development of a platform that acts as a one-stop solution for all bioinformatics related information as well as developing a bioinformatics news portal to report cutting-edge bioinformatics breakthroughs.