Seven Bridges Genomics, USA, scientists demonstrate an approach for iteratively augmenting tailored genome graphs for targeted populations on whole-genome samples of African ancestry.
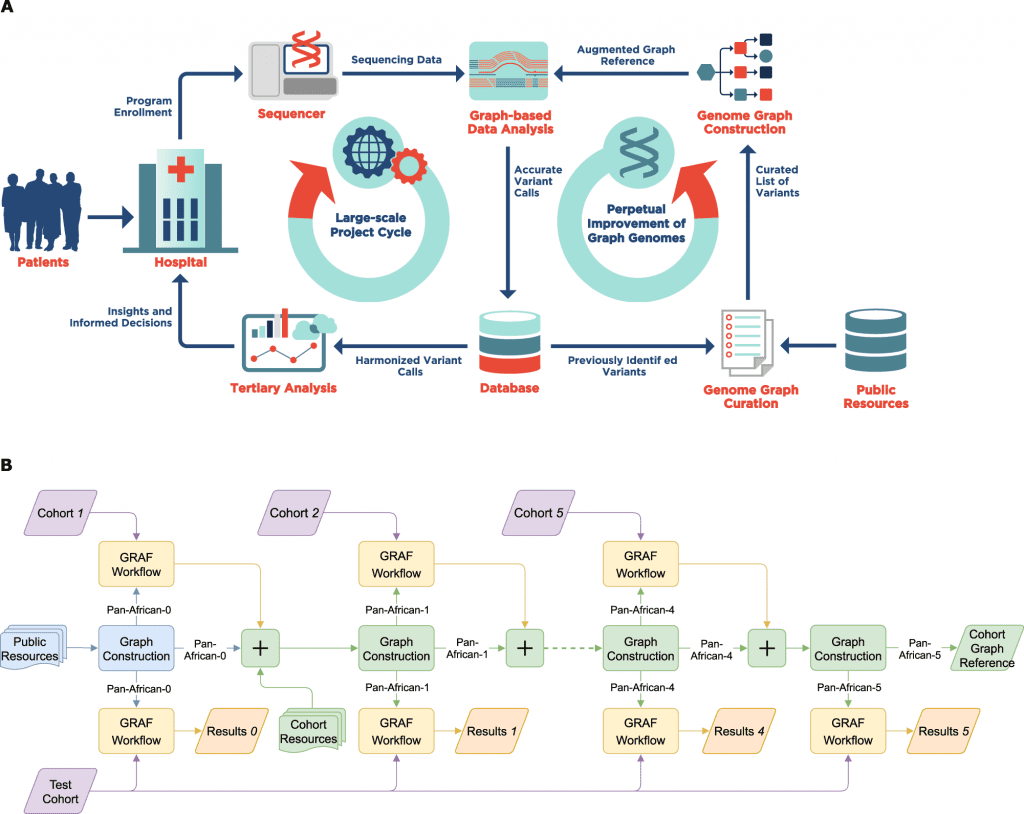
Image Source: https://doi.org/10.1038/s41467-022-31724-3
Due to the current human genome reference’s inadequacy in representing the varied genetic information from various human populations and its incapability to maintain the same level of accuracy for non-European ancestries, graph-based genome reference representations have undergone significant development.
Even though numerous attempts have been made to create computationally effective graph-based toolkits for NGS read alignment and variant calling, methods to curate genomic variants and subsequently construct genome graphs remain an unsolved issue that inexorably determines how well the bioinformatics pipeline functions as a whole.
In the given study, the scientists examine difficulties with graph building and suggest approaches for sample selection based on population variety, graph augmentation with structural variants, and resolving ambiguity in graph reference brought on by information overload.
Additionally, they argue in favor of iteratively enhancing customized genome graphs for target populations and show this method using whole-genome data with African heritage.
Their findings demonstrate that population-specific graphs, which are more representative than linear or generic graph references, can significantly reduce read mapping errors and improve variant calling sensitivity while providing joint variant calling improvements without requiring computationally demanding post-processing steps.
An Understudied Problem
The human genome reference is used by methods for next-generation sequencing (NGS) read alignment and variant calling to interpret the unprocessed data.
The genome reference essentially dictates the accuracy and reliability of these techniques.
The most recent version, GRCh38, is derived from a small number of people, with over 70% of the data related to only one person, and as a result, it is unable to accurately represent the genetic variety of the great majority of human populations.
Over the past ten years, numerous research has brought this issue to light. Numerous approaches, such as nucleotide additions and expansions to the current reference, have been suggested for incorporating a more comprehensive range of genetic information into the reference and de novo synthesis of unprocessed read data to provide a consensus sequence particular to a population, and graph-based references capable of simultaneously representing numerous distinct populations.
Accuracy, efficacy, and application trade-offs exist for each of these techniques. The selection of the right variation information to include in the reference, in addition to designing a proper data structure and acceptable algorithms to work with it, is a significant but understudied subject without an obvious solution.
Result Comparison by the Utilization of Joint Variant Calling
A novel genome reference and related bioinformatics tools should satisfy the following requirements to guarantee long-term utility and compatibility, particularly with large-scale sequencing projects:
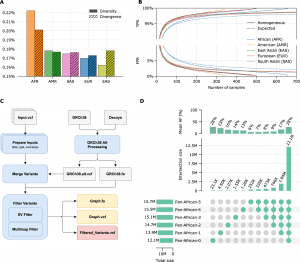
Image Source: https://doi.org/10.1038/s41467-022-31724-3
- Precise representation of various genetic data,
- Compatibility with current techniques and standards,
- Suitability for enhancements and other modifications,
- Computational effectiveness and scalability, and
- Adaptability to specific populations and/or applications
In this study, the scientists provide a population-specific graph creation method that satisfies all of these requirements and contrasts its applicability in variant calling, along with NGS read alignment to alternative methods.
They demonstrate that, while being computationally effective, a population-specific genome graph can dramatically increase read alignment and variant calling accuracy.
Furthermore, they demonstrate that genome graphs can be enhanced to increase the detection of both short variants (SNPs and insertions and deletions (INDELs)) and structural variants (SVs).
The researchers compare their results to those from joint variant calling, the most advanced technique for genotyping a large number of samples, and they demonstrate that a graph-based approach can achieve the majority of the benefits of joint calling with substantially less computing effort.
Compensating for the Shortcomings of the Sequencing Technologies
Regardless of the ancestry of the NGS samples, the scientists have demonstrated in this study that Pan-Genome graphs can provide an overall accuracy improvement over linear references, and population-specific graphs that are supplemented with cohort-specific information offer the highest utility in reading alignment and variant calling.
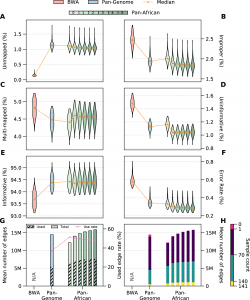
Image Source: https://doi.org/10.1038/s41467-022-31724-3
The nucleotide diversity within the population, the absolute divergence from the linear reference sequence, and the accessibility of pre-existing genomic data to be used for graph generation ultimately decide how well such graphs function. Although these two measurements are quantitatively comparable, they are fundamentally distinct.
The number of samples required to build a representative graph is determined by nucleotide diversity, which calculates the average genetic distance between any two members of the same population.
Populations with more diversity will need more samples to build a graph. The reference genome is solely utilized in the context of NGS secondary analysis to have an intermediate representation of an individual’s genome; hence, it does not theoretically affect the diversity measurement.
However, absolute divergence, which measures the genetic separation from a randomly chosen DNA sequence (such as GRCh38), can range from low to high for populations with comparable levels of diversity, was empirically demonstrated.
For instance, of the five super-populations, the East-Asian super-population has the greatest divergence from GRCh38 and the lowest level of diversity.
Absolute divergence can have a major impact on how well bioinformatics tools function. When applied to divergent populations, traditional procedures can lose accuracy, which is typically represented in increased alignment reference bias and lower variant calling sensitivity.
To make up for the constraints of the currently employed sequencing methods in cases of significant divergence, it may be advantageous to also incorporate outcomes from orthogonal technologies (for example, long-read sequencing data).
Capturing the Genetic Architecture
The strategy for sample selection in the variant curation method for graph creation given in this work is based on the genetic diversity of the population.
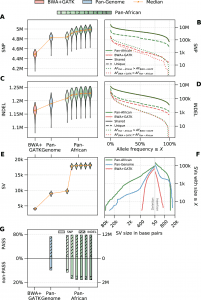
Image Source: https://doi.org/10.1038/s41467-022-31724-3
The needed number of samples and the representativeness of the resulting genome graph are also estimated, along with related TPR and FPR.
While keeping in mind that the size and sampling of the population ultimately influence how well the genome graph converges to the desired level of representativeness, the TPR and FPR table can be used for the assessment of the number of samples required for graph creation.
The scientists anticipate that for varied populations, the benefits of a genome graph will be more pronounced.
This is particularly crucial because it has long been established that the precise and sensitive detection of variations in under-represented populations is crucial to the precision of popular population genomics techniques like GWAS.
The African superpopulation, which is the most diverse and divergent of the five populations in the 1000 Genome dataset, was used to evaluate the graph creation method.
The iterative graph creation approach highlights the significance of obtaining genetic data directly from the cohort under research and making the graph reference more individualized to the cohort while exemplifying the appropriateness of genome graphs for large-scale sequencing efforts.
Secondary analysis is demonstrated to be improved on numerous fronts by moving from the least specific linear human genome reference, GRCh38, to a pan-genome and then to a collection of population-specific graphs.
As graphs are further tuned to sub-populations or even smaller groups of people with increased genetic similarity, the scientists anticipate seeing this improving trend continue—as long as there are still enough samples in the group to capture the genetic architecture adequately.
The Endpoint
Scientists have demonstrated that the detection of additional variations and the genotyping of SVs at the population scale are made easier by population-specific genome graphs.
The typical BWA+GATK pipeline entirely misses thousands of functionally significant mutations in the coding areas, which they were able to find.
Additionally, compared to dbSNP154, the researchers have found considerably more previously unknown SNPs and INDELs.
To fully comprehend the implications of these mutations for research and therapeutic applications, more research is necessary.
The researchers have also demonstrated that the variants that are genotyped using the Pan-African graph tend to have higher confidence than those that are not genotyped, demonstrating yet another benefit of population-specific graphs: they can easily provide the sensitivity and specificity improvements expected from joint calling without requiring the simultaneous processing of all samples in the cohort.
When used with large cohorts, this capacity of a graph-based approach eliminates the computational overhead associated with joint calling.
Article Source: Tetikol, H.S., Turgut, D., Narci, K. et al. Pan-African genome demonstrates how population-specific genome graphs improve high-throughput sequencing data analysis. Nat Commun 13, 4384 (2022). https://doi.org/10.1038/s41467-022-31724-3
Learn More:
Top Bioinformatics Books ↗
Learn more to get deeper insights into the field of bioinformatics.
Top Free Online Bioinformatics Courses ↗
Freely available courses to learn each and every aspect of bioinformatics.
Latest Bioinformatics Breakthroughs ↗
Stay updated with the latest discoveries in the field of bioinformatics.
Tanveen Kaur is a consulting intern at CBIRT, currently, she's pursuing post-graduation in Biotechnology from Shoolini University, Himachal Pradesh. Her interests primarily lay in researching the new advancements in the world of biotechnology and bioinformatics, having a dream of being one of the best researchers.