Scientists from the University of Michigan have developed a single-cell guided pipeline to aid the repurposing of drugs (ASGARD) by defining a drug score for drug recommendation. The drug score is calculated by considering intercellular heterogeneity in each patient tissue sample. Single-cell RNA sequencing (scRNA-seq) is essential for addressing and analyzing intercellular heterogeneity in several diseases. Existing drug repurposing methods that have been developed by considering the plethora of information that single-cell data provides are few. As compared to these methods, ASGARD has been shown to have higher accuracy and is found to be more robust. The authors have tested the pipeline on a breast cancer dataset and the COVID-19 patient datasets and predicted potential therapies. ASGARD promises to be a suitable tool for drug repurposing in personalized medicine.
Why do we need a single-cell guided pipeline?
Intercellular heterogeneity in disease tissue is the major cause of treatment failure in several diseases, such as cancer, COVID-19, Alzheimer’s disease, and stroke. Precision medicine is also challenged by the diverse cellular populations present within the diseased tissue. With the advent of single-cell RNA sequencing technologies and their advancements, the analysis of intercellular heterogeneity is possible at a very fine scale, thereby advancing our understanding of several disease mechanisms. The strategy of drug repurposing involves the identification of new drug uses outside the scope of its original approval. Previous bioinformatic pipelines developed for drug repurposing predict drugs for each cell cluster for the patient. These include the pipelines developed by both Alakwaa and Guo et al. developed during the COVID-19 pandemic to predict drug repurposing. However, in diseases caused due to intercellular heterogeneity, drugs need to address multiple cell clusters, which is not the case for these two pipelines. Thus, a pipeline is needed to address multiple cell clusters in drug repurposing. The authors developed A Single-cell Guided platform to Aid the Repurposing of Drugs, ASGARD.
Workflow of the ASGARD pipeline
- Normal and diseased cells are paired according to anchor genes that are expressed consistently between the two cell types.
- The differentially expressed (DE) genes are identified between normal and diseased cells, either in a cluster or within the same cell type.
- Potential drugs that significantly reverse the pattern of DE genes are identified using the consistent DE genes as input.
- As a final step, the pipeline estimates and ranks the drug scores for single drugs by targeting specific single-cell clusters or all cell clusters.
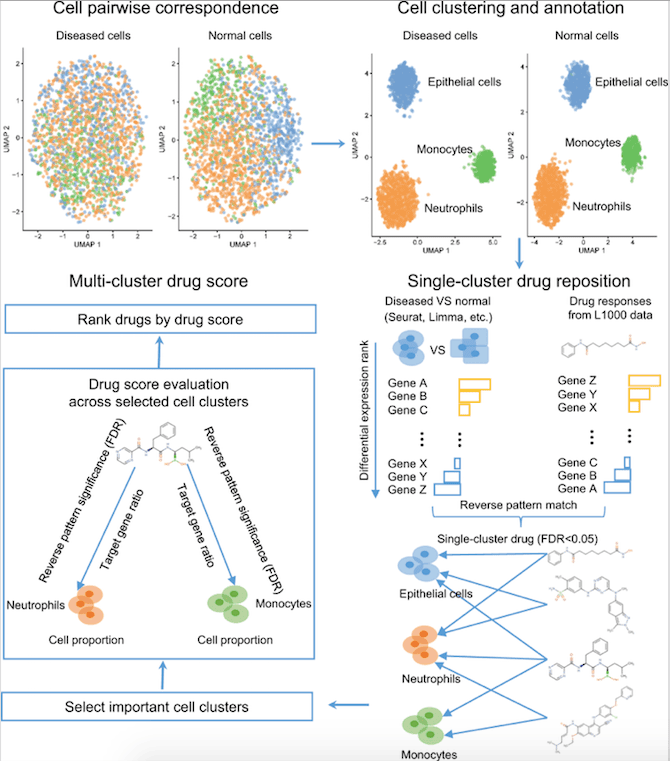
Image source: https://doi.org/10.1038/s41467-023-36637-3
The validated and accurate pipeline for drug repurposing: ASGARD
The authors validated AGARD using TRANSACT, the drug response prediction method. The ASGARD and TRANSACT pipelines, when applied to the TNBC (Triple-Negative-Brest-Cancer) dataset, yielded well-correlated results. The benchmarking results reveal that ASGARD performs more accurately on single drugs than other pipelines using bulk and single-cell RNA-seq data. ASGARD was found to have robust performance across different sizes of single-cell populations. The performance of ASGARD is also robust across different expression levels of cell populations.
Drug repurposing for diseases
ASGARD was applied to three datasets depicting three disease scenarios, viz., cancer, COVID-19, and leukemia. In each of these cases, the authors applied the pipeline to single-cell RNA-seq data from patients for drug repurposing.
The authors collected single-cell RNA-seq data from more than 24,000 epithelial cells of advanced metastatic breast cancer patients and around 17000 epithelial cells from normal breast tissue. Multi-cluster drug repurposing by ASGARD resulted in the prediction of 11 drugs.
The authors next applied the pipeline to address drug repurposing for COVID-19. They compared the single-cell RNA-seq data from deceased severe and cured severe COVID-19 patients. They identified the differential gene expression of four types of cells associated with COVID-19 disease and applied the pipeline to identify candidate drugs using the multi-cluster drug score. Rescinnamine and enalapril were among the identified drugs that are known to be angiotensin-converting enzyme (ACE) inhibitors. It is well established now that ACE2 mediates SARS-CoV-2 cell entry. This is indeed significant, given the massive impact of the pandemic on human health and life.
The authors also tested ASGARD on a leukemia dataset consisting of single-cell RNA-seq data from two precursor T-cell acute lymphoblastic leukemia and three normal healthy controls. The drugs and genes predicted by ASGARD have significance in the pre-cursor T-cell leukemia pathogenesis, as known previously.
Conclusion
The authors present ASGARD as a new generation of personalized drug recommendation systems. While previous pipelines, both based on bulk as well as single-cell RNA-seq data developed for drug repurposing predicted drugs for single-cell clusters efficiently, failed to do so for multi-cell clusters. Intercellular heterogeneity is the driving cause of treatment failure in several life-threatening diseases, it was the need of the hour to have a drug-repurposing pipeline addressing multi-cell clusters. The ASGARD pipeline efficiently and accurately predicts drugs for repurposing using single-cell RNA sequencing data as the guideline. The robustness of the method in terms of different cell population sizes and proportions, as well as different cellular expression levels, has been shown. The method has also been benchmarked and validated using standard methods for drug repurposing. The identification of drugs for the three disease cases, viz, cancer, leukemia, and COVID-19, emphasizes the fact that the pipeline is a breakthrough development in bioinformatics as well as a game changer in personalized medicine and therapeutics.
Article Source: Reference Paper | ASGARD is available as an R package on GitHub
Learn More:
Banhita is a consulting scientific writing intern at CBIRT. She's a mathematician turned bioinformatician. She has gained valuable experience in this field of bioinformatics while working at esteemed institutions like KTH, Sweden, and NCBS, Bangalore. Banhita holds a Master's degree in Mathematics from the prestigious IIT Madras, as well as the University of Western Ontario in Canada. She's is deeply passionate about scientific writing, making her an invaluable asset to any research team.