Predicting protein structure has been revolutionized by machine learning over the past two years. A similar breakthrough in protein design has now been reported in the journal Science. The researchers from the Baker lab at the University of Washington demonstrate that machine learning makes the design of proteins much more accurate and faster with a robust deep learning-based protein sequence prediction method called ProteinMPNN. This could lead to the discovery of many new vaccines, treatments, carbon-capture tools, and sustainable biomaterials.
Though deep learning has revolutionized protein structure prediction, Rosetta, a physically-based approach, has been used to generate almost all experimentally characterized de novo protein designs. A deep learning-based method for designing proteins, ProteinMPNN, is presented, which delivers outstanding results both in silico and in experiments.
What is ProteinMPNN?
ProteinMPNN is a deep learning algorithm-based protein sequence design method. It employs a neural network to analyze large sets of protein data and identify patterns that can be used to create new proteins with specific properties.
ProteinMPNN is to protein design what AlphaFold was to protein structure prediction.
David Baker, Biochemistry Professor at School of Medicine, University of Washington
Protein design challenges can be addressed by coupling amino acid sequences at different positions between single or multiple chains. ProteinMPNN is able to recover 52.4% of protein sequences on native protein backbones, while Rosetta is able to recover 32.9% of sequences. By incorporating noise during training, it is possible to improve sequence recovery on protein structure models, as well as produce sequences that encode structures more robustly when compared to those predicted by structure prediction algorithms. A wide range of protein monomers, cyclic homo-oligomers, tetrahedral nanoparticles, and target binding proteins can be rescued using X-ray crystallography, cryoEM, and functional studies using ProteinMPNN, which is highly accurate and useful.
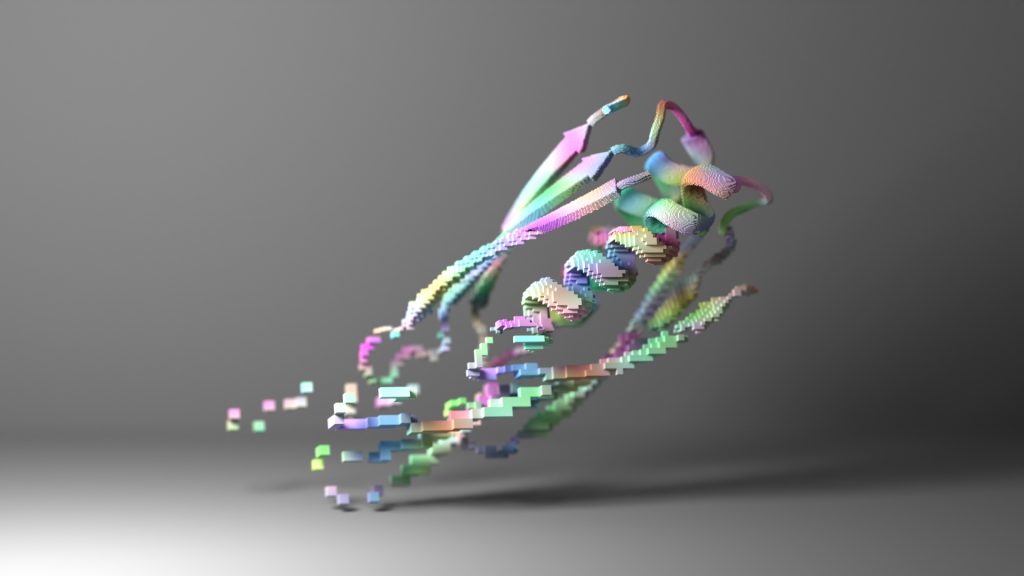
Image Source: https://doi.org/10.1101/2022.06.03.494563
An amino acid sequence must be designed to fold into the protein backbone structure, given a protein backbone structure of interest. The Rosetta algorithm, for instance, optimizes amino acid identities and conformations to find the combination of amino acid identities and conformations with the lowest energy for a given input structure. It has been found that deep learning approaches are promising for rapidly generating plausible amino acid sequences given monomeric protein backbones without requiring explicitly taking into account sidechain rotamerization. Using deep learning approaches, it is possible to rapidly generate plausible amino acid sequences given monomeric protein backbones without the need to perform intensive computational analysis. There is, however, an insufficient amount of challenges in designing a protein.
ProteinMPNN: The Future of Protein Design
A protein MPNN solves sequence design problems in a fraction of the time needed by physically based approaches such as Rosetta (1.2 seconds on a single CPU) for a 100-residue protein. Unlike Rosetta, which performs large-scale sidechain packing calculations, ProteinMPNN achieves much higher protein sequence recovery on native backbones (52.4% versus 32.9%) and, most importantly, rescues previously failed protein monomers, assemblies, and protein-protein interfaces that were previously failed using Rosetta or AlphaFold. ProteinMPNN is an extension of the previously described message-passing neural network (MPNN) method, which uses machine learning to design sequences. However, these approaches have mostly focused on monomer design and achieved lower native sequence recoveries. With the exception of a TIM barrel design study, no extensive crystallography or cryoEM verification has been carried out to evaluate the design accuracy.
The structure prediction method can be evaluated purely in silico, whereas protein design methods can’t be evaluated in silico: In silico metrics such as native sequence and recovery are extremely sensitive to crystallographic resolution and may not correlate with proper folding (even a single substitution of a single residue sequence design methods’ ultimate test is experimental characterization, which can cause no change in sequence recovery, but may prevent folding).
Unlike Rosetta and other physically based methods, ProteinMPNN does not require expert customization, making protein design more accessible to a wider audience. Sequence design problems are fundamentally framed differently, resulting in this robustness. An amino acid sequence will be identified based on its lowest energy state according to a traditional physical approach to sequence design.
Due to the need to compute energies over all possible structures, including unwanted oligomeric and aggregated states, this is computationally intractable; instead, Rosetta and other approaches use structure prediction calculations as a proxy to find the lowest energy sequence for a given backbone structure. A second step is required to make sure there are no other structures where the sequence has lower energy than the first. To generate sequences that fold, considerable customization may be necessary because the actual design objective is not in line with what is being explicitly optimized; Rosetta design calculations, for example, often restrict hydrophobic amino acids on the protein surface so that they do not stabilize undesired multimeric states, and it is unclear to what extent these restrictions should be applied at the boundary between the protein surface and core.
Despite lacking the physical transparency of methods like Rosetta, deep learning methods are trained directly to determine which amino acid is most likely to make up a protein backbone, given all the examples in the PDB, which eliminates ambiguities and makes sequence design more robust and less dependent on human judgment.
With its high experimental design success rate, high compute efficiency, applicability to almost any protein sequence design problem, and lack of customization requirements, ProteinMPNN has become the standard method for protein sequence design at the Institute for Protein Design, and it is expected to be quickly adopted throughout the research community.
ProteinMPNN designs exhibit a high propensity to crystallize as well, which greatly facilitates finding the structure of designed proteins. Using single sequence information in both cases, ProteinMPNN generated sequences are predicted more confidently and accurately to fold to native protein backbones.
Conclusion
ProteinMPNN could be widely useful in improving the expression and stability of native proteins expressed recombinantly (residues necessary for the function would obviously need to remain unchanged). ProteinMPNN is currently being extended to design protein-nucleic acids and protein-small molecules, which will greatly increase its utility.
ProteinMPNN code is available on GitHub.
Learn More:
Top Bioinformatics Books โ
Learn more to get deeper insights into the field of bioinformatics.
Top Free Online Bioinformatics Courses โ
Freely available courses to learn each and every aspect of bioinformatics.
Latest Bioinformatics Breakthroughs โ
Stay updated with the latest discoveries in the field of bioinformatics.
Srishti Sharma is a consulting Scientific Content Writing Intern at CBIRT. She's currently pursuing M. Tech in Biotechnology from Jaypee Institute of Information Technology. Aspiring researcher, passionate and curious about exploring new scientific methods and scientific writing.
[…] at crystallization also proved to be unsuccessful. A second set of proteins was designed using ProteinMPNN, with their sequences modified, and these were filtered with the help of AlphaFold2 (which was […]