Researchers at AstraZeneca and Ono Pharmaceuticals sought to create a model that could predict the likelihood that individuals with Type 2 Diabetes Mellitus (T2DM) who had no prior history of CKD or CVDs would develop CKD/HF. Using the prediction model, it would be possible to identify individuals with T2DM who are at a high risk of developing CKD/HF early on. Ultimately, the prognosis of these patients might be improved by tailoring therapeutic interventions based on the risk assessment.
T2DM, a recognized risk factor for heart failure (HF) and cardiovascular illnesses such as chronic kidney disease (CKD), was diagnosed in an estimated 537 million people worldwide.
In order to manage CKD and HF in individuals with early stages of T2DM, a complete treatment strategy is preferred. Early and better detection of these disorders can improve results. But recent research indicates that T2DM patients’ CKD and HF are not properly recognized, which results in increased rates of disease progression and a worse prognosis.
Over the last several years, numerous risk assessment prediction models, including diabetes severity, complications, hospitalizations, disease progression, and unfavorable outcomes, have been developed utilizing machine learning (ML) approaches. There are presently no ML algorithms for the prediction of progressing CKD/HF among patients in the initial stages of T2DM before CKD/HF emerges, despite the fact that ML algorithms can evaluate complicated relationships utilizing comprehensive data to enhance discrimination, learning patterns and decision rules.
Prevalence of comorbidities in Japan
Based on the study of 10,151 Japanese patients having T2DM between 2017 and 2019, the Japan Diabetes Comprehensive Database Project premised on an Advanced electronic Medical record System (J-DREAMS) study revealing that 35.4% had CKD and 22.1% had CVD, with the incidence rising with age and disease duration.
By examining a database of 1,177,896 individuals from six different countries, including Japan, Birkeland et al. demonstrated that CKD/HF is the most prevalent first symptom in patients with the early stages of T2DM.
Outcomes for risk predictions
The following clinical indicators were developed into risk prediction models:
The primary outcomes were
(1) Assessment of CKD/HF in an inpatient or outpatient environment.
(2) Hospitalization for CKD/HF or unclear causes, such as the greatest healthcare resource utilization during admission due to CKD/HF.
Secondary outcomes were
(1) an HF diagnosis (inpatient or outpatient);
(2) a CKD diagnosis (inpatient or outpatient); and
(3) hospitalization for HF or other causes for which the use of healthcare resources was at its highest during the admission.
Finally, exploratory outcomes were
(1) Myocardial infarction (MI) diagnosis, stroke diagnosis, or in-hospital death associated with MI or stroke;
(2) composite major adverse renal and cardiovascular events (MARCE), MI diagnosis, stroke diagnosis, or hospitalization associated with HF; renal outcomes (dialysis and kidney transplant); or in-hospital death associated with MI, stroke diagnosis, or HF; and
(3) all in-hospital deaths.
Model design and analysis
The model’s layout was designed in two distinct phases. In all rounds, 80% of the total analytic dataset was utilized for internal validation, and the remaining 20% was used to build the model.
First phase: a crude model
A feasibility analysis of algorithm development and a variable evaluation was part of the first step. Explanatory variables were entered, lab data was handled, and missing data were handled as part of the preparation of the data. The area under the receiver operating characteristic curve (AUROC), precision, accuracy, and recall were used to assess the model’s performance after the basic model had been constructed using random forest and logistic regression techniques.
Second phase: a complete forecasting model
The comprehensive prediction model was developed and adjusted in the second phase in order to complete and verify the model.
In Phase II, two distinct modeling approaches—gradient boosting [XGB] and deep learning [multilayer perceptron]—were compared using more conventional statistical models—logistic regression and Cox proportional hazards.
In this research, the precision, recall, and specificity for each outcome were used to assess the model’s accuracy. In addition, patients were split into high- and low-risk groups for Kaplan-Meier analysis based on the best cutoff value identified by the receiver operating characteristic (ROC) curve, which was determined as the point on the ROC curve that provides the shortest distance between the arc of the ROC curve and upper-left corner of the unit square (sensitivity = 1, specificity = 0). The ideal threshold (cutoff point) for separating the two groups in a survival analysis is at this point.
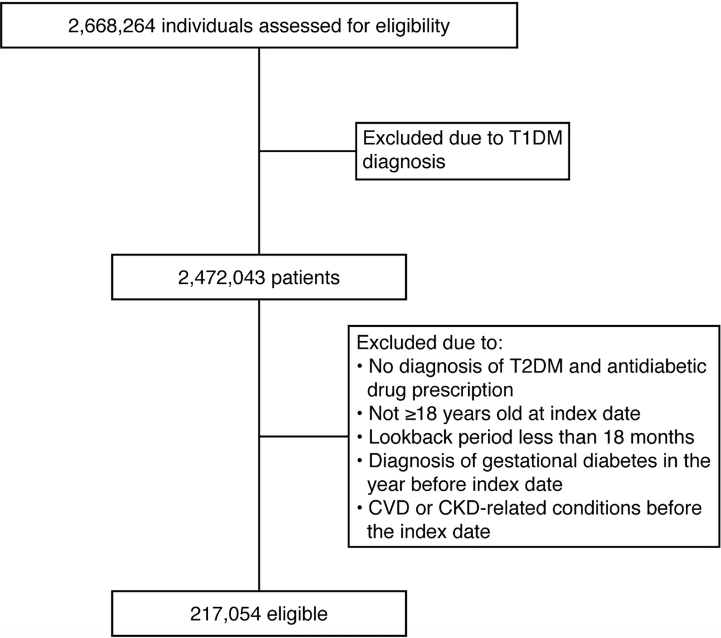
Image source: https://doi.org/10.1038/s41598-022-24562-2
External validation is a vital stage in determining a prediction model’s quality and clinical usefulness. AUROCs remained this model’s external validation. The model’s performance was assessed by contrasting the high-risk and low-risk groups found using the optimal cutoff values. The high-risk group showed a greater prevalence of all outcomes over the 5-year follow-up. These validation findings show that this model might be a helpful tool for numerous doctors in the treatment of T2DM patients.
One possible reason for the lack of early treatments and preventative measures for CKD and HF in individuals with T2DM might be the difficulty in identifying people at a high risk of these problems. It is generally known that HF exhibits phenotypic and pathophysiological variety and that this heterogeneity does not always manifest itself in HF-specific clinical manifestations. On the other hand, CKD can be detected using urine results or estimates of glomerular filtration rates. However, individuals with early-stage CKD seldom exhibit any symptoms or indicators, which delays the testing of renal function. Regardless of the length of the disease or the severity of T2DM, this model may help doctors become more aware of the value of surveillance for CKD and HF.
The analytical performance may be enhanced by applying the method utilized in the current work to other illness fields, such as infectious disease epidemiology. Experimental disease models have been extensively used to characterize, comprehend, and forecast the transmission of infectious diseases at various geographical scales, from the individual to the regional to the global. One such instance is COVID-19, for which recent investigations have shown crucial elements such as the vaccination rate for social spreading and the ventilation conditions for interior spreading.
Shortcomings
- First, the database for the study only contained data from DPC-covered institutions, a program for hospitals providing secondary care.
- Second, because this study employed a secondary database, it’s possible that explanatory factors and results need to be correctly categorized.
- Third, the databases utilized in this study only contain in-hospital fatalities, and the mortality rate for other types of hospitals, including clinics, may differ.
- Fourth, because the database only contains data from a select group of institutions, some statistics may have been overstated.
- Last but not least, a large proportion of patients in the MDV database have missing laboratory values.
Concluding views
In T2DM patients without a history of these illnesses, our ML model may predict the scientific data of acquiring CKD or HF as well as other clinical outcomes that are related to these disorders. Additionally, it might detect high-risk groups among individuals with poor prognoses. By promoting efficient communication between doctors, medical personnel, patients, and their families, visualizing patient risk may help multidisciplinary intervention and shared decision-making. In addition, the SHAP analysis revealed risk variables for undesirable outcomes that should be taken into account in clinical practice.
The generated model accurately predicted the likelihood of developing CKD/HF in T2DM patients in the external validation cohort. Clinical approaches may improve prognosis by encouraging early diagnosis and employing ML models to detect T2DM in patients at high risk of developing CKD/HF.
Article Source: Reference paper
Learn More:
Top Bioinformatics Books ↗
Learn more to get deeper insights into the field of bioinformatics.
Top Free Online Bioinformatics Courses ↗
Freely available courses to learn each and every aspect of bioinformatics.
Latest Bioinformatics Breakthroughs ↗
Stay updated with the latest discoveries in the field of bioinformatics.
Riya Vishwakarma is a consulting content writing intern at CBIRT. Currently, she's pursuing a Master's in Biotechnology from Govt. VYT PG Autonomous College, Chhattisgarh. With a steep inclination towards research, she is techno-savvy with a sound interest in content writing and digital handling. She has dedicated three years as a writer and gained experience in literary writing as well as counting many such years ahead.