There has been an increase in research in numerous areas of biology and medicine due to the development of mass spectrometry lipidomics. As a result, massive amounts of raw data have been produced, which calls for cutting-edge techniques to handle automated data processing. Several software tools that meet particular needs have been developed to address this. However, since they frequently have to test several options, which can be time-consuming and ineffective, scientists may need help deciding which tool is best for their research.
The review published in Nature Methods aims to help users navigate the vast landscape of software and tools available for lipidomics by highlighting the most widely used options and providing a convenient, interactive guide through the Lipidomics Tools Guide on LIPID MAPS.
What are lipids?
Lipids are water-insoluble molecules with different functions involved in different cellular processes as well as in tissue and organ physiology. Lipid functions range from subcellular partitioning to cell signaling. They are also involved in the maintenance of electrochemical gradients, energy storage, protein trafficking, and membrane anchoring. Owing to the varied functions of lipids, the physiological implications of lipid abnormalities manifest in various diseases, viz., cancer, diabetes, hypertension, Alzheimer’s disease, dermatological diseases, and many more, leading to the rising interest in understanding lipids.
Lipidomics, a sub-discipline of metabolomics, deals with the detailed analysis of lipids at a large scale using analytical chemistry principles and computational and technological tools. The recent development of effective analytical methods for identifying and characterizing lipids has also aided the biomedical community, as lipids act as biomarkers for disease pathophysiology.
Lipidomics involves the study of cellular lipid networks and pathways involving them. Lipidome implies the complete lipid profiling of a cell, tissue, organ, or organism. Lipidomics involves the identification and annotation of lipids, the interpretation of the pathways involved, and the understanding of complex interactions.
Lipids are identified using Mass Spectroscopy (MS) techniques. The ‘omics’ in lipidomics involve advanced bioinformatic analysis using computational tools. Over the last decade, advancement in MS technology has led to the discovery of vast numbers of ‘omics-type’ data, and this number is increasing exponentially. This has led to the development of advanced computational tools and solutions.
Tools like LipidXplorer, LipidFinder, and Lipid Data Analyser (LDA) have enabled the characterization of diverse biological processes and sometimes novel discoveries. LipidFinder aided in generating the first envelope composition for SARS-CoV2. Other noteworthy tools include Lipid Ontology, LION/Web, and XCMS. The plethora of available tools in lipidomics is ever-increasing and requires informed understanding for the user (scientist, biomedical practitioner) to be able to choose from this pool of tools.
Researchers are often faced with the daunting task of choosing among various approaches for data analysis. The choice of methods involves the underlying data structure and the research questions addressed. Understanding the algorithms aids in applying the most appropriate methods for a particular data set. This has mainly been done on an ad hoc basis resulting in a significant loss of time and effort.
LIPD MAPS has come up with a Lipidomics Tools guide and, as a luminary, will guide the user in identifying and testing appropriate computational tools as per their requirements. The guide comprises an interactive display guiding researchers in identifying appropriate pipelines based on the performance of tools. It also provides a detailed description of key features as illustrated in the following figure:
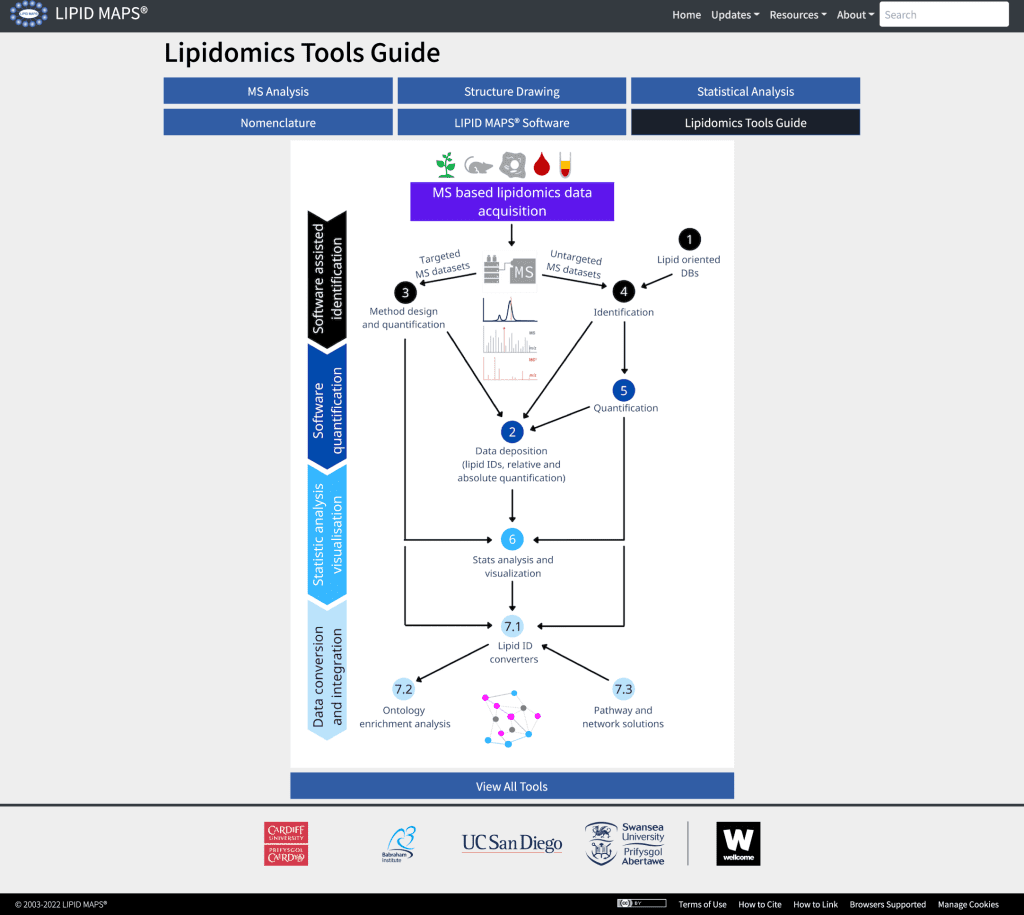
Image source:https://www.nature.com/articles/s41592-022-01710-0/figures/1
The website houses a GUI (Graphical User Interface) that illustrates several open-access solutions already available for lipidomics datasets. These are accessible via links to descriptions, video tutorials, and software developers’ contact details. The guide or tool spans the entire space of lipidomics data processing. The following figure details the seven major areas:
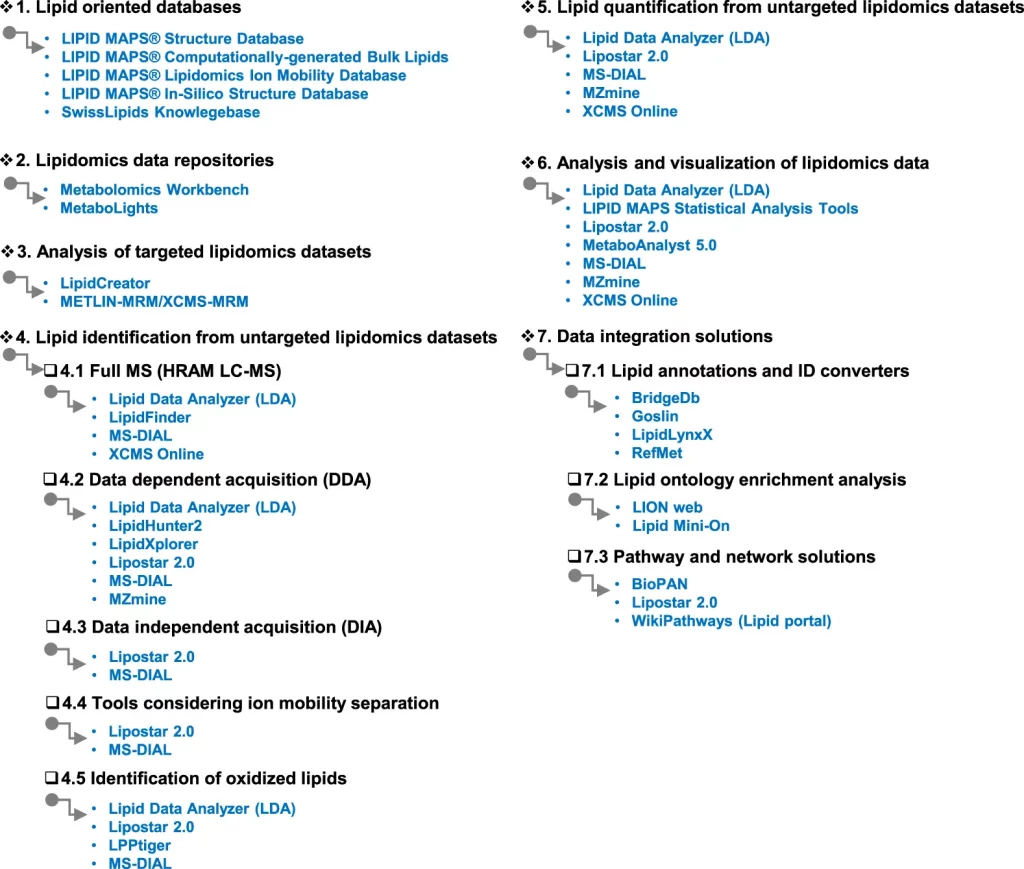
Image source: https://www.nature.com/articles/s41592-022-01710-0/figures/2
Categories of lipidomics tools
Lipid-oriented databases
The two most widely used lipid-specific databases are LIPID MAPS and SwissLipids. LIPID MAPS Structure Database (LMSD) contains over 47000 lipids obtained from both experimental and computational studies. The new addition to the existing toolkit is a display of reaction data linking lipid species by biochemical transformations. These were obtained from Rhea, WikiPathways, and Reactome databases.SwissLipids uses ChEBI ontology and Rhea for lipid metabolism.
MS data repositories
Metabolomics Workbench and MetaboLights are lipidomics-specific repositories for reporting raw and processed data.
Analysis of lipidomics datasets
There are two-generation strategies for Lipidomics data: Targeted and Untargeted. Targeted data acquisition is applied to a pre-defined set of lipids, whereas, in Untargeted data acquisition, the set of lipids is not known. LipidCreator and METLIN-MRM are target-based. Skyline and XCMS-MRM, although meant for peptides and metabolites, yet have been adapted for lipidomics as well.
Lipid identification
For the untargeted acquisition, Lipid Data Analyzer (LDA)47, LipidFinder48, MS-DIAL49, XCMS online50), DDA (LDA51,52, MS-DIAL, LipidHunter253, LipidXplorer54, Lipostar255, and MZmine56–58), DIA (MS-DIAL and Lipostar2) are available as applications.
Lipid quantification
Tools available for Lipid quantification are LDA, Lipostar2, MS-DIAL, MZmine, and XCMS online.
Statistical data analysis
Owing to the specific datasets generated by complex Lipidomics experiments, statistical data analysis often requires tailored approaches. The authors present clear guiding points for statistical analysis tests for statistically specific scenarios. From parametric and non-parametric hypothesis testing to PCA, these tests are widely used. Tools in this regard are LIPID MAPS Statistical Analysis Tools77 and MetaboAnalyst 5.0.
Data integration solutions
Available tools for pathway and network analysis in lipidomics are Lipostar2 and the stand-alone web application BioPAN. LION/Web and Lipid-Mii On are available lipid Ontologies.
Conclusion
The fast-growing field of Lipidomics has brought about several new software tools for processing high-throughput data. Open access resources in the form of tools for Lipidomics have been developed by academic researchers and are easily accessible. However, what remained elusive in terms of figuring out the right choice of tools has now been made easy by the authors. They present Lipidomics Tools Guide available on LIPID MAPS. Tutorials, video links, and relevant information are now available in one place. Tools ranging from lipid identification and quantification to visualization of networks and pathway analysis are now available under the same umbrella for novice users and research scientists.
Article Source: Reference Paper | The LIPID MAPS interactive Lipidomics Tools Guide
Learn More:
Top Bioinformatics Books ↗
Learn more to get deeper insights into the field of bioinformatics.
Top Free Online Bioinformatics Courses ↗
Freely available courses to learn each and every aspect of bioinformatics.
Latest Bioinformatics Breakthroughs ↗
Stay updated with the latest discoveries in the field of bioinformatics.
Banhita is a consulting scientific writing intern at CBIRT. She's a mathematician turned bioinformatician. She has gained valuable experience in this field of bioinformatics while working at esteemed institutions like KTH, Sweden, and NCBS, Bangalore. Banhita holds a Master's degree in Mathematics from the prestigious IIT Madras, as well as the University of Western Ontario in Canada. She's is deeply passionate about scientific writing, making her an invaluable asset to any research team.