A tumor is a complex tissue of cancerous cells associated with a heterogeneous cellular microenvironment. Using single-cell sequencing, tumor cells can be molecularly characterized. Identifying tumor cells within single-cell or spatial sequencing experiments is particularly challenging due to cell annotation, which assigns cell type or state to each sequenced cell. Ikarus is a machine learning pipeline to distinguish tumor cells from normal cells on the single-cell level. A variety of single-cell datasets show that Ikarus achieves high sensitivity and specificity.
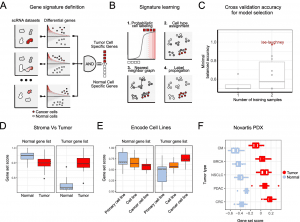
Image source: https://doi.org/10.1186/s13059-022-02683-1
As a disease that is caused by the disruption of the cellular state, cancer is a devastating disease. By undergoing genetic mutations, tumor cells become able to proliferate more rapidly than normal tissue. As a result of this process’ inherent variability, finding highly effective common therapies has been difficult, so precision medicine has become critical. By effectively identifying the complete heterogeneity within each tumor for each patient, single-cell experiments will revolutionize personalized medicine.
Tumor cells can be differentiated from surrounding tumor-associated tissue in an automated, parameterless manner. To gain direct clinical insights, a streamlined process for predicting neoepitopes can be applied to tumor cells. In addition, multi-omics measurements are increasingly available and could be used to characterize tumor subpopulations and suggest the best therapeutic measures for each subpopulation. Automated digital pathology is facilitated by the application of automatic tumor classification to spatial sequencing datasets. Quantitative characterization of the complete tumor heterogeneity for an individual is now possible with the current technological and computational advances in single-cell biology. Data analysis must, however, be completely automated, with robust performance guarantees, before personalized medicine can be readily adopted. The Ikarus machine learning pipeline can take a major step toward personalized cancer treatment.
Using single-cell technologies provides unprecedented precision in the characterization of biological systems, with all technical and biological influences clearly evident in the results. It is very difficult to enumerate and correct for all of the technical and biological variables causing the measured variability in cancer biology due to the heterogeneity of data composition. In addition to presenting new computational challenges, high-throughput single-cell sequencing also brought about new technical developments. Each sequenced cell must be assigned a cell type or a state as part of the single-cell data analysis process.
Cell dissociation is one example of an artifact that mimics MAP kinase pathway activation, but one cannot know exactly what the precise environment is inside a cell. Different oxygen gradients, physical constraints, or multiple signaling molecules may influence cells. Machine learning models must take into account this variability since data from different conditions will have different underlying distributions, limiting their ability to generalize from one dataset to another.
To mitigate distributional differences between single-cell RNA sequencing datasets, three types of methods have been developed: (1) Using manifold matching, multiple datasets are aligned into one space based on the similarities between their low dimensional representations; (2) Deep learning tools that adapt domains through latent space embeddings attempt to model batch effects (explicitly or implicitly); and classifiers that use learned marker genes and robust statistics to transfer knowledge between datasets based on gene sets.
A two-step approach to discriminating tumor cells from normal cells is perceived as a simple problem. A gene set that distinguishes tumor cells from normal cells is first extracted from multiple experts labeled datasets using the Ikarus pipeline. As a second step, Ikarus uses adaptive network propagation and robust gene set scoring to classify cells. Two common problems in single-cell analysis can be mitigated by robust gene set scoring and network propagation: batch effects on sample comparisons and parameter optimization during clustering.
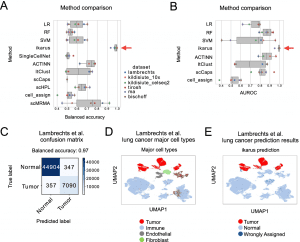
Image source: https://doi.org/10.1186/s13059-022-02683-1
When analyzing single cells, clusters of cells are aggregated, which are subsequently used to identify cell types. A clustering procedure, however, has an inherently high degree of parametric flexibility. When applying the same set of parameters to different datasets, it’s very difficult, if not impossible, to achieve the same level of accuracy (same cell type), necessitating manual intervention. The cell type and state form highly interconnected modules within the cell-cell similarity graph, so clustering has been replaced with network propagation. By integrating the annotation scores of each cell with those of its neighbors, network propagation reduces the uncertainty of cell annotation. As opposed to clustering, network propagation is parameterless and retains the same level of sensitivity for the annotation of individual cells.
In the process of Ikarus, two steps are involved: (1) the discovery of a comprehensive tumor cell signature as a gene set based on the consolidation of multiple annotated single-cell datasets from multiple experts; (2) the training of a robust logistic regression classifier that discriminates between tumor and normal cells stringently followed by network-based propagation of cell labels based on a custom cell-cell network. To test Ikarus sensitivity and specificity in several experimental contexts to determine whether it can be used as an in silico tumor cell sorter that is robust, sensitive, and reproducible, different sequencing technologies are used to acquire multiple single-cell datasets of various cancer types. The results of the testing process have been strictly monitored for contamination by information leakage from training.
Article Source: Dohmen, J., Baranovskii, A., Ronen, J., Uyar, B., Franke, V., & Akalin, A. Identifying tumor cells at the single-cell level using machine learning. Genome Biology, 23(1), 123. (2022). https://doi.org/10.1186/s13059-022-02683-1.
Learn More:
Top Bioinformatics Books ↗
Learn more to get deeper insights into the field of bioinformatics.
Top Free Online Bioinformatics Courses ↗
Freely available courses to learn each and every aspect of bioinformatics.
Latest Bioinformatics Breakthroughs ↗
Stay updated with the latest discoveries in the field of bioinformatics.
Srishti Sharma is a consulting Scientific Content Writing Intern at CBIRT. She's currently pursuing M. Tech in Biotechnology from Jaypee Institute of Information Technology. Aspiring researcher, passionate and curious about exploring new scientific methods and scientific writing.