Cancer is a complicated disease brought on by the interaction of various informational layers. Tumor origin, the appearance of genomic and transcriptomic variations, or interactions with the microenvironment are all factors that contribute to the difficulty of treating tumors. With reference to the types of tumor heterogeneity and the accessible data from next-generation sequencing, the current methods for selecting a therapy are presented in this review by the authors from the Spanish National Cancer Research Centre, Spain. The study elucidates the potential integration of bioinformatics in precision oncology.
Introduction
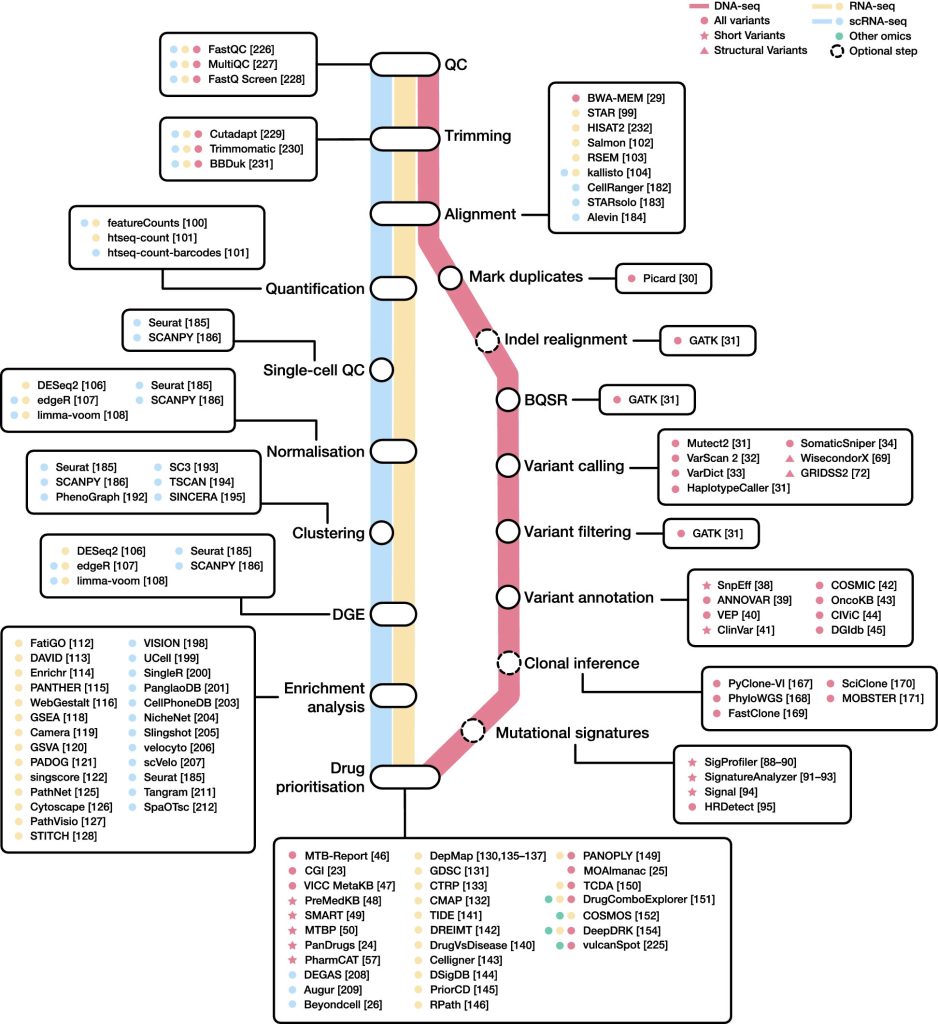
Image Source: https://doi.org/10.1002/1878-0261.13286
Cancer is an evolving dynamic disease that becomes more diverse as the disease progresses. One of the core reasons why medication doesn’t work and patients relapse has been identified as its heterogeneity. A burgeoning area called precision oncology aims to create personalized cancer treatments for every cancer patient using data from epidemiological, clinical, and omics sources. Targeted therapies are considered an anchorage of precision oncology. The Food and Drug Administration (FDA) has authorized 214 predictive biomarkers by the year 2022, up from 39 in 2013, as a result of ongoing attempts to identify novel predictive biomarkers of anticancer drug effectiveness. BRAF V600E inhibitors in melanoma patients and imatinib to target BCR-ABL translocations in chronic myeloid leukemia are common examples of targeted therapies.
Cancer is characterized by intra- and intertumor heterogeneity; both are included in the definition of tumor heterogeneity. The former refers to the occurrence of various genetic changes in cancer patients or within a single individual. The latter term refers to the inherent clonal diversity present in tumors as a result of somatic evolution and natural selection in cancer. This means that different tumors exhibit different molecular changes on various levels. Patients may react differently to the same therapy for all of these reasons. Therefore, it is critical to creating computational approaches for designing individualized anticancer therapy plans. The majority of the druggable genome has yet to be well examined, which makes developing targeted therapies difficult. A genomically guided therapy may not be effective for all individuals. New pharmacogenetic biomarkers have been discovered and developed as a result of next-generation sequencing (NGS) technologies.
Genomics Guided Drug Selection
With the goal of identifying clinically relevant genomic changes for cancer diagnosis and therapy recommendations, NGS has been widely used to study tumor DNA isolated from clinical and biological samples.
Data produced by targeted, whole-exome sequencing (WES) and whole-genome sequencing (WGS) investigations are put to use to computationally analyze, discover, and interpret DNA modifications, such as short variants and structural variations, which can help choose the best cancer therapy.
A variant calling analysis is used to find clinically meaningful variations in cancer patients as the first stage in the genomics-based therapy selection process.
SnpEff is a tool for automated variant annotation that assesses the biological effects of candidate variations. Other tools include ANNOVAR and the Variant Effect Predictor (VEP), which also offer data on each variant’s frequency.
Transcriptomics Guided Drug Selection
Transcriptomics profiling tries to prioritize treatment possibilities based on gene expression. The most popular NGS method for identifying and measuring RNA in biological samples is RNA-seq. Matching unprocessed sequencing reads to a reference genome or transcriptome, it creates a matrix of unnormalized gene counts. Some of the most popular tools for completing the aforementioned phase are the STAR aligner and aggregation programs like featureCounts or htseq-count. Following a DGE analysis, functional enrichment is frequently carried out with the goal of illuminating biological linkages in the list of differentially expressed genes and uncover underlying coordinated patterns.
Gene expression data and network-based algorithms can be used to prioritize drugs for cancer treatment. For instance, following the Celligner methodology, the transcriptomic profile of individual samples can be aligned to the most similar cancer cell line. PriorCD makes use of a network propagation algorithm and a drug-drug similarity network, along with pathway activity profiles, to prioritize drugs in cancer.
Drug Selection Using Integrated Multi-omics Strategies
To find and prioritize pharmacological targets, techniques like PANOPLY and MOAlmanac combine genomic and transcriptome data. A recently released database called The Cancer Druggable Gene Atlas (TCDA) contains details on genomic abnormalities such as short variants, CNVs, and gene fusions, as well as expression, gene dependence, and druggability.
Precision oncology is only beginning to incorporate bioinformatics approaches into immunotherapy. However, there are currently resources available that enable the creation of customized vaccinations. In order to create effective vaccinations based on patient-specific neoantigen profiles, it is possible to choose the prospective neoantigens with the best success rate from the extensive lists produced by NGS. Different computational techniques are included in neoantigen prediction pipelines like pVACtools to identify neoantigens in cancer DNA-seq and RNA-seq data. The presence of immunological infiltrates in tissue may also be inferred from expression data by programs like CIBERSORTX or MCP-counter.
Drug Selection Based on Tumor Heterogeneity
ITH functional diversity has been linked to somatic SNVs, SVs, transcriptome, and epigenetic modifications that affect gene expression levels, the presence of TMEs, and the antitumor immune response. ITH can be temporal if linked to clonal development and spatial if it occurs in several tumor areas. Based on the distinct mutational or transcriptome profiles of each subgroup of clonal subpopulations, we can presently assess the level of ITH and characterize them. Understanding ITH can be very beneficial for prioritizing medication therapies or determining how a tumor will respond to treatment.
Targeting Tumor Clonality via Genome Profiling
All tumor cells contain clonal mutations, while subclonal mutations affect only a subset. With SNV allele frequencies, CNV profiles, and tumor purity metrics as input, a number of bioinformatics algorithms have been created to estimate cancer subclones. PhyloWGS, PyClone-VI, FastClone, SciClone, or MOBSTER are some of the most notable instances. This strategy, nevertheless, has a few drawbacks. To start, only mutations that affect all or the majority of cells will be found. Furthermore, stromal contamination might change the frequency of mutations. Last but not least, these bioinformatics tools carry out numerous earlier inference processes that might create errors that propagate through later steps.
Drug Selection Based on Single-cell Transcriptomics
A new stride forward in our understanding of cancer biology has been made possible by the advent of single-cell RNA-seq (scRNA-seq) technology. Some of the most recent initiatives in this direction include the bollito pipeline, the Web-Accessible Single Cell RNA-Seq Processing Platform (WASP), and the Single Cell Interactive Application (SCiAp). Current single-cell analysis workflows can be broadly divided into three main steps:
- The steps for processing raw data, also known as the primary analysis.
- The steps for normalization and clustering, also known as secondary analysis.
- The steps for tertiary analysis involve the functional interpretation of the results.
One of the key shortcomings of scRNA-seq techniques is the absence of spatial context data. Tangram and SpaOTsc are two programs that map scRNA-seq data to spatial data gathered from the same area.
Implementing Drug Prioritization Tools in Clinical Practice
The aspect of drug prioritization presents numerous biological and technological impediments. Clinical decision support systems will incorporate computational approaches to analyze and interpret NGS data, including drug prioritization algorithms, relying on an extensive interchange of data, metadata, research tools, and computational infrastructure.
Data harmonization and standards are essential to connecting multimodal cancer data in a meaningful way to address this challenge.
Conclusion
In silico prioritization acts as a fundamental resource for precision medication. Computational methodologies are urgently needed to design personalized anticancer treatments. By using multi-omics profiles of individual tumors and clinical characteristics of each patient, precision oncology seeks to meet this challenge by proposing patient-specific therapies tailored to the multi-omics profiles of individual tumors and clinical characteristics of each patient.
In analogy to existing therapeutic approaches, which frequently combine a logical and medication-based synergistic therapy regime, these computational drug prioritization methods continue to rely on the one target-one drug-one disease idea. These approaches are still in their infancy. As a crucial part of designing precision medicine-based therapies, bioinformatics plays a crucial role in selecting tailored treatments that target tumor heterogeneity efficiently, as well as in incorporating these treatments into clinical practice.
Article Sources: Reference Paper
Learn More:
Top Bioinformatics Books ↗
Learn more to get deeper insights into the field of bioinformatics.
Top Free Online Bioinformatics Courses ↗
Freely available courses to learn each and every aspect of bioinformatics.
Latest Bioinformatics Breakthroughs ↗
Stay updated with the latest discoveries in the field of bioinformatics.
Riya Vishwakarma is a consulting content writing intern at CBIRT. Currently, she's pursuing a Master's in Biotechnology from Govt. VYT PG Autonomous College, Chhattisgarh. With a steep inclination towards research, she is techno-savvy with a sound interest in content writing and digital handling. She has dedicated three years as a writer and gained experience in literary writing as well as counting many such years ahead.