Occlusion myocardial infarction (OMI) impacts a large number of people, yet fewer get diagnosed. This is because their ECG fails to show an elevation in the ST region. An elevated ST region indicates the presence of OMI. Such patients can receive great help from immediate reperfusion therapy, but the problem is that no tool can accurately identify them in the initial assessment. At the University of Pittsburgh, USA, scientists conducted a study with a sample space of 7,313 patients in the US with the aim of developing machine-learning models for detecting OMI effectively through ECG. The developed models showed higher accuracy, precision, and sensitivity, outperforming clinicians and commercial interpretation systems. The features of the models were validated by clinical experts as well, increasing the scope for a better understanding of the underlying mechanisms of OMI.
Challenges in effective ECG diagnosis of Heart Attacks
Effective ECG diagnosis of acute coronary syndrome (ACS) in individuals with acute chest pain has long been difficult. Blockages in the coronary arteries cause ACS, a condition where there is less blood flow to the heart. To segregate individuals with ST-elevation myocardial infarction (STEMI) from those with other kinds of ACS, clinicians analyze ST-segment elevation (STE). In the absence of STE on the ECG, a biomarker-driven approach is followed. The biomarker-driven approach involves the measurement of cardiac biomarkers such as troponin. But this approach has two significant limitations. First, 25 to 35 percent of individuals with non-STEMI experience occlusion myocardial infarction (OMI), which entails total coronary artery blockage. Such patients are more vulnerable than patients with ACS with an open artery and require urgent insertion of a catheter into the blood vessels of their heart. They are also subjected to unnecessary treatment delays, which obviously increase their mortality risk. Even though ECG findings capable of indicating OMI are described in the literature, they are subtle and include complex changes in the ECG waveform. Thus, visual interpretation by clinical experts proves to be a rather suboptimal approach.
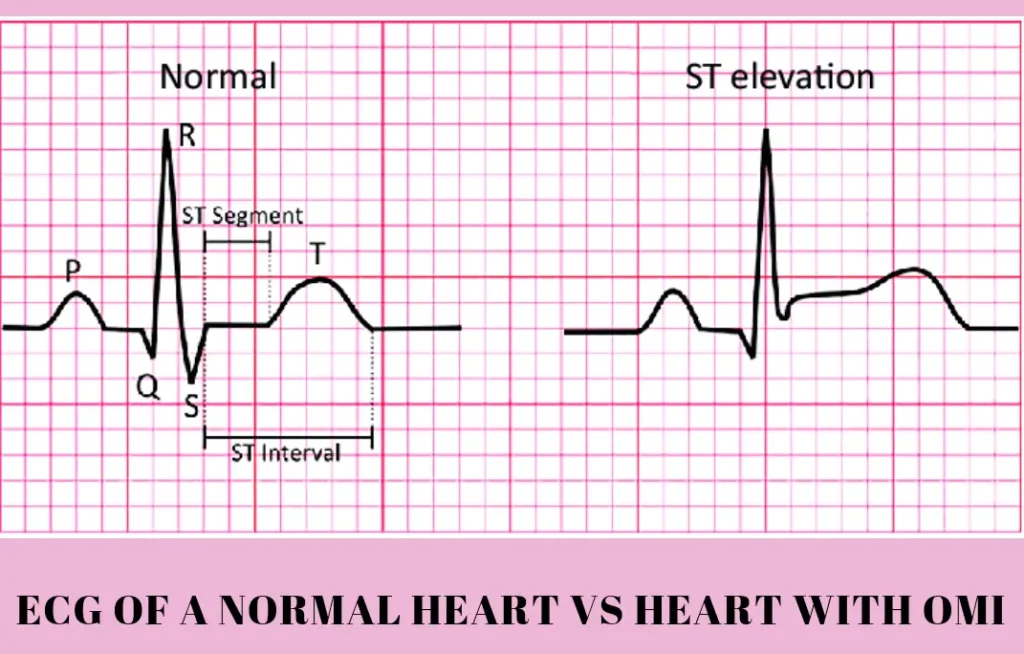
Image Source:https://www.techscience.com/CMES/v128n3/44018/html
The other limitation is that the troponin biomarker approach cannot differentiate OMI from other ACS until their levels reach a peak. Positive troponin results often come with a high false-positive rate, and about 33% of the patients have ambiguous results even after testing is done multiple times. To make matters more complicated, around 25% of acute myocardial infarction cases involving both STEMI and OMI initially show negative results. Such limitations lead to expensive and inefficient medical practices where most patients are over-monitored, and the remaining ones receive delayed treatment, increasing mortality risk by 14–22%.
So, is there an approach that can overcome these limitations and improve the accuracy of heart attack diagnoses? Maybe, coupling artificial intelligence with clinical practices can make a difference.
Empowering ECG Diagnosis with Machine Learning
The scientists conducted a cohort study to develop machine-learning models that can improve the diagnosis of OMI through ECG. The study involved three cohorts in total. One of them was a derivation cohort consisting of 4,026 patients with chest pain. The other two were validation cohorts, which together consisted of 3,287 patients. The validation and the derivation cohorts were similar with respect to age, gender, clinical characteristics, and 30-day cardiovascular mortality. However, in the validation cohort, a higher representation of black and Hispanic people was observed, along with a higher rate of acute coronary syndrome (ACS) and OMI.
At first, the models were tested on the derivation cohort. A total of 73 ECG features were chosen for training the models out of 554 metrics, and ten classifiers were trained to learn the patterns in ACS and non-ACS groups to estimate the probability of occurrence in OMI. A hybrid approach involving data-based methods and clinical expertise was employed. Out of all the models, the random forest (RF) model performed the best. The RF model succeeded in outperforming not only clinical experts but commercial interpretation systems as well. Patients were split into low-risk, moderate-risk, and high-risk categories with the help of probability density plots and OMI ratings (obtained with the use of the algorithms). The OMI score showed high sensitivity (to rule out OMI) and high specificity (to rule in OMI). The OMI score improved the sensitivity by 28% and the precision by 32%. The OMI score was also compared to the HEART score. The HEART score uses additional information such as age, risk factors, and troponin values. The OMI score fared much better than the HEART score in detecting OMI, and that too with ECG data alone.
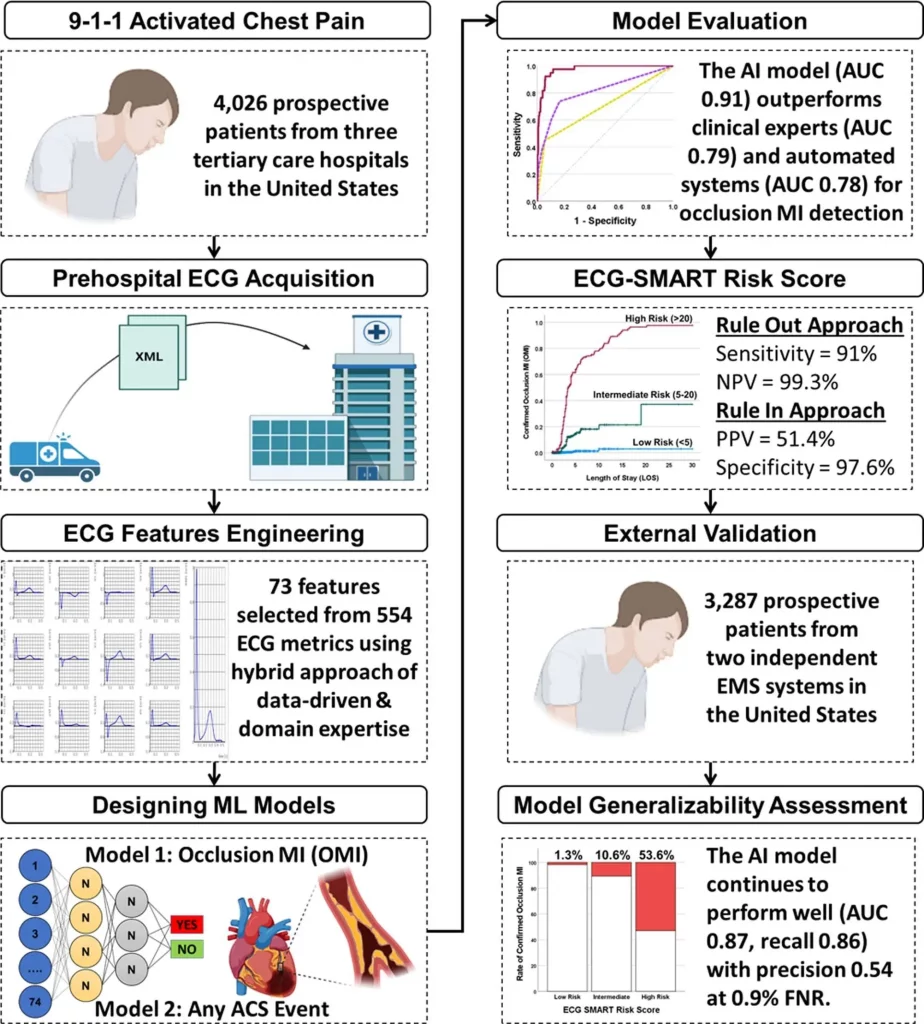
Image Source: https://doi.org/10.1038/s41591-023-02396-3
The final model was tested on 3,287 patients from two independent clinical sites. The OMI score succeeded in effectively predicting OMI risk. The accuracy of the model also remained consistent across various demographic and clinical characteristics. This indicates that the performance of the model was completely unbiased. Not only that but when coupled with the judgment of clinical experts, it was able to correctly reclassify one in three patients with chest pain. The model also demonstrated the ability to pinpoint certain ECG patterns for the detection of coronary occlusion that transcend clinical guidelines. It is sensitive enough to capture subtle ECG features that may be ignored by clinical experts.
Hence, the inclusion of machine learning-based methods in clinical practices indeed empowers clinical diagnosis as well as patients.
Current Scope of Machine Learning in the Medical World
The results shown by the machine-learning model are pretty satisfactory and point towards a hopeful future. Yet, currently, its scope has limitations. First, the model needs to be retrained for a variety of ECG software, as there are discrepancies between manufacturers. Discrepancies may also arise across different populations and regions, as the study only involved patients from the USA. The third limitation is that the ‘ruling in of ACS’ by the model outperformed the ‘ruling out.’ This is suggestive of certain events getting missed. Last but not least, its performance in the actual world and in real-time still lacks clarity.
Conclusion
Clinicians encounter certain challenges when it comes to accurately diagnosing patients with OMI via ECG alone. Even biomarker-based approaches come with their own set of shortcomings. To overcome these barriers that hinder the accurate diagnosis of OMI in cases where no ST-segment elevation appears in the ECG, researchers have tried incorporating machine learning into ECG diagnosis. So far, in their study, the developed machine-learning model has shown satisfactory and promising results. The model has outperformed clinical experts as well as commercial interpretation systems in terms of accuracy, precision, and sensitivity. The model has shown unbiased results, and when coupled with the expertise of clinicians, it even accurately reclassifies one in three patients with chest pain. Additionally, this study has helped researchers identify ECG patterns corresponding to coronary occlusion that go beyond the scope of clinical guidelines.
Even though the machine-learning approach seems invincible, it has its limitations too, especially its performance in real-time, which is yet to be ascertained. Nevertheless, the direction of progress appears to be optimistic and promising. Further research can certainly overcome the limitations and provide a better future for humanity.
Article Source: Reference Paper
Learn More:
Neegar is a consulting scientific content writing intern at CBIRT. She's a final-year student pursuing a B.Tech in Biotechnology at Odisha University of Technology and Research. Neegar's enthusiasm is sparked by the dynamic and interdisciplinary aspects of bioinformatics. She possesses a remarkable ability to elucidate intricate concepts using accessible language. Consequently, she aspires to amalgamate her proficiency in bioinformatics with her passion for writing, aiming to convey pioneering breakthroughs and innovations in the field of bioinformatics in a comprehensible manner to a wide audience.