Google’s DeepMind scientists have made a monumental stride with the inception of AlphaMissense, an AI tool that can pinpoint genes responsible for diseases. This move signals substantial progress in gene research and potentially transforms how rare genetic disorders are detected. The state-of-the-art capacities of AlphaMissense gear towards improving our grasp on intricate genetic changes, thus guiding us towards more exact diagnoses and tailored treatments. With this groundbreaking technology now unveiled, we’ve reached a crucial juncture in demystifying the enigma around genetic disorders.
What is a Missense Variant?
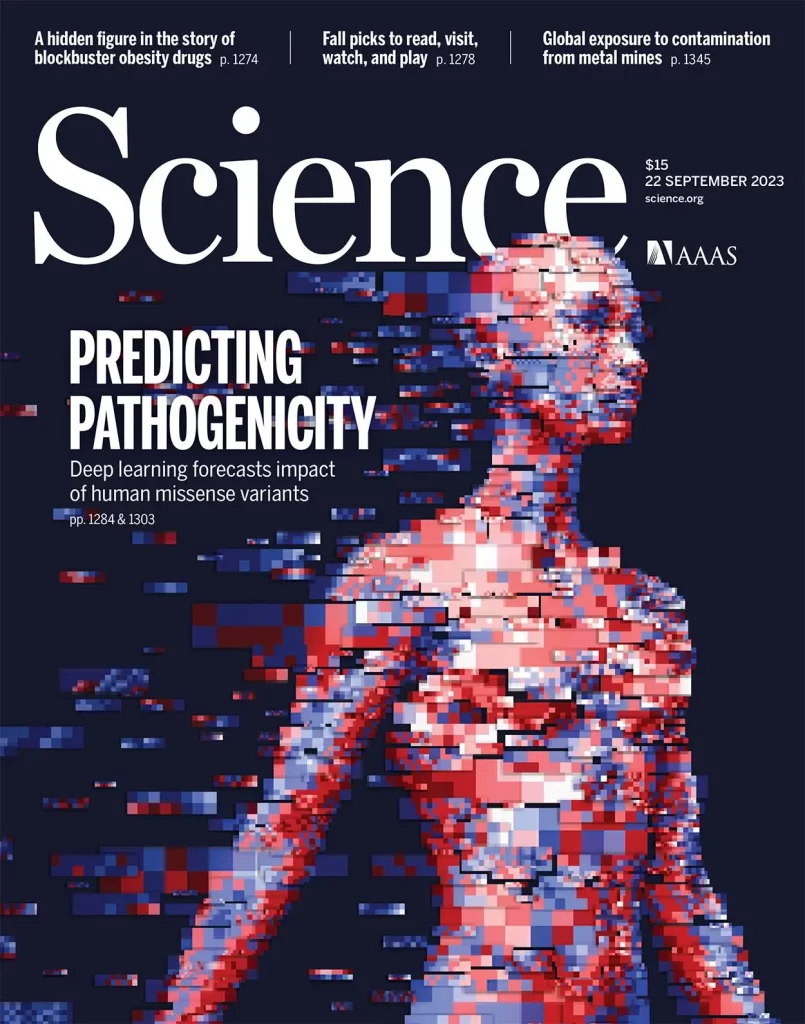
A missense variant is a single DNA letter alteration that causes the translation of a protein to contain a different amino acid. The function of the protein might be impacted, and illnesses can occur. Missense variations must be acknowledged and classified in order to understand their impact on human health and disease. The categorization of missense mutations is greatly improved by AlphaMissense, revealing their probable pathogenicity. Pathogenic missense variations can severely impair protein function, which has an effect on the organism’s overall fitness. Nevertheless, only a small portion of these missense variations have been categorically determined to be harmful or benign. A significant difficulty in human genetics continues to be the classification of the remaining variations with unclear relevance.
A predictive algorithm called AlphaMissense was created to evaluate the pathogenicity of missense variations. AlphaMissense, a cutting-edge protein structure prediction tool, is developed using human and primate variation population frequency databases. Even without direct training on such data, AlphaMissense achieves top-tier performance across a variety of genetic and experimental criteria by combining structural context and evolutionary conservation.
The pathogenicity score offered by AlphaMissense is useful for estimating a gene’s cell essentiality as well as the harmfulness of missense variations. The identification of short important genes, which are difficult to find using traditional statistical methods, is made possible by this prediction ability.
Current methodologies like multiplexed assays of variant effect (MAVEs) provide valuable insights into variant effects, but conducting proteome-wide surveys using MAVEs is impractical due to cost and labor constraints. Machine learning approaches offer promise for bridging this interpretation gap by predicting the pathogenicity of unannotated variants based on patterns in biological data.
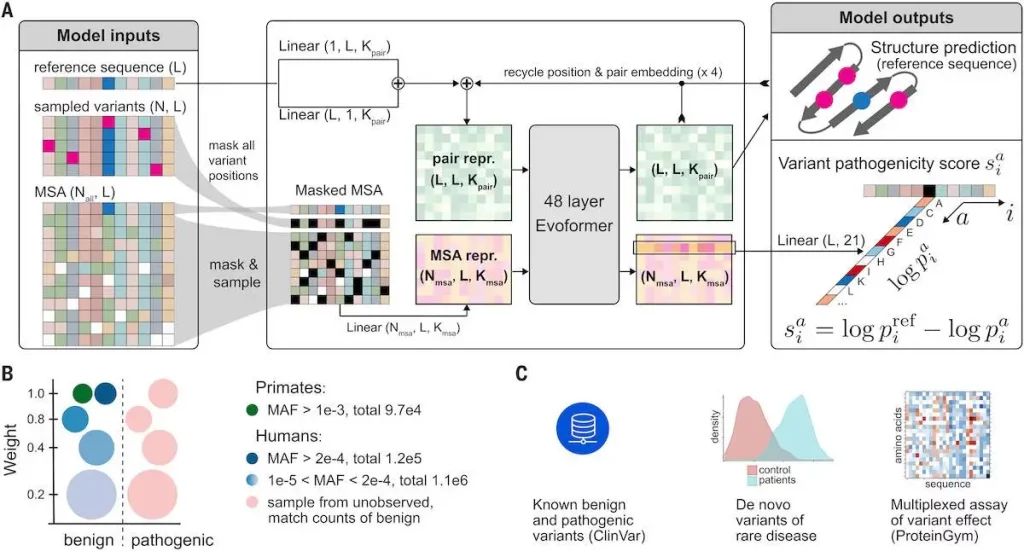
Image Source: https://doi.org/10.1126/science.adg7492
AlphaMissense: Fine-tuning AlphaFold for variant effect prediction
Existing machine learning strategies can be broadly categorized into four classes. The first class directly trains on human-curated variant databases, leveraging prior knowledge and potentially inheriting biases present in these databases. The second class employs weak labels based on population frequency data to mitigate human curation biases. The third class utilizes unsupervised approaches to model amino acid distributions, capturing high-order dependencies between protein sequences. The fourth class integrates protein structure information to reason about pathogenicity, utilizing accurate protein structure predictions like those from AlphaFold.
AlphaMissense incorporates elements from these strategies, including training on weak labels from population frequency data, unsupervised protein language modeling, and leveraging the accurate protein structure predictions from AlphaFold. The model predicts the pathogenicity of single amino acid changes in a protein sequence and achieves superior performance in predicting clinical annotation, de novo disease variants, and experimental MAVE benchmarks. By making predictions for all possible single amino acid substitutions in the human proteome and offering this as a resource to the community, AlphaMissense significantly contributes to advancing our understanding of variant pathogenicity.
Improved pathogenicity classification across multiple clinical benchmarks
AlphaMissense outperformed other models, achieving a high area under the receiver operator curve (auROC) of 0.940 on ClinVar missense variants, compared to the next-best model EVE with an auROC of 0.911. AlphaMissense even surpassed models trained directly on ClinVar, which suffer from data leakage and label circulatory issues. It demonstrated notable performance in distinguishing pathogenic from benign ClinVar variants within regions of high evolutionary constraint. The model consistently performed well across different AlphaFold confidence levels.
Furthermore, AlphaMissense was evaluated for clinically actionable genes and genes prioritized for future studies. It demonstrated improvements over EVE in both sets of proteins, showcasing its effectiveness in clinically relevant scenarios.
Calibrated AlphaMissense predictions expand the number of confidently classified variants relative to other methods
After establishing AlphaMissense’s superior performance, the researchers predicted pathogenicity for 71 million missense variants across the human proteome. They carefully calibrated these predictions using a logistic regression model, aligning them with clinically curated pathogenic and benign variants. The calibrated scores, ranging from 0 to 1, represent the likelihood of a variant being clinically pathogenic. Using these scores, variants were classified into three categories: likely pathogenic, likely benign, and ambiguous. This approach significantly expanded the number of confidently classified variants compared to existing models, improving classification precision by 25.8 percentage points on ClinVar test variants compared to the well-performing model EVE. This expansion in confident predictions proves invaluable in the context of proteome-wide variant analysis.
Overall properties and examples of AlphaMissense predictions
AlphaMissense predictions were systematically assessed concerning evolutionary and structural properties. Lower conservation and effective sequence alignments were correlated with reduced predicted pathogenicity. Evolutionarily constrained genes displayed higher predicted pathogenicity for variants. Variants in structured regions, possibly impacting protein stability, exhibited elevated pathogenicity scores. Amino acid substitutions involving key structural elements were more likely to be predicted as pathogenic. The model’s predictions aligned with established biological and structural insights, reinforcing its accuracy. For instance, structurally disordered regions correlate with benign predictions. Protein domains associated with critical functions were less tolerant to mutations, validating AlphaMissense’s predictive power.
AlphaMissense achieves state-of-the-art agreement with multiplexed assays of variant effect
AlphaMissense demonstrates state-of-the-art agreement with multiplexed assays of variant effect (MAVE) data, providing accurate predictions of variant impact on protein function. Comparisons with MAVE data from ProteinGym and an additional benchmark set show that AlphaMissense aligns strongly with experimentally observed variant effects. This includes proteins such as SHOC2 and GCK, associated with diseases like cancer and maturity-onset diabetes of the young (MODY), respectively. Insights into the functional consequences of variations in protein behavior and disease-related traits are gained through AlphaMissense’s accurate capture of domain-level pathogenicity, amino acid characteristics, and their substitution effects. In such assessments, the model performs better than other prediction techniques already in use, demonstrating its accuracy and efficacy in predicting various outcomes.
Decoding AlphaMissense: Using Component Analysis to Uncover Performance Drivers
Essential elements for AlphaMissense’s outstanding performance were found in an ablation study. The study made it clear that the performance of AlphaFold depends on both its pretraining and fine-tuning phases. In addition, it was determined that training data, variant sampling, and structure prediction were important factors in the model’s overall effectiveness. To decrease bias, it was stressed to use a wide variety of training data and sample variants to account for gene bias and enhance model robustness.
Predicting Cell Essentiality with AlphaMissense Gene-Level Pathogenicity
The alignment of AlphaMissense pathogenicity with LOEUF reveals gene intolerance. It accurately identifies genes responsive to functional modifications, especially underpowered genes. Experimentally essential genes significantly outperform LOEUF in identifying gene essentiality, especially in smaller genes, among highly pathogenic AlphaMissense predictions. A thorough method to assess the functional relevance of genes can be created by combining AlphaMissense with population-based approaches. This method is especially useful for short human genes.
AlphaMissense: Empowering the Community with Variant Predictions
The scientific community can benefit from the materials provided by the AlphaMissense initiative. The human proteome is covered by a dataset of 71 million missense variant predictions, which assists with variant prioritization in the diagnosis of uncommon diseases. Predictions provide comprehensive insights for all potential amino acid alterations across canonical human proteins and alternative transcript isoforms, in addition to predictions for gene-level pathogenicity. Prioritized by ACMG, clinically significant genes exhibit good coverage and predictive performance. Additionally, by suggesting potential candidate harmful rare variants for association analysis, AlphaMissense predictions aid research on complex trait genetics. It should improve our knowledge of disease gene discovery, variation effects, and diagnostics.
With the help of Genomics England, AlphaMissense’s precise variant predictions have the potential to improve the identification of uncommon genetic diseases and support the hunt for disease-causing genes, improving medical research and treatment development.
Article source: Reference Paper | AlphaMissense source code is available at Zenodo and GitHub
Learn More:
Prachi is an enthusiastic M.Tech Biotechnology student with a strong passion for merging technology and biology. This journey has propelled her into the captivating realm of Bioinformatics. She aspires to integrate her engineering prowess with a profound interest in biotechnology, aiming to connect academic and real-world knowledge in the field of Bioinformatics.