How much of our body chemistry is related to what we eat can be determined through untargeted metabolomics by comparing blood or stool samples to a reference database of foods.
A team of international scientists, led by investigators at the University of California San Diego, introduced untargeted metabolomics – a new method to determine the large number of molecules obtained from food that were previously unknown but were present in human blood and feces.
As a pseudo-MS/MS reference library, scientists propose reference-data-driven analysis to compare metabolomics tandem mass spectrometry (MS/MS) data to source data annotated with metadata.
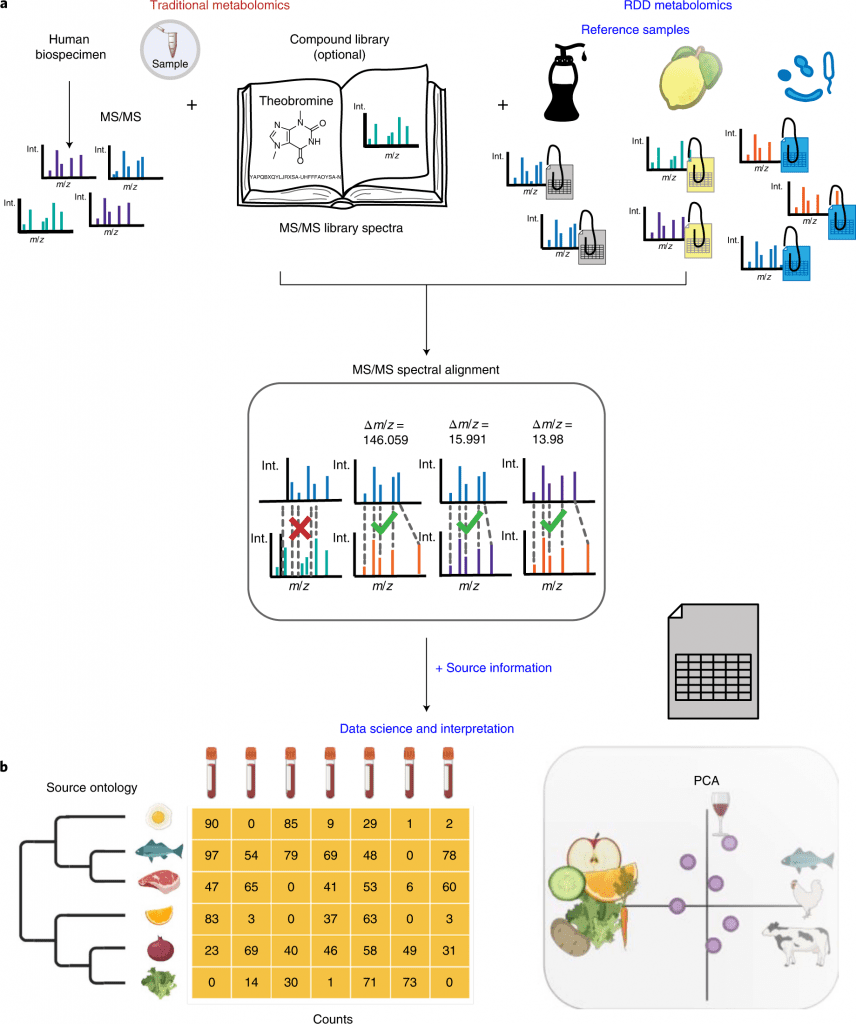
Image Source: https://doi.org/10.1038/s41587-022-01368-1
Only 10% of molecular characteristics are annotated in human untargeted metabolomics investigations.
In order to create a pseudo-MS/MS reference library, the scientists introduce reference-data-driven analysis to compare metabolomics tandem mass spectrometry (MS/MS) data against metadata-annotated source data.
By using food source data to demonstrate this method, they demonstrate how it boosts the use of MS/MS spectrum data by 5.1 times compared to traditional structural MS/MS library matches and enables empirical analysis of dietary patterns from untargeted data.
Interpretation Of Liquid Chromatography-Tandem Mass Spectrometry (LC–MS/MS)-Based Untargeted Metabolomics Data
Both curated gene databases and reference data, such as complete genomes or other sequence data with highly curated metadata, are needed for the interpretation of complex sequence data from metagenomic or metatranscriptomic investigations (developmental stage, tissue location, phenotype, etc.).
This reference data-driven (RDD) methodology uses matches between genes or transcripts of known and unknown origin to improve knowledge of complicated communities.
The majority of metatranscriptomics or metagenomics data must be successfully analyzed, and this requires the RDD approach.
By way of comparison, structural MS/MS library searches are used to evaluate untargeted metabolomics data from LC-MS/MS-based experiments.
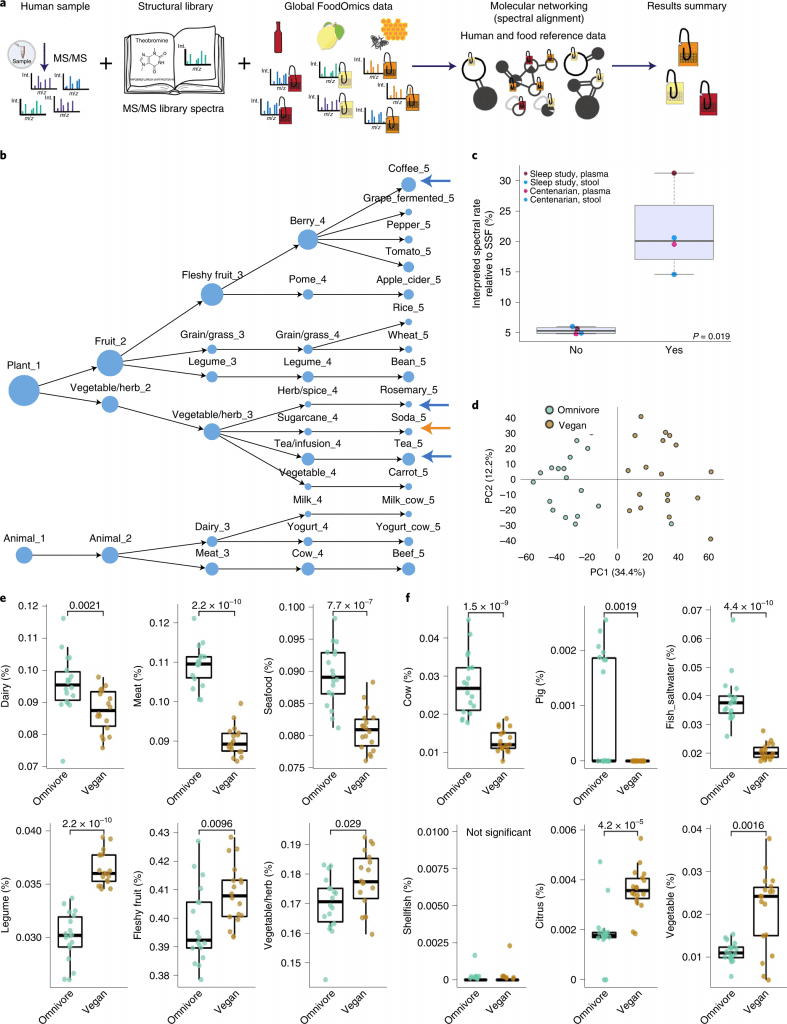
Image Source: https://doi.org/10.1038/s41587-022-01368-1
To improve the insights gained from untargeted MS/MS-based metabolomics, reference data with curated and organized controlled vocabulary metadata is still not used.
Introduction to the RDD Approach
RDD analysis uses all spectra, including unannotated ones, in addition to annotated MS/MS spectra. A step toward RDD has been taken by the gas chromatography-mass spectrometry (GC-MS) BinBase resource. If a spectrum match has been found in a private GC-MS dataset, one can annotate it using BinBase.
However, the metadata cannot be added to contextualized metadata and is not effectively controlled. Additionally, literature mining can be used to identify the source, as we have previously shown using structural annotations.
However, the methods mentioned above to annotate unknowns cannot be utilized to systematically analyze the source data at the dataset level because of the restrictions noted above and/or the impossibility of correlating corresponding spectra in the case of metabolism.
The RDD technique for metabolomics is therefore introduced, followed by a use example illustrating empirical food readouts from untargeted human data.
RDD Analysis Using GNPS
Since the late 1970s, untargeted MS/MS-based metabolomics experiments have involved exploring MS/MS structural libraries or, more recently, examining how an MS/MS spectrum is distributed over public untargeted data.
RDD metabolomics employs all MS/MS spectra from untargeted metabolomics files, which contain hundreds to thousands of MS/MS spectra, for metadata-based source annotation rather than just using one MS/MS spectrum to obtain an annotation.
The output presents contextualized data from source reference datasets, which is one of the main differences.
The contextualized data must be curated using controlled vocabularies for RDD analysis to be successful; otherwise, the findings won’t be suitable for further analysis.
The scientists looked at which dietary compositions may be reconstructed from data obtained from human biospecimens in the RDD application that was shown.
It was necessary to use a source of reference food MS/MS source data and related curated metadata to respond to this inquiry. The source data consists of isotopes, adducts, in-source fragments, multimers, and MS/MS spectra of several ion forms of both known and unidentified molecules.
The curated reference dataset can be matched in human biospecimens via either molecular networking or direct MS/MS spectra matching.
As opposed to static libraries, RDD analysis allows for the addition of customized files or metadata and gives the user control over the processing of the reference data.
Using Global Natural Products Social Molecular Networking (GNPS), the scientists developed a step-by-step tutorial for RDD analysis (https://ccms-ucsd.github.io/GNPSDocumentation/tutorials/rdd/).
Expansion of Food Source Data
The researchers developed a food metabolomics reference dataset to serve as an example of RDD metabolomics since food is essential for health.
In addition to the current state-of-the-art mass spectrometry nutrition readout approaches targeting up to 150-200 metabolites, food frequency and abundance questionnaires, diet records, and 24-hour recalls, which can be self-monitored or assisted by a nutritional specialist, there is an unmet need to retrospectively and empirically read out food and beverage information from human metabolomics data.
The food reference dataset includes around 3,500 items’ worth of extensive and structured metadata as well as untargeted metabolomics.
It combines 1,907,765 spectra into 107,968 distinct MS/MS spectra. By producing and submitting new datasets and metadata to GNPS/MassIVE, the food source data may be easily expanded.
Obtaining Correct Diet Information Using RDD
Food source data for RDD is exposed to GNPS-based molecular networking along with datasets from human metabolomics.
The scientists evaluated whether RDD recovers food known to be consumed using data on the controlled research diets of study participants who participated in sleep and circadian investigation.
Because the individuals in this study were housed for four days on two separate occasions and received a controlled diet, they can determine whether the findings corroborated the known diet from that study.
Eleven of the 15 food categories were exact duplicates of the items that were given to the participants.
Three of those eleven matches were to fermented variations of non-fermented foods, such as fermented grapes in place of grapes, apple cider in place of apples, and yogurt in place of milk.
Four categories were not recorded as being consumed during the study, and three of those could be explained.
Only two volunteers showed signs of consuming caffeinated beverages—one during the first 48 hours and the other once in the middle of the study.
The fact that there were few matches to caffeinated beverages is consistent with their exclusion from the controlled diet.
Rosemary is a frequently used component added to ground meat to prevent oxidation and spoilage, even if it isn’t always listed on the ingredient list of the packages.
The unknown is the origin of the soda matches. This shows that RDD can be used to track diet adherence in controlled-diet research and to successfully get the correct diet information from untargeted metabolomics data.
RDD: Strengths and Applications
RDD makes it possible to read out dietary patterns (for instance, vegan versus omnivore consumption) and the consumption of particular foods.
More generally, RDD can be used to match against any reference database of sources that have been carefully curated and is ontology-aware, including environmental or microbial sources.
As it involves highly scalable molecular networking and the inclusion of specific metadata, RDD metabolomics is currently exclusive to GNPS.
However, it will be feasible to leverage other resources for RDD metabolomics as other analysis ecosystems provide molecular networking capabilities or make RDD compatible with other spectrum alignment procedures.
Specialized tools, like BinBase, may eventually be used for RDD analysis of particular applications or problems because scalable molecular networking for GC-MS is also feasible.
Well-curated datasets of personal care products, medications (not just active ingredients but also formulations), microbial isolates, country of origin, biological sex, age, etc., may also be used as source reference data for RDD metabolomics to broaden its application beyond food readout.
This requires careful curation with controlled vocabularies and the structuring of metadata.
Understanding diet and nutritional intake, exposure risks, medication use, drug use, environmental allergens, pollution studies, microbiome investigations, food ingredients/adulteration, forensics, and personal care product tracing are some potential applications of RDD metabolomics that could be used to inform of potential exposures and health implications.
Article Source: Gauglitz, J.M., West, K.A., Bittremieux, W. et al. Enhancing untargeted metabolomics using metadata-based source annotation. Nat Biotechnol (2022). https://doi.org/10.1038/s41587-022-01368-1
https://ucsdnews.ucsd.edu/pressrelease/you-are-what-you-eat-and-now-researchers-know-exactly-what-youre-eating
Learn More About Bioinformatics:
Top Bioinformatics Books ↗
Learn more to get deeper insights into the field of bioinformatics.
Top Free Online Bioinformatics Courses ↗
Freely available courses to learn each and every aspect of bioinformatics.
Latest Bioinformatics Breakthroughs ↗
Stay updated with the latest discoveries in the field of bioinformatics.
Tanveen Kaur is a consulting intern at CBIRT, currently, she's pursuing post-graduation in Biotechnology from Shoolini University, Himachal Pradesh. Her interests primarily lay in researching the new advancements in the world of biotechnology and bioinformatics, having a dream of being one of the best researchers.