Scientists from Germany have developed SCEMILA, an inherently explainable AI tool for identifying diagnostic cells of acute myeloid leukemia (AML) subtypes. The authors find that the high-attention cells identified by SCEMILA coincide with cells labeled as diagnostically relevant by human experts. The model is trained based on single white blood cell images from digitized blood smears obtained from four categories of acute myeloid leukemia and images obtained from blood smears of healthy stem cell donors as controls. SCEMILA, when applied to classify single cells, was also able to deconvolve a patient’s blood smear to highlight subtype-specific cells without requiring single-cell annotation of the training data.
Artificial Intelligence in Healthcare and Diagnostics
Applications based on artificial intelligence (AI) in the healthcare and diagnostics space is on the rise. This is due to the Deep Neural Networks (DNNs) revolution owing to which feature extraction and classification can be seamlessly intertwined. DNNS have been successfully implemented to extract information from large image data sets with a single-cell resolution to predict cancer patient mortality, discriminate cancer types, and classify single blood and bone marrow cells. DNNs have also been used for distinguishing between leukemia subtypes with very high accuracy. Such models have displayed an impressive performance in classification, however, the exact mechanism behind the classification is not clear, and thus they are known as the black box models.
Explainable AI and Why it is Preferred
Previous methods have applied attention mechanisms for identifying relevant regions in large histological scans. These methods achieved qualitative agreement between diagnostically relevant images and high-attention algorithmic image patches. However, a quantitative comparison between the two remains elusive. A plethora of methods highlights relevant image areas using posthoc explainability at the pixel level. However, their usefulness and reliability have been greatly criticized by the scientific community.
Using a featured-based approach alongside DNNs could lead to identifying important features and also aid in instructing human experts. The authors implement an inherently explainable AI model for identifying AML subtypes from single-cell images of patient blood smears.
Acute Myeloid Leukemia (AML) Diagnosis
Early identification of AML is crucial for patient survival as well as for determining therapeutic measures. Identifying the AML subtype is morphology-based and requires microscopic inspection of the patient’s bone marrow and blood smears. While typical AML identification involves identifying more than 20% of WBCs (white blood cells) as blast cells, specific subtype identification relies on identifying specific cell anomalies. These cells are usually low in number and hence pose a significant challenge in identifying them.
SCEMILA: Single-cell based Explainable Multiple Instance Learning Algorithm
The application of explainable AI for AML subtype identification is justified for the following two reasons. Firstly, the AML subtype information is present in the patient information, which provides annotated training data without label noise. Secondly, morpho-genetic correlations between the atypical cells and the PML::RARA fusion for APL, a genetic subtype of AML, are well established and thus aid in model validation.
The workflow involves the following components:
- Single-cell feature extraction: The author used ResNet34 for single-cell feature extraction using over 300,000 annotated single-cell images from a white-blood-cell dataset.
- Attention-based Multiple Instance Learning (MIL): MIL involves classification based on available labels at the bag level rather than at the instance level. Here, the patient diagnosis (bag level) label is available, but instance level (single-cell level) labels are not. Hence, it is appropriate to apply MIL in this case. The authors implement a permutation invariant method for the analysis of a set of single-cell images of a particular patient and return the AML subtype.
- Algorithm training involved a 5-fold cross-validation approach after the algorithm was randomly initialized.
- An expert hematologist annotated all single-cell images from one patient from each subtype. This was to ensure the diagnostic correlation with the algorithm’s single-cell attention.
- The authors used UMAP for generating low-dimensional embeddings.
- SCEMILA also performed single-cell classification generating single-cell AML subtype predictions.
The following figure illustrates the usefulness of SCEMILA.
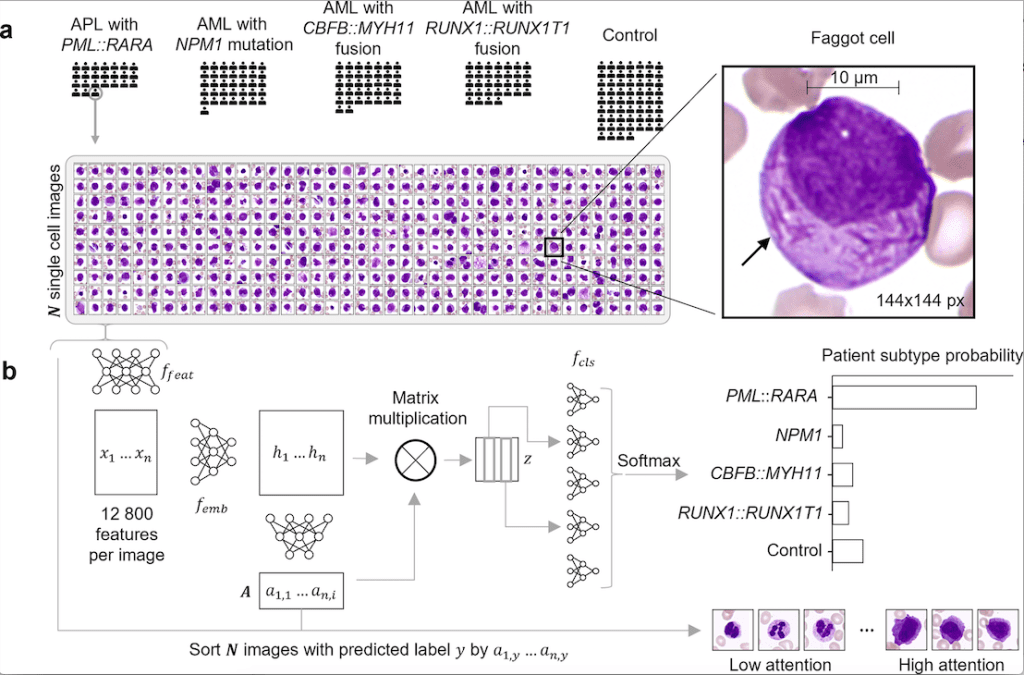
Image Source: https://doi.org/10.1371/journal.pdig.0000187
Usefulness of SCEMILA
The clinical relevance and usefulness of SCEMILA are paramount. It clearly has the potential to support the clinical workflow by identifying diagnostically rare and relevant images. This will result in increased speed in identifying disease subtypes and hence make therapy easily accessible. The following results illustrate it further
- It classifies four AML genetic subtypes accurately.
- The method results in diagnostically relevant cells gaining high attention.
- The method deconvolves patient blood smear to identify cell subtypes.
- It identifies subtype-specific single-cell features.
Conclusion
Computational blood smear analysis aids in cytological diagnosis speed. With SCEMILA, the authors implemented an inherent explainable AI algorithm that reliably and accurately identifies four genetic subtypes of AML, a life-threatening disease. With SCEMILA, the speed of diagnosis is highly improved, and this will certainly facilitate early clinical intervention and better disease management. However, it is limited by the fact that it can only identify the specific four subtypes currently and not any new subtypes. However, in future iterations, this can be addressed. Also, in the future, SCEMILA could be tweaked for analyzing bone marrow smears as well. The black box model alternative, SCEMILA, is a game-changer in AML patient diagnosis and an effective supporting tool for clinicians.
Article Source: Reference Paper
Learn More:
Banhita is a consulting scientific writing intern at CBIRT. She's a mathematician turned bioinformatician. She has gained valuable experience in this field of bioinformatics while working at esteemed institutions like KTH, Sweden, and NCBS, Bangalore. Banhita holds a Master's degree in Mathematics from the prestigious IIT Madras, as well as the University of Western Ontario in Canada. She's is deeply passionate about scientific writing, making her an invaluable asset to any research team.