Scientists present an interpretable, loosely guided, multimodal deep learning strategy for cancer prognosis, which also investigates the use of multimodal interpretability to describe how WSI and molecular characteristics affect risk.
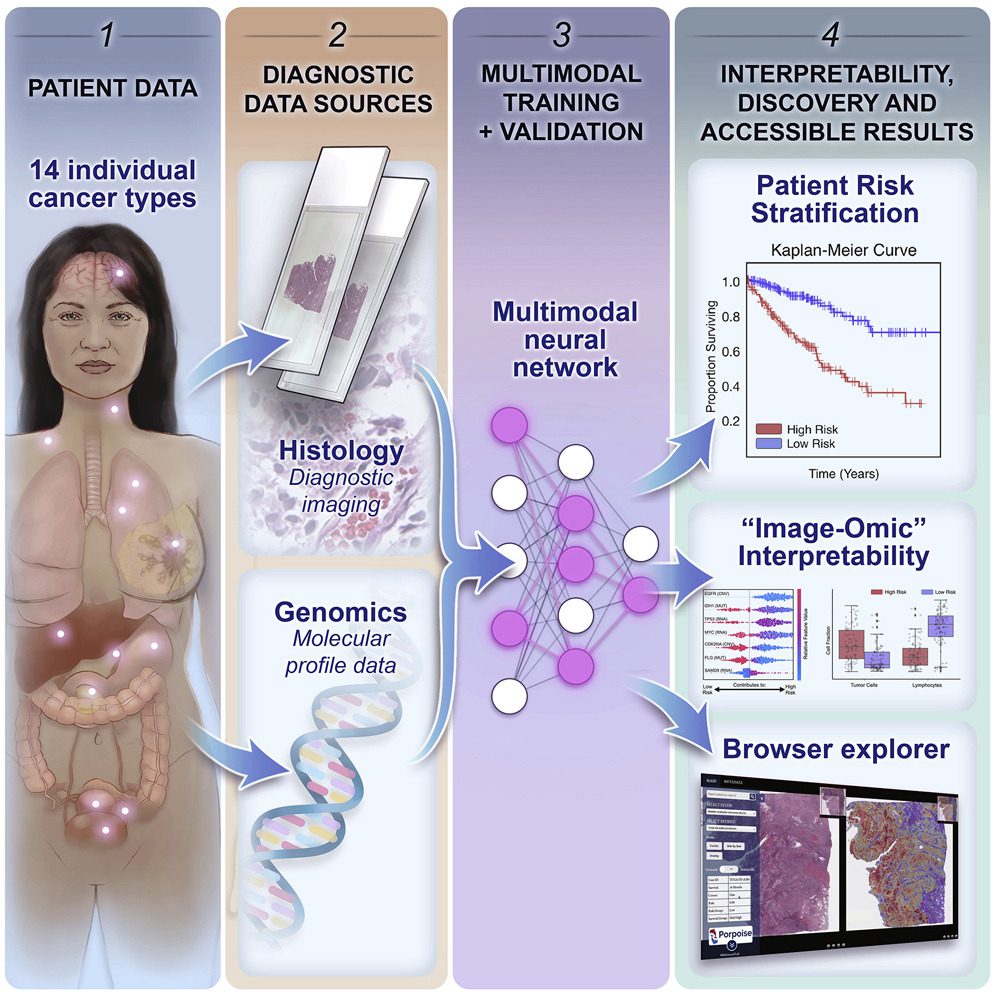
The objective prognostic models that can be created from histology pictures have shown promise in the quickly evolving field of computational pathology.
Most prognostic models, however, are based solely on histology or genomes and do not consider how these data sets might be combined to create joint image-omic prognostic models.
It is also interesting to determine the explainable morphological and molecular characteristics that control this prognosis from these models.
Using multimodal deep learning, the scientists concurrently analyze molecular profile data from 14 cancer types and pathology whole-slide images.
Their multimodal weakly supervised deep learning algorithm can combine these disparate modalities to forecast outcomes and identify prognostic features that correspond with good and bad outcomes.
The researchers publish their findings on morphological and molecular correlates of patient prognosis across 14 cancer types in an interactive open-access database for further study, biomarker development, and feature evaluation.
A Lacking Comprehension of Contribution of Tumor Microenvironment towards Patient Risk
Characteristic histopathological, genomic, and transcriptomic heterogeneity in the tumor and tissue microenvironment, which affects therapy response rates and patient outcomes, is what defines cancer.
For many cancer forms, the current clinical paradigm involves manually assessing histopathologic traits such as tumor invasion, anaplasia, necrosis, and mitosis.
Once the patients have been divided into various risk categories, these characteristics are utilized as grading and staging criteria to decide on the best course of treatment. For instance, the tumor, nodes, and metastases (TNM) staging system classify primary tumors according to their severity (e.g., size, growth, atypia), which is then used to plan treatments, identify patients who are candidates for surgery, choose how much radiation to administer and make other treatment choices.
However, it has been shown that there is significant inter- and intra-observer heterogeneity in the subjective assessment of histopathologic characteristics, and individuals with the same grade or stage still have a wide range of outcomes.
Despite the fact that numerous histopathologic biomarkers have been developed for diagnostic purposes, the majority of them are based solely on the morphology and distribution of tumor cells and lack a detailed understanding of how the spatial arrangement of the stromal, tumor, and immune cells in the larger tumor microenvironment affects patient risk.
Potential of Deep Learning for Automated Biomarker Discovery
Recent advances in deep learning for computational pathology have enabled the use of whole-slide images (WSIs) for automated cancer diagnosis and evaluation of morphologic aspects in the tumor microenvironment.
Using poorly supervised learning, slide-level clinical annotations can instruct deep-learning systems to recapitulate typical diagnostic tasks, such as cancer detection, grading, and subtyping.
Even though such algorithms can outperform human experts for narrowly defined problems, their ability to quantify novel prognostic morphological features is limited because training with subjective human annotations may fail to extract previously undetected properties that could be used to improve patient prognosis.
Recent deep-learning-based approaches suggest supervision using outcome-based labels such as disease-free and overall survival times as ground truth to collect additional objective and predictive morphological features not extracted in typical clinical procedures.
Recent research has demonstrated that applying deep learning for automated biomarker discovery of novel and prognostic morphological determinants has great potential.
Deciding Targeted Molecular Therapies without NGS
In the larger context, cancer prognosis is a multimodal problem that is driven by markers in histology, clinical data, and genomes, even if prognosis morphological biomarkers may be clarified utilizing outcome-based labels like supervision in WSIs.
The use of molecular biomarkers in prognostication has increased the complexity of treatment decision-making processes for many cancer types since the advent of next-generation sequencing and the introduction of targeted molecular medicines.
For instance, the existence of EGFR exon 19 deletions and exon 21 p.Leu858Arg substitutions indicate that erlotinib should be used to treat EGFR mutant lung and pancreatic cancers.
When employed in conjunction with histological evaluation, joint image-omic biomarkers, such as oligodendroglioma and astrocytoma histologies with IDH1 mutation and 1p/19q-codeletion status, can conduct fine-grained categorization of patients into low-, intermediate-, and high-risk categories.
Determining deep learning-based multimodal fusion of molecular biomarkers and extracted morphological features from WSIs may improve patient risk stratification accuracy and aid in discovering and validating multimodal biomarkers in cases where the combined effects of histology and genomic biomarkers are unknown.
Recent multimodal studies on the TCGA have concentrated on discovering genotype-phenotype connections using histology-based prediction of molecular abnormalities, which can help patients choose targeted molecular therapy without next-generation sequencing.
PORPOISE: Goals
Clinical staging approaches that incorporate computationally generated histomorphological indicators have great promise for improving patient risk categorization.
The TNM classification system, one of the current cancer staging methods, struggles with accuracy and consistency, impacting patient management and results.
Researchers integrate WSIs and molecular profile data for cancer prognosis using a strategy for interpretable, weakly supervised, multimodal deep learning in this study.
The researchers used paired molecular profile data from 6,592 WSIs from 5,720 patients to train and validate their strategy and compared it to Cox models with clinical variables and unimodal deep-learning models.
Their method outperformed these models on 12 of the 14 cancer types in a one-versus-all comparison.
Their approach investigates multimodal interpretability to explain how WSI and molecular characteristics affect risk.
The researchers created PORPOISE, a freely accessible interactive application that uses their model to immediately produce WSI and molecular feature explanations for each of the 14 cancer kinds.
With PORPOISE, the scientists hope to start transforming the present state-of-the-art black-box computational pathology techniques into more accessible, understandable, and practical ones for the larger biomedical research community.
The researchers envision that by making available heatmaps and decision plots for each cancer form, their tool will enable academics and physicians to develop their theories and further explore the findings made possible by deep learning.
Although multimodal integration improves patient risk stratification for the majority of cancer types, their results also indicate that for some cancer types, training unimodal algorithms using either WSIs or molecular features alone may achieve comparable stratification performance, as the variance of cancer outcomes can be equally captured in either modality.
Unimodal deep-learning-based cancer-prognosis algorithms may have lowered obstacles to clinical deployment in this situation because practical settings may not include paired diagnostic slides or high-throughput sequencing data for the same tissue samples.
Despite the fact that the integration of audio, visual, and linguistic modalities has been successful in technical fields, the researchers emphasise that for clinical translational tasks, the basis of improvement from multimodal integration must be grounded in the biology of each type of cancer, because phenotypic manifestations in the tumor microenvironment that are entirely explained by genotype contributions have a high mutual information.
Their findings suggest that multimodal integration should be applied per-cancer basis, which may help introspect clinical issues for unimodal or multimodal biomarkers on single disease models. The researchers established unimodal and multimodal baselines for 14 different cancer types.
The Endpoint
The given study demonstrates the creation of multimodal prognostic models from orthogonal data streams using weakly supervised deep learning and identifying correlating features that drive such prognosis.
Future research will concentrate on creating prognostic models that are more narrowly focused by selecting larger multimodal datasets for specific disease models, modifying models to fit large independent multimodal test cohorts, and utilizing multimodal deep learning to predict treatment response and resistance.
When combined with whole-slide imaging, sequencing technologies like single-cell RNA-seq, mass cytometry, and spatial transcriptomics continue to develop and become more widely used.
As a result, their understanding of molecular biology will become more spatially resolved and multimodal.
The multimodal learning technique introduced in the study is regarded as a “late fusion” design when it uses bulk molecular profile data, where unimodal WSIs and molecular characteristics are fused close to the output end of the network.
Nevertheless, spatially resolved genomics and transcriptomics data along with whole-slide imaging have the potential to enable “early fusion” deep-learning techniques that combine local histopathology image regions and molecular features with precise spatial correspondences, leading to more reliable characterizations and spatial organization mappings of intratumoral heterogeneity, immune cell presence, and other morphological determinants.
Article Source: Chen, Richard J. et al. (2022). Pan-cancer integrative histology-genomic analysis via multimodal deep learning. Cancer Cell, Volume 40, Issue 8, 865 – 878.e6 https://doi.org/10.1016/j.ccell.2022.07.004
Learn More:
Top Bioinformatics Books ↗
Learn more to get deeper insights into the field of bioinformatics.
Top Free Online Bioinformatics Courses ↗
Freely available courses to learn each and every aspect of bioinformatics.
Latest Bioinformatics Breakthroughs ↗
Stay updated with the latest discoveries in the field of bioinformatics.
Tanveen Kaur is a consulting intern at CBIRT, currently, she's pursuing post-graduation in Biotechnology from Shoolini University, Himachal Pradesh. Her interests primarily lay in researching the new advancements in the world of biotechnology and bioinformatics, having a dream of being one of the best researchers.