Scientists from Peking University, China, presented a new protein-protein docking framework, ColabDock, that incorporates experimental restraints into protein complex structure modeling with an aim to circumvent incongruity between experiment and prediction through integrating AlphaFold2 (AF2) energy function and restraints posteriori, outcompetes previous FFT (Fast Fourier Transform)-based protein-protein docking algorithms, and has the potential to generate structures resembling the native form. ColabDock can also execute antigen-antibody interface prediction.
A Brief about the ColabDock Model
Instead of implementing the FFT (Fast Fourier Transform) algorithm like ZDOCK, pyDock, SwarmDock, HADDOCK, and ClusPro; ColabDock applies gradient backpropagation and also automatically integrates the AF2 energy function and restraints posteriori. It follows a segment-based optimization protocol to manage longer proteins, as backpropagation consumes large GPU memory and limits the conceivable protein length. Moreover, the framework consists of two stages, which are the generation stage and the prediction stage.
The generation stage adopts a protein design protocol framework developed based on AF2, named ColabDesign. In this stage, the input sequence of templates of each chain in the complex optimizes in the logit space to develop a complex structure corresponding to the individual structures of each component and the mentioned restraints through updating the input sequence while maximizing pLDDT and pAE criteria. ColabDock adopts four types of losses during the generation stage that are monomer distogram loss, restraint loss, pLDDT loss, and ipAE loss.
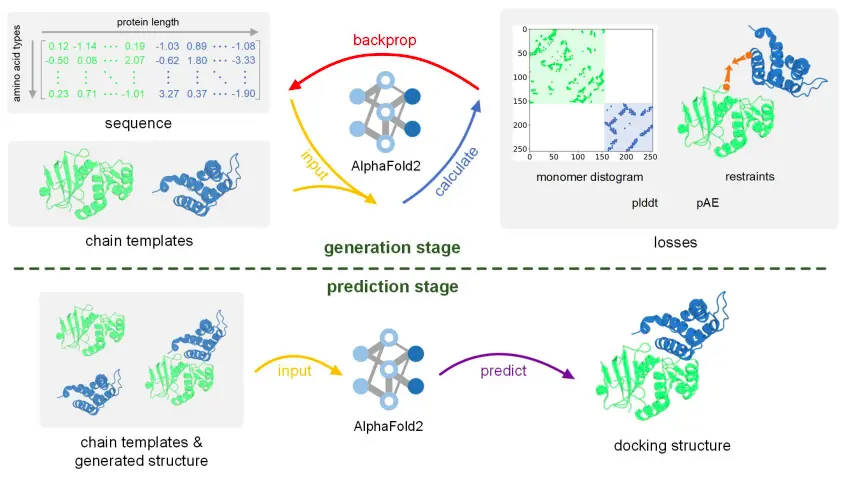
Image Source: https://doi.org/10.1101/2023.07.04.547599
The final structure is predicted using AF2, based on the generated complex structure and individual component structures during the prediction stage. The framework undergoes multiple runs for each protein and generates multiple conformations. Using the RankingSVM algorithm, the best conformation is chosen. DockQ and Cα-RMSD are the two criteria associated with the model evaluation in ColabDock. DockQ depicts the quality of the interface, while the Cα-RMSD measures the global structural difference.
Understanding the Context Behind Developing ColabDock Model
Solving protein structure alone cannot address the fundamental questions regarding cellular machinery and can’t establish a comprehensive understanding of the cellular landscape. Since proteins interact and form complexes to execute their destined responsibility, obtaining structural information about such protein-protein complexes is cardinal for depicting how complex phenomena happen inside a cell.
X-ray crystallography, nuclear magnetic resonance (NMR) spectroscopy, cryo-electron microscopy, etc., are principal experimental approaches for gathering structural information about protein assembly, but experimental approaches are time-consuming, laborious, and error-prone and thus subjected to limiting conditions. Conjugating the previous findings and technology, computational approaches are the alternative attempt to assist computational biologists.
In this regard, the researchers here mention the Fast Fourier Transform (FFT)-based algorithm that is applied to generate conformations for protein-protein docking, and the scoring functions help to determine the quality of the prediction. Such programs deliver moderate accuracy. Restraints derived from experimental methods are incorporated into docking algorithms to counteract the problem.
Many restrained docking algorithms have also been formulated, such as the HADDOCK framework, which can define the active residues that directly interact with other subunits and the passive residues likely to make contact in the protein complex. Another program, ClusPro, generates a feasible translation set for each restraint and selects translations from the intersection set with a frequency larger than a cutoff.
Moreover, a deep learning based model like AF2 makes ab-initio structure prediction learning from an approximate biophysical energy function from massive protein structure data possible and has achieved state-of-the-art performance in protein model quality estimation. However, the authors enunciate that protein complex prediction with Alphafold-Multimer is limited, and prediction is often inconsistent with experimental observations.
Therefore, the researchers propose ColabDock in an attempt to omit such discrepancies by replacing the FFM-based algorithm, integrating sparse experimental restraints into the AF2 derived energy function. Predicting the cognate antigen and antibody interaction is very challenging, the ability of ColabDock can also address this problem with emulated interface scan restraints and is potentially applicable for antibody design.
Conclusion
ColabDock fulfills accurate restrained protein interface prediction by optimizing the input sequence under the designed loss to generate complex structures that are in accord with the provided experimental restraints and refining the overall structure with AlphaFold. Therefore, it is flexible enough to accommodate different restraints as input. As an example, in this paper, the researchers demonstrated the performance of the framework with two types of restrain which are the distance of a residue pair below a certain threshold and the other restrain due to chain multiplicity, surface coverage, and noise.
ColabDock surpasses HADDOCK and ClusPro, both of which performed excellently in the CAPRI (Critical Assessment of PRediction of Interactions) in complex structure predictions with simulated residue and surface restraints, as well as in the prediction assisted by NMR chemical shift perturbation along with covalent labeling, and has confirmed robustness and high accuracy. While the number of looser restraints decreased, the performance of ColabDock displayed a significant improvement compared to that of HADDOCK and ClusPro, indicating that ColabDock relies weakly on the quality of restraints.
Although ColabDock can only handle complexes with less than 1200 residues and can only accept restraints on residue pairs at a distance below 22 Å. Apart from that, ColabDock can utilize different types of restraint information effectively and has the potential to generate native-like protein complex structures that use information from restraints and fit well with the provided experimental evidence. Overall, the mindful application ColabDock can facilitate novel findings.
Article Source: Reference Paper | The ColabDock code will be available on GitHub
Important Note: bioRxiv releases preprints that have not yet undergone peer review. As a result, it is important to note that these papers should not be considered conclusive evidence, nor should they be used to direct clinical practice or influence health-related behavior. It is also important to understand that the information presented in these papers is not yet considered established or confirmed.
Learn More:
Aditi is a consulting scientific writing intern at CBIRT, specializing in explaining interdisciplinary and intricate topics. As a student pursuing an Integrated PG in Biotechnology, she is driven by a deep passion for experiencing multidisciplinary research fields. Aditi is particularly fond of the dynamism, potential, and integrative facets of her major. Through her articles, she aspires to decipher and articulate current studies and innovations in the Bioinformatics domain, aiming to captivate the minds and hearts of readers with her insightful perspectives.