Scientists from Shanghai have developed a de novo drug design method, QADD (quality assessment-based drug design approach). The multi-objective reinforcement learning-based method is capable of designing chemical molecules with desired properties using a novel Quality Assessment (QA) module. The novel drug design framework is able to cover a large territory of the chemical space that was previously unexplored. Instead of using a weighted sum of objective functions as in previous methods for drug design, QADD incorporates a multi-objective framework to jointly optimize multiple properties. The molecular QA model is an effective discriminative model capable of estimating good drug potentials for generated molecules. The authors also generate novel molecules with high binding affinity for biological targets such as DRD2 (dopamine receptor type 2).
Previous Drug Design methods and why we need the novel method QADD
Drug discovery initiates with the generation of potential drug candidates. Due to inefficient and deficient exploration of the chemical space, many potential drug candidates remain unmined, thereby limiting the number of molecules deposited in databases. A large number of potential drug-like molecules remain undiscovered as a consequence. The ChEMBL database houses approximately two million bioactive molecules with drug-like properties, of which the FDA has approved only 43,264 as drugs. This calls for method development for novel drug design that covers the chemical space extensively.
Wet laboratory experiments for novel drug discovery are time-consuming, and with the advent of technology and high-performance computing, a plethora of computational methods for drug design and discovery have been developed. Such methods include REINVENT, MolGAN, and others implementing deep learning techniques for molecule generation with desired properties.
These deep learning methods can be broadly classified into two categories. The first group of methods uses deep generative models to learn the distribution of the training molecules and generates similar novel molecules. An Auto-Encoder-Decoder based method uses the SMILES format to propose new molecules into a low -dimensional latent space using the Encoder and then samples molecules from the latent space and reconstructs molecules using the Decoder as demonstrated by Blaschke et al. The General Adversarial Networks (GANs) comprise a generator network and a discriminator network and have been used in MolGAN to propose molecular graphs. The generator outputs a molecular graph from a feature vector sampled with a prior, and the discriminator determines if the graph is from the training set or the generator. Methods implementing bidirectional recurrent neural networks (BiRNNs) have also been developed by Grisoni et al.
The second group of methods is based on optimizing objective functions derived from a combination of multiple properties. These methods are typically based on the Reinforcement learning paradigm, given the good optimization and exploration abilities of the methodology. Such methods include ReLeaSE. These methods typically use a weighted combination of objective functions rendering a dominant objective function. Also, such methods are limited by the fact some high potential drug-like molecules are ignored as they do not meet these optimized properties. Thus, an effective Quality Assessment module is required for assessing the potential of drug-like molecules. Hence, with these limitations in mind, the authors developed QADD (Quality Assessment-based Drug Design), an iterative refinement framework combining a multi-objective deep reinforcement learning generator with a novel QA discriminator for de novo drug discovery.
A brief overview of the method QADD: Quality Assessment-based Drug Design
The QADD pipeline involves the following steps:
- The multi-objective deep reinforcement learning model estimates the value function of the generated molecules and chooses the most appropriate action at each step to maximize the discounted return.
- The QAscore scored by the molecule quality assessment model serves as one of the reward functions of the multi-objective deep reinforcement learning model, whose generated molecules are fed back to retrain the GNN (Graphical Neural Network)-based QA model iteratively.
- Finally, the generated molecules are further modified using functional group modification.
The following figure illustrates the QADD pipeline.
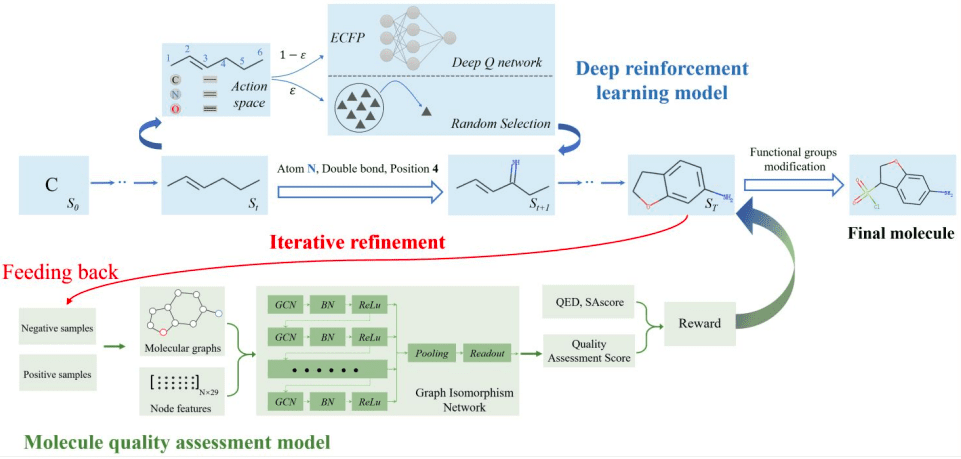
Image source: https://doi.org/10.1093/bioinformatics/btad157
Generating novel molecules with high binding affinity to DRD2
The QADD methodology avoids information loss by optimizing multiple objective functions in parallel. The molecular QA module effectively discriminates and learns the distribution of molecules with high drug potential. The authors also generate molecules with high binding affinity to DRD2( Dopamine receptor type2). This is achieved using a binding prediction model designed to score target-specific binding affinity as one reward function of QADD. This module can be used to generate novel molecules for any biological targets.
Conclusion
The novel drug design methodology, QADD, is a game changer in the drug design and development field. It combines a reinforcement learning-based generator model with a molecular QA model discriminator in a synergistic way for generating de novo molecules as potential drug molecules. The GNN-based novel QA module ensures the generation of high potential drug molecules. The iterative refinement framework improves the quality of the molecules iteratively. The high efficacy of the method, coupled with the ability to span a greater scope of the chemical space, renders it as a groundbreaking method that will aid in the discovery of novel drug-like molecules and advance the development of better therapeutics. The ability of the method to generate molecules with high binding affinity for DRD2 also opens doors to finding such molecules for other biological molecules of interest as targets, thereby contributing significantly to drug design, development, and delivery.
Article Source: Reference Paper
Learn More:
Banhita is a consulting scientific writing intern at CBIRT. She's a mathematician turned bioinformatician. She has gained valuable experience in this field of bioinformatics while working at esteemed institutions like KTH, Sweden, and NCBS, Bangalore. Banhita holds a Master's degree in Mathematics from the prestigious IIT Madras, as well as the University of Western Ontario in Canada. She's is deeply passionate about scientific writing, making her an invaluable asset to any research team.