Researchers from Trinity College Dublin have introduced a machine-learning model that classifies human macrophages based on cellular autofluorescence imaging of NAD(P)H. They have also shown that this technique is powerful for analyzing macrophage metabolism and classification.
The Two-photon fluorescence lifetime imaging microscopy (2P-FLIM) is a non-invasive imaging technique that aids in visualizing cellular metabolism by tracking the fluorophores. Fluorescence lifetime is a fluorophore’s time to reach the ground state from an excited state. Co-enzymes such as NAD(P)H, FAD+, and the amino acid Tryptophan are the autofluorescence markers used to measure changes in intracellular metabolism.
On the other hand, macrophages are essential immune cells involved in innate immunity, normal tissue development, homeostasis, and tissue repair. There are two major types of macrophages,
1) M1 Macrophages – Interferon Gamma (IFNγ) or Lipo Polysaccharides (LPS) classically activate these macrophages. This activation leads to the production of pro-inflammatory cytokines by the M1 to phagocytose the pathogens.
2) M2 Macrophages – These are activated by cytokines such as Interleukin 4 (IL-4) or Interleukin 10 (IL-10) and induce cell proliferation.
Phenotypic macrophage behavior is assessed by techniques such as measuring cytokines released due to the stimulation of macrophages, staining cellular surface markers, and gene analysis. But in recent years, novel techniques such as Raman microscopy and fluorescence lifetime have been used to visualize the macrophage behavior in a non-invasive way.
In the current study, scientists have utilized 2P-FLIM imaging of NAD(P)H to understand the macrophages’ metabolic state in real-time. For data visualization, an algorithm called the Uniform Manifold Approximate and Projection (UMAP), and for statistical analysis, machine learning algorithms were used.
Findings
By applying sophisticated imaging techniques to create single-cell metrics that are grouped and verified using machine learning classification models, human blood-derived macrophage polarisation may be categorized by means of their metabolism.
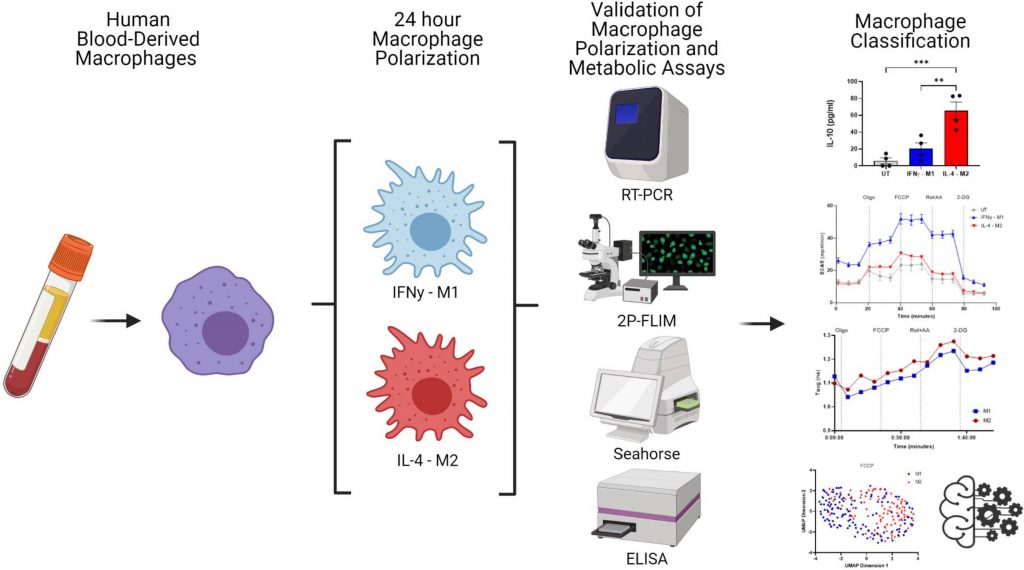
Image Source: https://doi.org/10.7554/eLife.77373
The process through which macrophages create distinctive functional phenotypes in response to specific microenvironmental stimuli is known as “macrophage polarisation.”
Higher expression of Tumor necrosis factor α (TNFα) and IFNγ and low expression of IL-10 signifies classically activated M1 cells, whereas high production of IL-0 and higher expression of Mannose receptor C type 1 (MRC1) and Chemokine ligand 13 (CCL13) signifies the alternately activated M2 cells. Flow cytometry was performed to validate the presence of the markers indicating macrophage polarization.
Next, 2P-FLIM was used for the non-invasive evaluation of macrophage polarization. UMAP was integrated with 2P-FLIM variables associated with M1 and M2 macrophages for data visualization purposes.
The popular machine learning algorithm Random Forest is a part of the supervised learning methodology. One may apply it to ML issues involving both classification and regression. Random Forest is a classifier that uses many decision trees on different subsets of the provided dataset and averages the results to increase the dataset’s predicted accuracy. Overfitting is prevented by the larger number of trees in the forest, thus leading to high accuracy. Neto et al. used the random forest model to classify FCCP-treated (p-trifluoromethoxy carbonyl cyanide phenyl hydrazone) macrophage polarization. The model successfully predicted macrophage polarization with an area under the curve of 0.944 when the 2P-FLIM variables are considered predictors (τ1, τ2, α1, α2 and τavg, fluorescence lifetime variables). The computational model showed high sensitivity to cell-to-cell variability in macrophages in response to FCCP treatment.
Concluding Remarks
Future prospects include increasing the total number of cells analyzed, and other modes of tagging, including IFNγ-M1 and IL4-M2, can help improve accuracy. Imaging using 2P-FLIM enables spatio-temporal resolution of cellular metabolism in a non-invasive manner. No fixing or staining is required, and a small subset of cells is sufficient for analysis. Overall in the discussed study, 2P-FLIM was used as a means to classify cellular metabolic states and fluorescence lifetime. 2P-FLIM with ML models clearly distinguished M1 and M2 types of macrophages treated with FCCP. This methodology can be extrapolated to other cell lines and organoid models for clinical applications and drug validation.
Article Source: Reference Paper
Learn More:
Top Bioinformatics Books ↗
Learn more to get deeper insights into the field of bioinformatics.
Top Free Online Bioinformatics Courses ↗
Freely available courses to learn each and every aspect of bioinformatics.
Latest Bioinformatics Breakthroughs ↗
Stay updated with the latest discoveries in the field of bioinformatics.
Shwetha is a consulting scientific content writing intern at CBIRT. She has completed her Master’s in biotechnology at the Indian Institute of Technology, Hyderabad, with nearly two years of research experience in cellular biology and cell signaling. She is passionate about science communication, cancer biology, and everything that strikes her curiosity!