The novel clustering algorithm HAL-x provides insights into single-cell clustering, bridging the biomedicine sciences for healthcare purposes. With its specificity and sensitivity, it allows the generation of multiple-clustering for in-depth analysis of high-dimensional data. The future directions of algorithms are extremely promising in understanding disease, disease progression, and drug discovery.
Throughout the years, clustering has been studied both extensively and intensively in statistics and machine learning. Apart from classical methods, clustering for high-dimensional data is primarily done using non-linear embeddings, which largely lacked visualization and identification of accurate clustering. Followed by it, various high-dimensional algorithms have surfaced since then, such as PARC and FlowSOM. These algorithms extensively use large data machinery, often adding to the high machinery cost and selective availability.
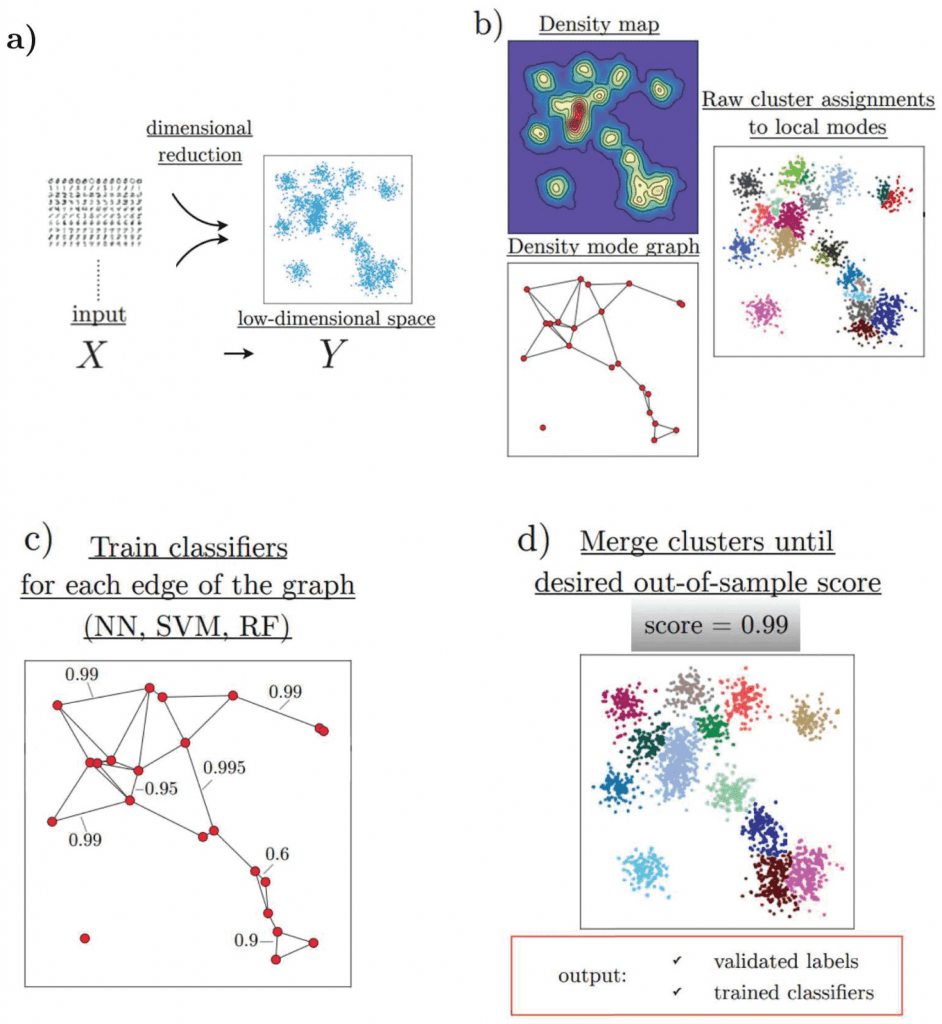
Image source: https://doi.org/10.1371/journal.pcbi.1010349
In order to overcome limitations displayed by earlier clustering methods, researchers from the National Cancer Institute, Maryland, and Boston University, USA, proposed a reliable and efficient algorithm to decipher questions from single-cell population analysis. The hierarchy density clustering method utilizes linkage methods to build a cluster ranking from raw data. Since clustering requires prior knowledge of datasets to allow the distinct populations of cells to align and provide meaningful results.
Hence, the researchers focused on mass spectrometry data (CyTOF) to apply more than 30 features to each single biological cell type sample. Unlike other limitations of downsized data and the least efficient trained dataset. The current method of HAL-x employs supervised linkage methods wherein the ultimate prediction of the true class labels is determined from the clustering. The supervised learning model utilizes random forest and support vector machine to classify potential clusters and their performance outcomes.
HAL-x has four major components, which efficiently allow the clustering of high-dimensional biological datasets:
- HAL-x applies a dimensional reduction procedure (t-SNE algorithm).
- It uses the nearest neighbor algorithm to identify an early set of ‘pure clusters.’
- It defines the density-based neighborhood for each pure cluster.
- It builds a sparsely connected k-nearest neighbor (KNN) graph that connects each cluster with a similar k-cluster.
HAL-x has been designed to cluster datasets with embedded data points up to 100 million points across 50 and more dimensional space. As designed by the researchers, it uses the z-score technique to normalize and downsize the sample of data. In order to identify the pure clusters, the algorithm identifies the initial set as ‘pure’, these high-density clusters further form the starting of agglomerative clustering. Low-dimensional embedding was used for this step, using a variation proposed by the team to overcome the limitations. Upon identification of these clusters performs, the second step for validation is using two separate protocols.
As the data is clustered, the algorithm applies agglomerative clustering by linking clusters that are difficult to distinguish. This classification is done by training the random forest and support vector. Furthermore, these are connected with the KNN graph. As the ensemble is set, the algorithm uses a tree data structure to predict multiple clusters for unreduced data points. To determine the predictions, this clustering is applied to all datasets. Successive predictions are made using classifiers by merging all the data.
Real-life dataset: Lupus
One of the real-life datasets was establishing the scalability and reliability of the HAL-x algorithm on a mass cytometry dataset of 25.9 million individual cells analyzed from PBMC (peripheral blood mononuclear cells) samples. To determine the differential populations based on potential biomarker discovery or immunotherapy, the team suggested the generation of 5 clusters with different populations.
For each cluster, researchers trained the classifier to separate healthy and lupus cells. Multiple clusters were identified for specific immune cells with altered phenotypes, which the group believes can be subsequently used as novel biomarkers for the detection of autoimmune disease. Upon administration of wet-lab protocols, these can highlight the relevance of targeted immunotherapy and other interventions for drug discovery.
Final Thoughts
While the study has its limitations, the researchers have directed them toward future implications with better interaction of raw data and a better-trained dataset which can be applied to supervised classification such as the phenotypic population of cells for its future prospects in screening drugs and diagnostic markers. Since the HAL-x algorithm does not use large amounts of memory space, it simply outturns the use of specialized computing resources, simplifying usage easy for biologists.
Article Source: Reference Paper | HAL-x is Publicly Available at: HAL-x | Raw Data: Lupus Dataset
Learn More:
Top Bioinformatics Books ↗
Learn more to get deeper insights into the field of bioinformatics.
Top Free Online Bioinformatics Courses ↗
Freely available courses to learn each and every aspect of bioinformatics.
Latest Bioinformatics Breakthroughs ↗
Stay updated with the latest discoveries in the field of bioinformatics.
Mahi Sharma is a consulting Content Writing Intern at the Centre of Bioinformatics Research and Technology (CBIRT). She is a postgraduate with a Master's in Immunology degree from Amity University, Noida. She has interned at CSIR-Institute of Microbial Technology, working on human and environmental microbiomes. She actively promotes research on microcosmos and science communication through her personal blog.