Scientists from Pennsylvania State University integrated 3D genomic and epigenomic data with transcriptome-wide association studies data to improve gene expression predictions.
The researchers propose an integrative method PUMICE that integrates 3D genomic and epigenomic data with expression quantitative trait loci (eQTL) to predict gene expressions more accurately. An extension of the method PUMICE +, also combines transcriptome-wide association studies (TWAS) results from single- and multi-tissue models.
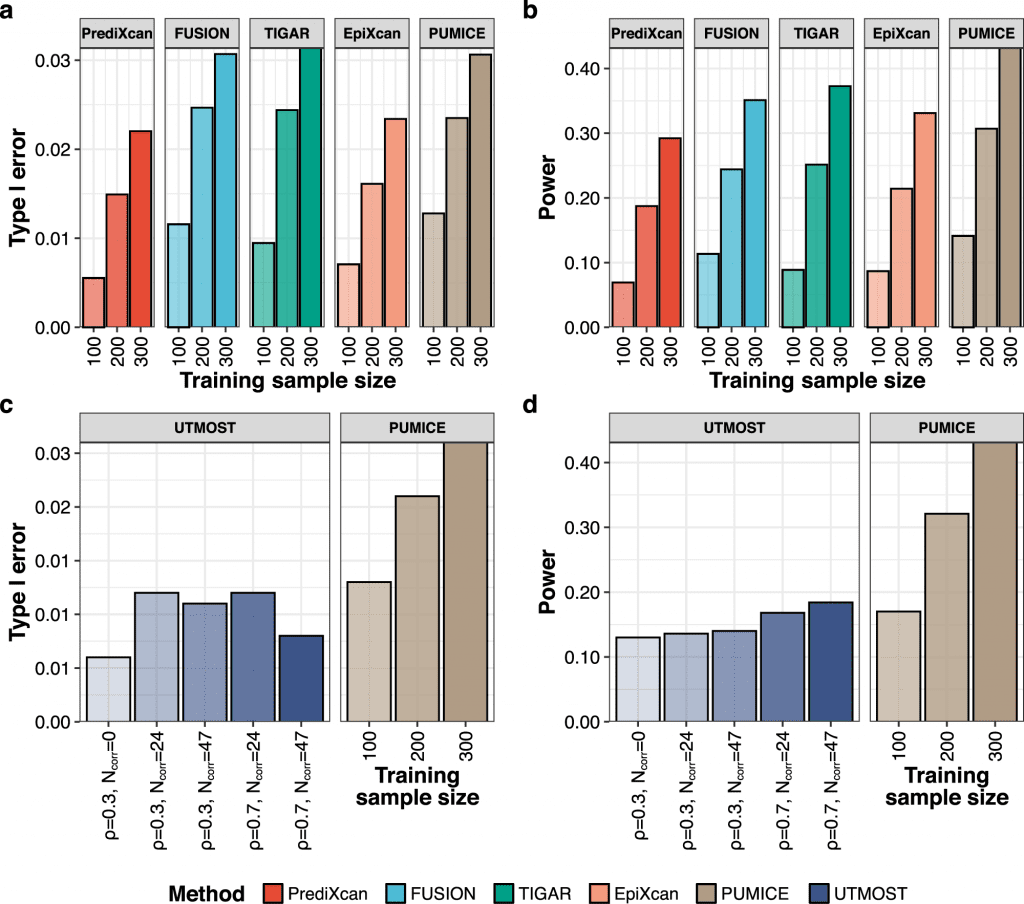
Image Source: Integrating 3D genomic and epigenomic data to enhance target gene discovery and drug repurposing in transcriptome-wide association studies
The TWAS are prominent ways to deal with tests for the association between imputed gene expression levels and traits of interest.
In the given study, the scientists propose an integrative strategy, PUMICE (Prediction Using Models Informed by Chromatin conformations and Epigenomics), to coordinate 3D genomic and epigenomic information with eQTL to all the more precisely predict gene expressions.
PUMICE characterizes and focuses on regions that harbor cis-administrative variations, which beats contending techniques.
The scientists further depict an augmentation to their technique PUMICE+, which mutually consolidates TWAS results from single-and multi-tissue models.
Across 79 characteristics, PUMICE+ distinguishes 22% more independent novel genes and increases median chi-square insight values at known loci by 35%, contrasted with the second-best strategy, as well as accomplishes the narrowest credible interval size. In conclusion, the scientists performed computational drug repurposing and affirmed that PUMICE+ outflanks other TWAS strategies.
The Necessity of Integration of Multi-Omics Data
With the rapid decrease in high-throughput genotyping costs, GWAS has been generally used to look for genetic risk factors inclining people toward complex diseases or traits.
These investigations to date have recognized a huge number of single nucleotide polymorphisms (SNPs) related to complex phenotypes, yet a considerable lot of these SNPs are non-coding, which are trying to decipher their biological functions.
Incorporating multi-omics information is certainly important for clarifying the biological functions and clinical results of non-coding variants.
TWAS and its Methods
As of late, a novel methodology named transcriptome-wide association studies (TWAS) has been proposed and acquired notoriety as an elective method for performing gene-based association analyses.
Momentarily, TWAS determines the gene expression prediction models from datasets with matched genotypes and gene expression information (e.g., Genotype-Tissue Expression project (GTEx), Depression Gene Network (DGN), Common Mind Consortium (CMC), Genetic European Variation in Disease (GEUVADIS)).
By the utilization of these models, gene expression levels can be credited in a GWAS dataset and then tested for associations with complex traits to distinguish genetically-regulated target genes.
Until this point, various TWAS techniques have been created, with every strategy utilizing different specifications of regression models for the construction of gene expression prediction models.
TWAS Techniques Integrating 3D Genomic Information
Since the innovation of chromosome conformity capture technology, the 3D genome structure has given significant novel insights of knowledge into cis-regulatory elements.
In particular, chromosomes possess distinct locations inside the nucleus, called chromosome territories, which can be apportioned into chromosomal compartments and further divided into domains and loops.
Critically, it has been shown that genomic segments inside a similar domain have a higher recurrence of interactions with one another than they do with adjoining regions.
The 3D organization of the genome is firmly connected with various pivotal biological functions, including gene expression, DNA repair, and DNA replication regulations. Simultaneously, epigenetic processes, including DNA methylation and histone modification, are remembered to impact gene expression.
Genetic variants that cross over with key annotation tracks (such as enhancers) are bound to be functionally significant and should be focused on improving a gene expression prediction model.
EpiXcan is the main technique that endeavors to consolidate epigenomic information further to develop prediction precision. In particular, EpiXcan produces priors from Roadmap chromHMM annotation and utilizes quadratic Bezier functions to rescale SNP priors to penalty factors utilized in a weighted elastic net regression.
In any case, it is computationally costly to get a rescaling condition, and in the study, the researchers just utilized eight representative genes to pick an ideal Bezier function. As far as anyone is concerned, none of the TWAS techniques to date coordinates 3D genomic information in demonstrating gene expression levels.
PUMICE and PUMICE+
In this study, the scientists feature the strength of the utilization of both 3D genomic and epigenomic information to all the more precise model gene expression and all the more capably lead TWAS, through another strategy which we call PUMICE (Prediction Using Models Informed by Chromatin conformations and Epigenomics).
PUMICE beats existing single-tissue and multi-tissue strategies as far as the predictive preciseness of gene expression levels, the power for recognizing related genes, and the fine-mapping resolutions in the examinations of 79 complex traits/diseases.
PUMICE can be additionally improved by joining with the multi-tissue TWAS strategy UTMOST (which we call PUMICE+), as multi-tissue techniques offer corresponding data.
The scientists additionally performed computational drug repurposing by utilizing their TWAS results and identified clinically-significant small molecule compounds that might be repurposed for treating immune-related traits, COVID-19-related results, and other relevant disease results.
The PUMICE Framework: Future Scope
The PUMICE framework exhibited the power of an integrative technique in gene discovery, fine-mapping, and clinical interpretation. A few future bearings in information generation and strategy improvement could additionally upgrade PUMICE and future techniques thereof.
To start with, PUMICE would profit from greater resolution 3D genomic information from matched tissues and cell types. Tissue/cell-type-explicit 3D genomic information is still restricted right now; consequently, the researchers involved 3D genomic information from proxy tissues in the PUMICE model.
It relied on the understanding that tissues with similar global gene expression profiles have similar 3D genome organizational structures. This assumption was held in high regard by perceiving that domains are steady across various cell types and that 3D genome structures are associated with gene expression profiles.
Regardless, the scientists accepted that 3D genomic information would turn out to be all the more broadly accessible in the future as the Hi-C strategy requires less starting material to perform experiments.
What’s more, the researchers likewise envisioned that incorporating richer 3D genomic and epigenomic information from matched tissues would help models that mutually consider cis-and trans-eQTLs.
Second, it is conceivable in the future to consolidate transcription factors (TFs) into the PUMICE model. Enhancers and their related TFs play the main role in the commencement of gene expression.
While the researchers’ technique integrates the data of enhancer sites, enhancers simply go about as operational platforms to recruit TFs. In this way, further improvement can be anticipated by integrating TF chromatin immunoprecipitation followed by sequencing information or TF binding models.
Third, the PUMICE system would profit from tests of non-European ancestral lines for fine-mapping causal genes.
Through integrative methodologies, PUMICE+ offers the narrowest credible sets among all techniques. However, complex LD patterns can, in any case, be a significant obstacle to limiting the credible sets to single-gene resolution.
As LD patterns are varied across lineages, incorporating non-European samples and the development of relevant strategies would permit further refinement of causal genes and work with downstream functional follow-up.
Ultimately, current TWAS techniques model gene expression levels with straight models, overlooking the chance of epistasis where multiple regulatory variants mutually influence gene expression (such as enhancer-promoter interactions).
Thus, it is of interest in the future to show gene expression levels with machine learning techniques that can deal with non-linear relationships (such as random forest and boosting) as well as consolidate useful genomic information.
The Endpoint
In the given study, the scientists present a technique that coordinates genomic, transcriptomic, epigenomic, and 3D genomic information for the identification of target genes related to complex traits/diseases.
By the integration of functionally relevant epigenomic and 3D genomic information, they noticed a critical improvement in gene expression prediction performance contrasted with other past TWAS strategies.
They attributed the improvement to combining earlier information, which guided the selection of biologically informative models. Improved transcriptome imputation performance prompts expanded ability to identify more trait-associated genes and works on downstream fine-mapping and drug repurposing investigation.
All in all, PUMICE is an integrative, adaptable, and flexible system for performing gene-based association analysis. PUMICE is based on the abundance of multi-omics information that is openly accessible, permitting the framework to recognize prediction models with higher imputation accuracy.
The scientists envisioned that the PUMICE software pipeline and pre-computed models could help various forthcoming in-silico functional follow-up studies and assist with recovering valuable data from the abundance of GWAS information.
Article Source: Khunsriraksakul, C., McGuire, D., Sauteraud, R. et al. Integrating 3D genomic and epigenomic data to enhance target gene discovery and drug repurposing in transcriptome-wide association studies. Nat Commun 13, 3258 (2022). https://doi.org/10.1038/s41467-022-30956-7
Learn More:
Top Bioinformatics Books ↗
Learn more to get deeper insights into the field of bioinformatics.
Top Free Online Bioinformatics Courses ↗
Freely available courses to learn each and every aspect of bioinformatics.
Latest Bioinformatics Breakthroughs ↗
Stay updated with the latest discoveries in the field of bioinformatics.
Tanveen Kaur is a consulting intern at CBIRT, currently, she's pursuing post-graduation in Biotechnology from Shoolini University, Himachal Pradesh. Her interests primarily lay in researching the new advancements in the world of biotechnology and bioinformatics, having a dream of being one of the best researchers.