Scientists from Johns Hopkins University lay out the possibility of energy-efficient biocomputing using brain organoids (3D brain cell cultures) that surpass in-silico computing capabilities. The recent advances in how human stem cell-derived brain organoids replicate crucial cellular, and molecular aspects of learning and memory have led to the coining of the term Organoid Intelligence (OI). The development in brain organoid research promises to address in-vitro cognition or, as the authors say, intelligence-in-a-dish. The authors anticipate OI-based systems to yield faster decision-making capabilities, uninterrupted learning during tasks, and greater data and energy efficiency as compared to Artificial Intelligence-based systems. The research was recently published in Frontiers in Science.
Biological learning or Machine learning: which is more efficient?
Biological as well as machine learning involves building internal representations of the world to enhance task performance. But the implementation mechanisms involved in the two learning paradigms result in drastic efficiency differences.
Firstly, biological learning requires much less power to perform computational tasks as compared to machine learning. For example, a larval zebrafish uses only 0.1 microwatts of power to navigate the world and successfully hunt prey and avoid predators. While for an adult human being, the consumption is 100 watts, twenty percent of which is consumed by the human brain itself. On the other hand, clusters implementing state-of-the-art machine learning models require around 1 million watts.
Secondly, biological learning involves fewer observations for learning problem-solving. As an example, humans require as few as ten training samples to learn a “same vs different “task. The same is achieved by honeybees using approximately 100 training samples. However, as shown in the literature, machines have failed to achieve this using a million samples and also ten million samples in two different trials and instances.
The United States houses the world’s most powerful supercomputer with a computing ability of 1015 floating point operations per second. For this, it consumes 21 megawatts. The human brain, on the other hand, performs approximately the same number of operations consuming only 20 watts. This difference is indeed remarkable and alludes to the energy efficiency of biological learning over machine learning.
These observations have led to greater interest in biological and brain-directed computing as an alternative to in-silico computing. While biocomputing promises advancing computing speed, processing power, and storage capabilities, all with lower energy requirements, realizing the biocomputing potential has been posed with challenges, and the research still remains in a state of infancy. Thus the need for a consortium and the establishment of OI as an emerging field of research to forward brain-directed OI computing.
The blueprint for the development and implementation of the OI program
The authors envision systematically training organoids using biofeedback with complex sensory inputs. The architecture aims to interface the brain organoids with computers, sensors, and machine interfaces for facilitating supervised and unsupervised learning. While Artificial Intelligence aims to make computers function more like brains, OI research will aim to explore how a three-dimensional (3D) brain cell culture can be more computer-like.
What are brain organoids?
Brain organoids are three-dimensional (3D) brain cell cultures. They are shown to recapitulate organ histoarchitecture and functionality much closer than two-dimensional (2D) cell cultures. They contain myelinated axons and exhibit spontaneous electrophysiological activity as well as complex oscillatory behavior. Myelination increases electrical conductivity 100-fold, thereby boosting computing performance. They also exhibit high cell density and layered patterning. The brain organoids can be enriched with various cell types, such as microglia, astrocytes as well as oligodendrocytes, which are all involved in learning and memory formation.
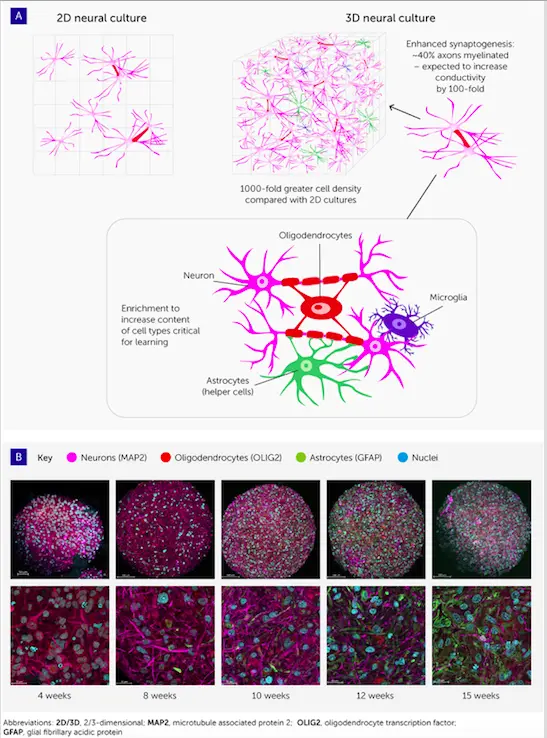
Image source: https://www.frontiersin.org/journals/science/articles/10.3389/fsci.2023.1017235
The following figure illustrates the blueprint for the OI-based system architecture.
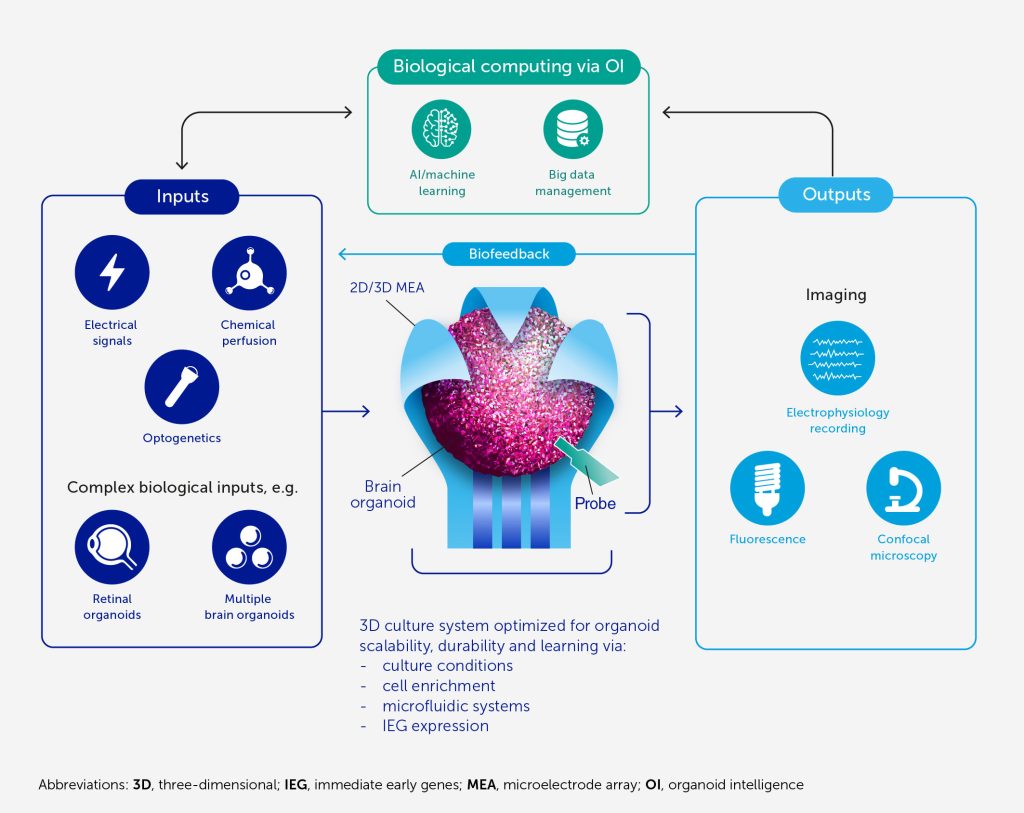
Image source: https://www.frontiersin.org/journals/science/articles/10.3389/fsci.2023.1017235
The architectural blueprint:
- At the center is the 3D brain culture, the organoid that performs the computation.
- The learning potential of the organoid is enhanced by culture conditions as well as by enriching the culture with other cell types as well as genes involved in learning and memory formation.
- The durability, scalability, as well as viability of the organoid is maintained by integrated microfluidic systems.
- Inputs range from electrical and chemical to synthetic signals from machine sensors as well as natural signals from connected organoids such as retinal organoids.
The authors anticipate high-resolution output measurements by electrophysiological recordings using specially designed 2D or 3D micro-electrode arrays (MEAs). Output measurements are also possible via implantable probes imaging organoid structural and functional properties. These outputs can then act as biofeedback to promote organoid learning. It is noteworthy that both artificial intelligence and machine learning are expected to be heavily used in encoding and decoding signals as well as for developing hybrid computing solutions.
OI could advance medical research and innovation
In addition to the usage of brain organoids as units of enhanced computing and learning, the brain organoid intelligence system can be of paramount importance in medical research and innovations. OI can bring forth inter-individual neurodevelopmental as well as neurodegenerative differences between stem cell donors, thereby advancing a better understanding of disease mechanisms in diseases like Alzheimer’s and other forms of dementia. This could aid in future innovations in therapeutics for these life-threatening diseases.
Conclusion
The authors present a collaborative multidisciplinary program for the establishment of Organoid Intelligence as a genuine form of biocomputing. They aim to revolutionize biological computing, overcoming the shortcomings of in-silico computing in terms of energy efficiency as well as faster decision-making. With the intelligence-in-a-dish, the authors aim for OI-based biocomputing systems to allow continuous learning during tasks and better energy and data efficiency. OI will further aid in elucidating the biological basis of human cognition as well as learning and memory formation, thereby highlighting disease-causing mechanisms. This will lead to further innovations in terms of therapeutics. Thus, OI, the new biocomputing alternative to Artificial Intelligence, is set to revolutionize computing and neuroscience research.
Article Source: Reference Paper
Learn More:
Banhita is a consulting scientific writing intern at CBIRT. She's a mathematician turned bioinformatician. She has gained valuable experience in this field of bioinformatics while working at esteemed institutions like KTH, Sweden, and NCBS, Bangalore. Banhita holds a Master's degree in Mathematics from the prestigious IIT Madras, as well as the University of Western Ontario in Canada. She's is deeply passionate about scientific writing, making her an invaluable asset to any research team.