Understanding the intricate physical interactions between a molecule and its surroundings is necessary to generate unique active molecules for a particular protein, which is a very difficult problem for generative models. In a recent study, scientists from Insilico Medicine and their collaborators introduced a unique generative model called BindGPT that builds 3D molecules inside protein binding sites using an intuitive yet effective method. By producing molecular graphs and conformations simultaneously, the BindGPT model does away with the requirement for a separate reconstruction process. It uses external simulation tools to fine-tune with reinforcement learning after pretraining on a large-scale dataset. The model can be used as a pocket-conditioned 3D molecule generator, a conformer generator conditioned on the molecular graph, and a 3D molecular generative model in three dimensions. Regarding the domain of generation, it does not assume representational equivariance. This straightforward method is less expensive to sample and performs better than the best-specialized diffusion models, language models, and graph neural networks at the moment.
Introduction
The effective exploration of the large space of drug-like compounds, estimated to be approximately 1060 in size, requires the application of molecular models in drug creation. A key approach is computer-aided drug design (CADD), which explores these compounds through physical simulations and software screenings. This investigation has been transformed by developments in deep learning, such as neural generative models trained on large compound datasets. The creation of new, drug-like compounds has been made possible by the textual representation of chemical structures using SELFIES and SMILES.
According to recent research, deep generative models can produce 3D molecular compounds, including protein pockets and ligand subfragment conditions. In order to produce physically feasible molecular structures, diffusion models such as EDM and DiffDock begin the production process with an arbitrary spatial distribution of atoms and refine their placements. Building on the current molecular framework, autoregressive models such as Pocket2Mol successively anticipate the type and location of each subsequent atom. Additionally, language models have proven to be adept in managing spatial representations of protein and molecular structures in formats such as PDB, XYZ, and CIF. Still, the majority of spatial molecular generators only consider atom locations and kinds, depending on additional resources such as OpenBabel to recreate bonds.
Understanding BindGPT
Researchers have developed a unique framework to express 3D molecular data using textual tokens. This data-driven methodology, grounded in the GPT paradigm, improves the scalability of inference and model training. A potent causal language model that can navigate the intricate space of three-dimensional molecules is fostered by the model pretraining paradigm. Applications that have been successful include:
- Building compounds with targeted binding affinities to particular proteins.
- Synthesizing 3D conformations.
- Learning the distribution of molecules.
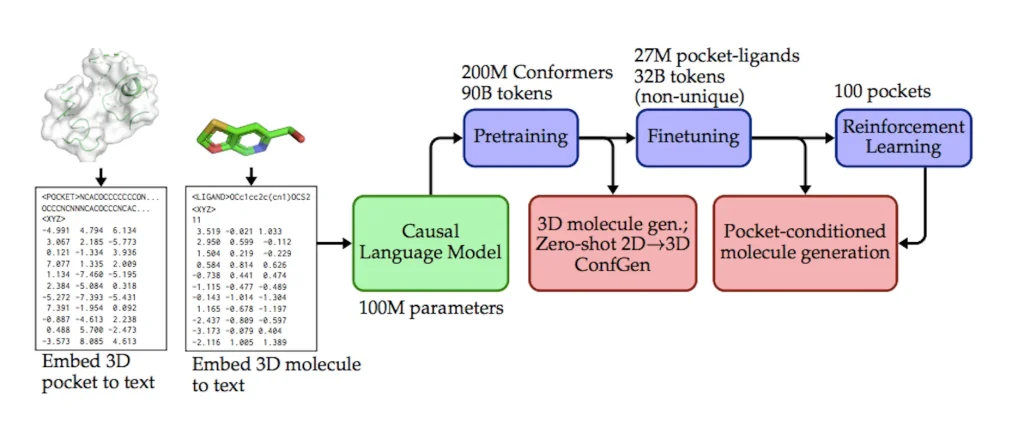
Key Features of BindGPT
- BindGPT, a language model, manages spatial molecular structures in text format by describing molecular graphs and atom locations using structural SMILES and spatial XYZ formats, hence removing the need for graph reconstruction tools.
- Researchers have proposed a scalable pretraining-finetuning approach that addresses many 3D molecular production tasks in a single paradigm for 3D drug discovery.
- Researchers demonstrate how BindGPT, which has the ability to use molecular graphs or protein pocket descriptions as prompts, can produce realistic and precise 3D molecular structures both zero-shot and after fine-tuning. With a speedup of increase to 100x, the method provides generation quality that is equivalent to leading approaches.
- Lastly, using external feedback from docking software, the researchers show how well the Reinforcement Learning (RL) framework optimizes BindGPT. Researchers demonstrate that as a result of the RL fine-tuning, the resulting model can locate structures with high binding scores for any given protein.
Advantages of BindGPT
Explicit hydrogen modeling is a difficult task that few baseline approaches can accomplish. However, the pre-trained BindGPT model performs better than the XYZ-TF baseline. The GEOM-DRUGS dataset demonstrates the state-of-the-art performance of BindGPT, the first model able to explicitly represent hydrogen at a large scale. BindGPT performs worse than MolDiff; however, it may be because of its narrower vocabulary and more common atoms. The Platinum dataset contrasts BindGPT and Uni-Mol-BERT, which have both been pre-trained and refined using the same data, with Torsional Diffusion (TD). With the aid of the RDKit tool, BindGPT can equal TD’s performance, showcasing its generalizability and capacity to do various tasks at this level of output.
Conclusion
BindGPT is a scalable framework for training language models that can produce text with 3D molecules, as presented in this paper. The scalable system, free from inductive biases, matches or exceeds baselines to tackle various 3D molecular generative challenges. In contrast to baselines with substantial inductive biases, the approach is data-driven and universal. Of particular relevance is the pocket-based molecule generation task, in which the model surpasses all baselines. This study shows that 3D drug discovery can effectively leverage the large-scale pretraining paradigm from natural language processing.
Article Source: Reference Paper
Important Note: arXiv releases preprints that have not yet undergone peer review. As a result, it is important to note that these papers should not be considered conclusive evidence, nor should they be used to direct clinical practice or influence health-related behavior. It is also important to understand that the information presented in these papers is not yet considered established or confirmed.
Follow Us!
Learn More: