Phylogenetic approaches can be used to infer the order in which mutations arise during cancer progression by analyzing patterns of mutation occurrence in a population of cells. By identifying clades of related cells, it is possible to trace the evolutionary history of each cell and determine which mutations occurred first. This information can then be used to inform cancer treatment decisions and further our understanding of the disease.
The evolutionary processes that might affect disparities in clinical outcomes are receiving more attention, despite the fact that the importance of evolution in the development of cancer is generally acknowledged. Somatic evolution is the process through which cancer develops. USA-based researchers at Ohio University studied that it matters in what temporal order somatic mutations develop throughout the development of cancer.
Sequencing information from a single biopsy gives a snapshot of this process that can show when distinct genomic abnormalities first appeared and how the effect of mutational processes changed over time. An exceptional prospect to investigate the impact of mutation order on cancer development and therapeutic outcomes is provided by single-cell sequencing (SCS).
The majority of cancer phylogenetics research makes use of bulk high-throughput sequencing data, however, these signals only represent a population of sequenced cells as a whole rather than specific properties of individual cells. For instance, some approaches compare the frequency of mutations spanning bulk sequencing data from various tumor samples and individuals to estimate mutation order. It is challenging to assess the variance in mutations across various tumor cell types only from bulk sequencing data. Because technology makes it possible to sequence individual cells, single-cell sequencing (SCS) is concerning because it produces high-resolution data that may be used to interpret the mutational history of cancer.
The approach integrates models on two different levels by simulating both the technical faults that might occur during the single-cell sequencing data-gathering procedure as well as the evolutionary process of somatic mutation within the tumor. This method significantly dominates existing approaches for determining mutation order through assessments of simulations covering a wide range of actual circumstances. Most significantly, this strategy offers a special way to identify and measure the degree of uncertainty associated with the predicted mutation order along a particular phylogeny. Data acquisition from colorectal and prostate cancer patients demonstrated this technique, which supports previously published mutation orders. This research is a significant step in measuring the uncertainty of anticipated mutation order in cancer patients and producing relevant mutation order predictions with high accuracy. A new technique for determining the order of somatic mutation emergence inside a single tumor using noisy single-cell sequencing data. This study has the potential to provide new insights into the evolutionary pathways of cancer.
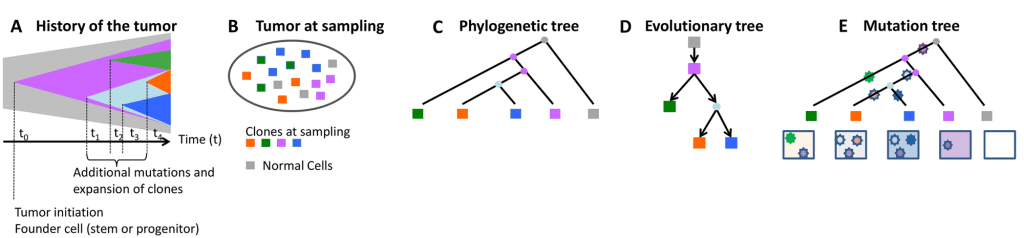
Image source – https://doi.org/10.1371/journal.pcbi.1010560
The advent of computational tools centered on a phylogenetic paradigm for use in researching the evolution of cancer offers a great deal of understanding of the mechanisms that cause ITH, particularly the function of the temporal order of mutations in the development of cancer. For instance, Ortmann et al. have demonstrated differences in clinical characteristics and treatment response for patients with different mutation orders, denoting that the inference of the order in which mutations arise within a particular tumor has direct implications in clinical oncology, for both diagnostic applications in determining the extent of ITH and targeted therapy. SCS data offer a rare chance to predict mutation order with the highest level of detail. However, the whole-genome amplification procedure exposes such data to significant technical flaws.
Walking Through the Features of Mutational Order Inference from Single-cell Sequencing Data
MO, a novel Bayesian method for rebuilding the sequencing of mutational events from the incomplete mutation profiles of single cells, to assess such data. Relying on a phylogeny of cell lineages that enables modeling of the mistakes at each tip, MO is made to deduce the chronological order of a group of interesting mutations. When single-cell data sets are vulnerable to technological noise, such as ADO, false positive errors, and missing data, MO can infer the mutation order that best matches the data. The application of MO to clonal trees and models that take into account reported data errors for several cells in a tip as opposed to a single cell might be expanded.
Furthermore, MO may be easily changed to account for the faster mutation rates typical of late-stage malignancies, to permit back or parallel mutation, or to permit heterogeneity in mutation rates among locations. The flexibility of MO also allows the creation of an evolutionary model that takes into account the consequences of point mutations in single-cell data sets. Even with a limited number of single cells, MO functions properly and is resilient to variations in error rates. The simulation findings show that MO gives a more precise estimate as the number of relevant single cells grows.
The Precision of Mutation Order (MO) Prediction
By simulating both the tumor’s internal evolutionary process and the faults that can occur during the gathering of SCS data, MO improves the precision of mutation order prediction. The unique strategy for assessing uncertainty in the estimated order along the phylogeny is another significant distinction between MO and other techniques like SCITE and SiFit. The calculation of the posterior probability distribution across orders is possible using SCITE’s available options. SiFit, on the other hand, does not provide this information when reporting the mutation order. MO, on the other hand, captures a different source of uncertainty than SCITE and SiFit since it utilizes a predictive model to deduce mutation positions along a tree and is, therefore, able to offer an estimate of the uncertainty in the detected locations conditional on the tumor phylogeny.
A thorough series of simulations that consider several facets of contemporary SCS data sets by looking at a wide variety of error probabilities, fractions of missing data, branch lengths, and numbers of cells in each tree demonstrates that MO operates properly. The simulation experiments also show that MO performs better than cutting-edge techniques, particularly when the phylogeny contains a lot of cells. MO can accurately rebuild the mutation order that has been shown to be crucial in the course of cancer. MO is also utilized to reconstruct the mutation order for data from patients with prostate cancer and colorectal cancer. The technological hiccups that occur during whole-genome amplification are resilient to MO. The ordering of cancer-related mutations may be understood by MO, as well as the degree of confidence in the order. MO integrates across the uncertainty in these parameters rather than offering estimates of transition rates and error probability.
MO is prepared to analyze the derived large-scale data sets to draw meaningful conclusions about the mutation order during tumor progression for specific patients as SCS data collection technology advances, making it possible to analyze hundreds of cells in parallel at lower cost and higher throughput. As a result, MO represents a significant advancement in our knowledge of the significance of mutation order in the progression of cancer, and as such, it may have substantial translational implications for enhancing cancer detection, care, and individualized therapy. Future studies can investigate the causes of clinical outcomes given distinct mutation orders in order to create innovative targeted therapies after the links between the projected mutation order and clinical implications are proven. This will enable medical professionals to base treatment choices on the mutational profiles of individual patients.
Significance of Mutation Order Inference
MO may be used to analyze single-cell mutation profiles from various domains, including immunology, neuroscience, and microbiology, even though the current work focuses on cancer. These applications will offer fresh perspectives on how to comprehend cancer and other human illnesses.
Article Source: Reference Paper
Learn More:
Top Bioinformatics Books ↗
Learn more to get deeper insights into the field of bioinformatics.
Top Free Online Bioinformatics Courses ↗
Freely available courses to learn each and every aspect of bioinformatics.
Latest Bioinformatics Breakthroughs ↗
Stay updated with the latest discoveries in the field of bioinformatics.
Riya Vishwakarma is a consulting content writing intern at CBIRT. Currently, she's pursuing a Master's in Biotechnology from Govt. VYT PG Autonomous College, Chhattisgarh. With a steep inclination towards research, she is techno-savvy with a sound interest in content writing and digital handling. She has dedicated three years as a writer and gained experience in literary writing as well as counting many such years ahead.