Deep learning extensively affects studying genetics and the breakthrough research associated with them. The study focuses on understanding the building of complex models rather than complex datasets using model interpretations in Explainable Artificial Intelligence (xAI). This helps in understanding the utility of complex models to decipher biological datasets.
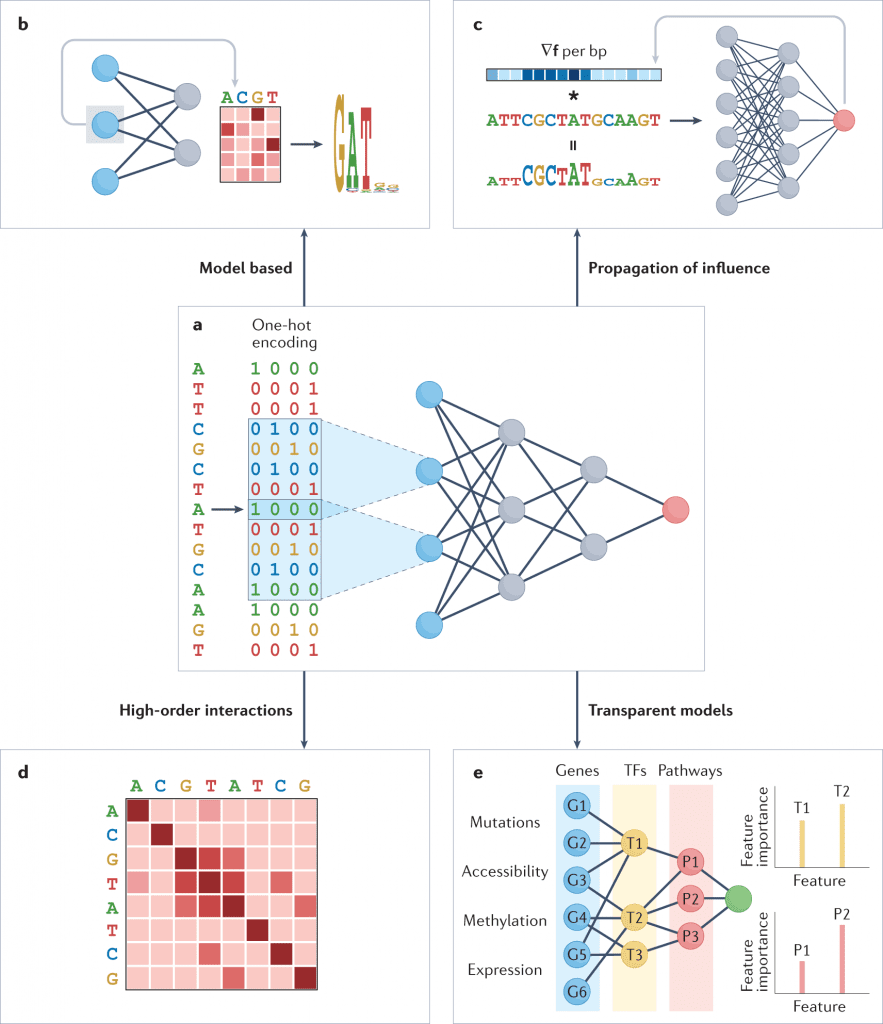
Image Source: https://doi.org/10.1038/s41576-022-00532-2
Artificial intelligence-based deep learning models are known to produce predictions for deciphering the human genome and other biological datasets. These deep learning models can determine the gene transcription from single cell type based on regulatory factors and quantification of chromatin structure to determine the interactions for annotations and single-cell data analysis.
Researchers from the University of British Columbia, Canada, have reviewed these deep learning models to address the black box due to its complexity. The bigger question addressed by reviewers is how these models develop such predictions. The question has seemingly moved from understanding the complexity of datasets to understanding the complex model. The review systematically overviews the key interpretation approaches and strengthens the use of AI among life science researchers.
Reviewers have employed post-hoc interpretation—an important class of explainable AI, which operates on trained models and identifies relevant combinations of scaler inputs, also called features, and the importance of each feature. The post-hoc interpretation method has two types: local interpretation and global interpretation. The local interpretation aims to identify relevant features that affect the prediction of the model based on a single input, whereas global interpretation determines these features affecting the prediction model based on combinations across all the features.
To understand the relativity of deep learning models in regulatory genomics and conceptual approaches to explainable artificial intelligence, four approaches for interpretation were categorized:
1) Model-based interpretation.
2) Mathematical propagation of influence.
3) Identification of interaction between features.
4) Use of prior knowledge for transparent models.
The following approaches are believed to provide an intuitive understanding of how deep neural networks work on high throughput biomedical datasets and deduce fundamental assumptions and limitations of each model. Briefing about deep learning methods in regulatory genomics provides the architecture to model construction. Reviewers have employed the explanation using sequence-to-activity models-based neural networks and why the interpretation of DNN (deep neural network) is challenging. The two challenges are, first, the lack of a global combinatorial strategy for model optimization. Secondly, inability to systematically evaluate the interpretation changes due to the absence of standard datasets.
Model-based Interpretation
This approach examines the individual components associated with the model and its performance of predictions. Since this approach is effective in smaller datasets, the large datasets of DNN are difficult to determine. The review team has highlighted the use of partial model-based interpretation for DNN, such as determining the first layer of neurons representing transcription factor motifs. The two approaches are direct and attention mechanism, wherein the direct approach directly determines the hidden neurons to determine important features, whereas the attention mechanism measures the applicability based on each input feature through a set of learned prioritization in the trained dataset of DNN. Other approaches include Interpreting first-layer convolutional nodes, Attention mechanism weights for visualizing feature importance.
Mathematical Propagation of Influence
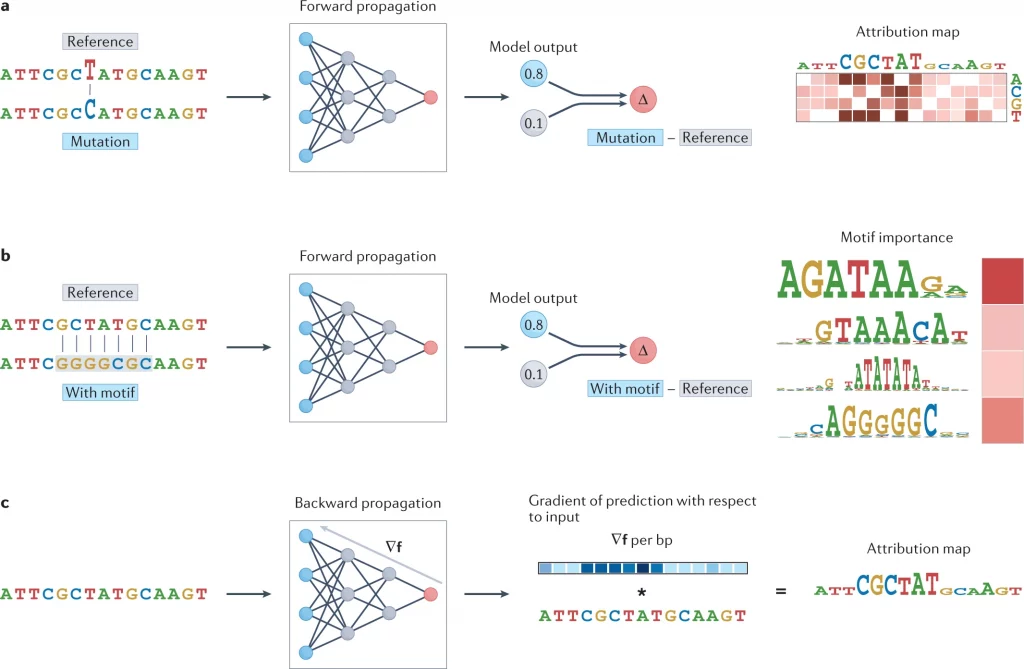
Image source: https://doi.org/10.1038/s41576-022-00532-2.
In contrast to earlier interpretation methods, which explore the trained neural networks, mathematical propagation operates on input examples by propagating unsettled data through the model and determining the effect of prediction based on it. Propagation-based attribution methods are bifurcated into two groups: forward propagation of influence and backward propagation of influence.
The idea of forwarding propagation of influence is simply understood as flipping elements associated with nucleotides of a sequence to determine the importance of features for trained models. The entire strategy is also termed in silico mutagenesis (ISM). Whereas the idea of backward propagation of influence addresses the limitations of forward, approximates ISM by evaluating the derivative of model F for the given input sequence to determine changes in the model’s prediction.
Identifying Interactions Between Features
The earlier approaches were used to analyze the effects based on individual features, however, using this approach, the reviewers have highlighted methods to produce local interpretations to explain the interactions within the features based on neural networks. Both model-based identification systems (models using self-attention) and mathematical propagation (targeted analysis for the specific motif) systems use models to predict the interactions.
Using Prior Knowledge for Transparent Models
Reviewers compared the input nodes to hidden nodes within the deeper layers of DNN since it is more challenging due to complexity and non-linearity. The transparent model construction is based on these hidden nodes, which can determine the composition helpful for human exploration. The model’s transparency helps assist in understanding the mutations and disruptions between the genes that transcend to understanding the regulatory genomics for novel discovery.
Final Thoughts
The review focused on understanding the sequence-to-activity models and has described explainable artificial intelligence (xAI) and methods associated to determine applications of deep learning on various aspects of biological databases. The applicability of the approach helps researchers to use prediction models by identifying hidden nodes and training data for better predictions for gene therapy, identifying novel biomarkers and rare disease types.
Article Source: Reference Paper
Learn More:
Top Bioinformatics Books ↗
Learn more to get deeper insights into the field of bioinformatics.
Top Free Online Bioinformatics Courses ↗
Freely available courses to learn each and every aspect of bioinformatics.
Latest Bioinformatics Breakthroughs ↗
Stay updated with the latest discoveries in the field of bioinformatics.
Mahi Sharma is a consulting Content Writing Intern at the Centre of Bioinformatics Research and Technology (CBIRT). She is a postgraduate with a Master's in Immunology degree from Amity University, Noida. She has interned at CSIR-Institute of Microbial Technology, working on human and environmental microbiomes. She actively promotes research on microcosmos and science communication through her personal blog.