Researchers from the Helmholtz Centre Munich, Germany, provide CellRank, a method for single-cell fate mapping in a variety of circumstances where the direction is unclear, such as regeneration, reprogramming, and disease.
During numerous biological processes, cells go through state transitions in a highly asynchronous manner. Single-cell RNA sequencing (scRNA-seq) successfully captures the heterogeneity that comes from these processes, but it misses lineage links because each cell can be measured only once. To address this issue, scRNA-seq can be used in combination with lineage tracing methods, which use heritable barcodes to track clonal evolution over long time scales, or metabolic labeling methods, which use the ratio of nascent to mature RNA molecules to link observed gene expression profiles over short time windows. Both methodologies, however, are primarily limited to in vitro applications, so it encourages the development of computational algorithms to reconstruct pseudotime trajectories that take advantage of the fact that developmentally related cells have comparable gene expression profiles.
Here the researchers present a single-cell fate mapping method, CellRank, that learns directed, probabilistic state-change trajectories under normal or perturbed conditions by combining the robustness of similarity-based trajectory inference with directional information from RNA velocity. CellRank computes fate probabilities that account for the stochastic nature of cellular fate decisions as well as uncertainty in velocity estimates by automatically inferring the initial, intermediate, and terminal populations of a scRNA-seq dataset.
CellRank clubs gene expression similarity with RNA velocity to reliably predict directed cellular trajectories across experimental platforms in development, reprogramming, and regeneration. The algorithm takes a count matrix from scRNA-seq and a related RNA velocity matrix as input. All pseudotime techniques that properly capture trajectories are based on the notion that cell states change in short increments with multiple transitional populations. The same assumption is used by CellRank to model state transitions using a Markov chain. To visualize gene expression programs executed by cells along trajectories leading to terminal states, the researchers combine fate probability estimations with a pseudotemporal ordering.
CellRank improves the ability to find putative trajectory-specific regulators by connecting gene expression with fate probabilities. Gene expression cascades were visualized specific to their cellular trajectory while accounting for the ongoing nature of cellular fate commitment by sorting putative regulators according to their peak in pseudotime.
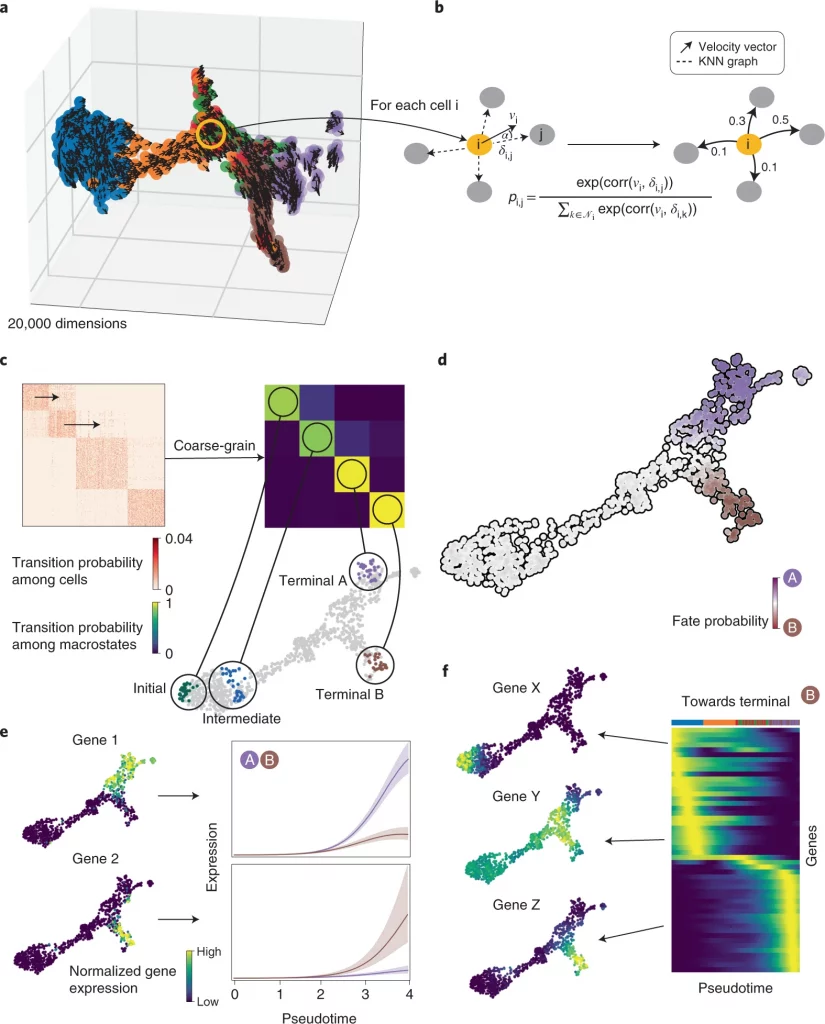
Image Source: CellRank for directed single-cell fate mapping.
Next, the researchers used CellRank to analyse a scRNA-seq dataset of E15.5 mouse pancreas development. The main developmental trends were recapitulated using a UMAP representation with original cluster annotations and scVelo-projected velocities; cells progress from an initial cluster of endocrine progenitors (EPs) expressing low levels of the transcription factor neurogenin 3 (Neurog3 or Ngn3) to alpha, beta, epsilon, and delta cell fates. Using eigenvalue gap analysis, they coarse-grained CellRank’s directed transition matrix into 12 macrostates, revealing a block-like structure in the transition matrix. All developmental stages in this dataset were represented by macrostates, which were annotated based on their overlap with the underlying gene expression clusters. These included a starting state, intermediate states, and terminal hormone-producing alpha, beta, epsilon, and delta cell states.
CellRank beat prior methods by accurately recovering initial and terminal states, fate potentials, and gene expression patterns on 100,000 cells, as well as efficiently computing terminal states and fate potentials. According to the coarse-grained transition matrix, the alpha, beta, and epsilon states are the three most stable states. The ductal and endocrine terminal states were automatically identified using coarse-grained transition probabilities among five macrostates, and the likelihood of ductal and endocrine lineage destiny aligned well with known lineage markers.
Delta cells show how CellRank’s global approach overcomes RNA velocity restrictions. The dynamics of delta cell growth are not captured by RNA velocity; however, CellRank can recover them well because it constrains velocities to the phenotypic manifold through the KNN graph, incorporating cell-cell similarity, and models long-range trends.
In a perturbation scenario, CellRank was used to analyze 48,515 mouse embryonic fibroblasts (MEFs) reprogramming into induced endoderm progenitors (iEPs) throughout six time periods. Only about 1% of cells are projected to reprogram effectively, while the rest will enter a ‘dead-end’ state. This dataset comprises CellTagging lineage tracing data that can be used to rebuild clonal relationships among cells, providing ground truth on how early cells ultimately ended up.
scVelo was used to calculate velocities and projected them onto the original t-SNE embedding. Both a dead-end and a rare successful state were included in CellRank’s macrostates. By calculating the likelihood of these states and comparing them with lineage-tracing derived labels, it was found that fate probabilities were greatly predictive of reprogramming outcomes and that predictive accuracy reduced for earlier days in the course of time, as expected.
The researchers compared CellRank to similarity-based approaches that offer cell-fate probabilities (Palantir, STEMNET, and FateID) and a velocity-based method that computes initial/terminal states (velocyto) on pancreas data to assess the impact of including velocity information. CellRank was the only algorithm that accurately recognized both the initial and final states.
The researchers also sent CellRank’s terminal states to all approaches and examined cell-fate probabilities, finding that only CellRank and Palantir accurately identified beta as the dominating fate.
To show CellRank’s ability in the context of regeneration, we used it to study mouse lung regeneration following acute injury. The scRNA-seq dataset included 24,882 lung airway and alveolar epithelial cells sequenced with Drop-seq, a lower resolution single-cell technology, at 13-time points spanning days 2–15 after bleomycin damage.
Here, CellRank was applied for unbiased discovery of unexpected regeneration trajectories among airway cells, and they computed scVelo velocities, applied CellRank and found nine macrostates that were used to compute fate probabilities. CellRank analysis predicted a novel dedifferentiation trajectory to basic stem cells, revealing a pathway for creating multipotent stem cells during the resolution phase of the regenerative response to injury.
The researchers anticipated that in the future, an end-to-end framework would be developed that propagates uncertainty from initial counts to end-state assignments and fate probability. They remark that if the velocity vectors are systematically biased, then the computed fate probabilities will reflect these biases, despite uncertainty propagation. CellRank is expected to be beneficial in the future in describing complex trajectories in regeneration, reprogramming, and cancer, where establishing the process’s direction might be difficult.
Story Source: Lange, M., Bergen, V., Klein, M., Setty, M., Reuter, B., Bakhti, M., … & Theis, F. J. (2022). CellRank for directed single-cell fate mapping. Nature methods, 1-12.
Data Availability: https://doi.org/10.1038/s41592-021-01346-6
Learn More:
Top Bioinformatics Books ↗
Learn more to get deeper insights into the field of bioinformatics.
Top Free Online Bioinformatics Courses ↗
Freely available courses to learn each and every aspect of bioinformatics.
Latest Bioinformatics Breakthroughs ↗
Stay updated with the latest discoveries in the field of bioinformatics.
Swati Yadav is a consulting intern at CBIRT. She is currently pursuing her Master's from Delhi Technological University (DTU), Delhi. She is a technology enthusiast and has a keen interest in the latest research on bioinformatics and biotechnology. She is passionate about exploring new advancements in biosciences and their real-life application.