Scientists have developed DeepCycle, a deep learning approach combined with RNA velocity to reveal cell cycle gene regulation. This methodology is particularly appropriate to study the interplay of the cell cycle with pluripotency and cell reprogramming. The method could lead to the discovery of cell-cycle dysregulated mechanisms in malignancies, as well as prospective therapeutic targets.
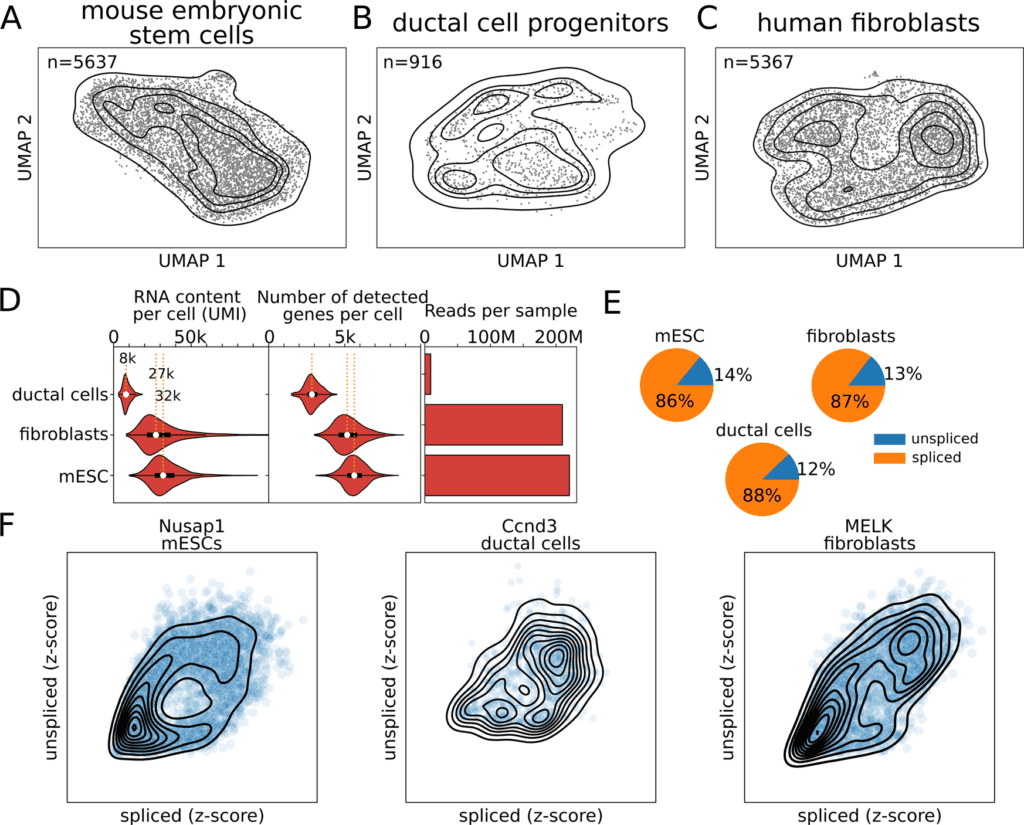
Image Source: Cell cycle gene regulation dynamics revealed by RNA velocity and deep-learning
Even though the cell cycle is a significant process of life, a definite quantitative comprehension of gene regulation dynamics all through the cell cycle is not even close to the end.
Single-cell RNA-sequencing (scRNA-seq) technology gives admittance to these dynamics without externally perturbing the cell. By producing scRNA-seq libraries in various cell systems, the researchers noticed cycling patterns in the unspliced-spliced RNA space of cell cycle-associated genes.
Since existing strategies to examine scRNA-seq are ineffective in quantifying cycling gene dynamics, the scientists proposed a deep learning approach (DeepCycle) to fit these patterns and build a high-resolution map of the whole cell cycle transcriptome.
After the characterization of the cell cycle in embryonic and somatic cells, the scientists distinguished significant transcriptional waves during the G1 stage and methodically studied the phases of the cell cycle. This work would facilitate the investigation of the cell cycle in numerous cellular models and different biological contexts.
An Approach to Generate Gene Expression Series
Cells divide by advancing through exceptionally organized phases in which they grow, synthesize a duplicate of their genetic material, and, at long last, go through mitosis.
On the other hand, cells can quit cycling and reversibly transition into quiet, irreversibly differentiate or become senescent. These cycles require tight dynamic regulation of gene expression. Regardless of tremendous research during the previous many years, a quantitative image of the gene regulation dynamics across the cell cycle is incomplete.
With the coming of single-cell RNA sequencing (scRNA-seq), researchers can now examine intrinsically asynchronous populaces of cells empowering the simultaneous identification and investigation of cells at various cell cycle stages.
In this way, scRNA-seq gives a high-resolution strategy to deal with the studies on the cell cycle without external constraints, for example, synchronization by drugs or engineered fluorescent reporters.
While many endeavors to computationally assign cell cycle stages have been performed, these typically lack generalizability and fail in precisely capturing cell-cycle dynamics. To overcome these issues, the researchers proposed to utilize RNA velocity, a methodology that characterizes the transcriptional state of individual genes given their spliced and unspliced RNA signals in single cells.
In short, RNA velocity depends on how the course of transcription operates. RNA polymerase II begins the transcription of a gene, where it initially produces a pre-mRNA molecule that contains the two exons and introns. Then, at that point, the pre-mRNA molecule goes through splicing to deliver the mature mRNA molecule with no introns.
The assessment of the pre-and mature mRNA levels from unspliced and spliced reads subsequently permits evaluation of the transcriptional changes occurring at the single-gene level.
Then, by the study of the distribution of unspliced and spliced reads across all cells (unspliced-spliced RNA space), it is feasible to estimate if a particular gene is being transcribed or inactivated in any single cell.
This can be all the while applied to all genes in all cells of a populace. With such examination, cell-cycle-associated genes would be supposed to go through activation and deactivation stages inside a single cell cycle, bringing about a circular pattern in the unspliced-spliced RNA space.
Because of the profundity of the scRNA-seq datasets created in this work, such circular patterns in the unspliced-spliced RNA space can be noticed obviously for a subset of genes and can be exploited to stratify cells across the cell cycle naturally.
The challenge is then to allocate, dependably and strongly, a single parameter to every cell that depicts its cell-cycle state combining the data in the cycling patterns.
To achieve this, the scientists designed DeepCycle, a deep learning strategy to credit a ceaseless high-resolution cell cycle trajectory to single cells because of RNA velocity. The methodology applies to various cell types and has self-consistency checks to determine whether the analysis worked appropriately.
DeepCycle permits us to fit the dynamics of gene activation and inactivation in the unspliced-spliced RNA space with negligible assumptions and assign cells to cell cycle stages, creating gene expression series.
Highlighting the Specifics of Cellular Models across Cell Cycles
Different cell types have explicit cell-cycle dynamics. For instance, the cell cycle is profoundly impacted by the degree of stemness, to such an extent that pluripotent and neural stem cells have short G1 stages, while committed cells broaden their G1 stages and present with longer cell cycles.
Through DeepCycle, the scientists not just reiterate these discoveries in mESCs and human fibroblasts yet additionally stretch out the examination to a public dataset of ductal cell progenitors.
This permits them to recommend fundamental regulatory mechanisms included, featuring various genes and transcription factors that are dynamic in the different cell models across the cell cycle.
Confirming Previous Findings
At last, as the vast majority of the cells inside multicellular organisms are not effectively cycling, tight control over cell cycle entry and exit is fundamental, as seen, for instance, in embryonic development, hematopoiesis, adaptive immune response activation, and wound healing.
Be that as it may, in diseases like cancer, cells don’t reliably respond to the typical regulatory cues and signals. It is thus essential to comprehend the cycles that determine cell cycle entry, cell cycle progression, and exit to quiescence.
In this study, the researchers characterized the expanding point where human fibroblasts exit from the cell cycle. This confirms past discoveries and reveals marker genes and transcriptional factors fundamental to the cycle, preparing for the orderly characterization of the G1-G0 progress in other cellular models.
The Endpoint
The scientists created scRNA-seq datasets in mouse embryonic stem cells and human fibroblasts with high sequencing depth. The circular RNA velocity patterns arose plainly in cell-cycle managed genes uncovering the activation/inactivation stages that these genes go through during the cellular cycle.
They developed DeepCycle, a deep learning approach, to take advantage of the RNA velocity patterns and study gene regulation dynamics during the cell cycle. DeepCycle doles out a cell-cycle transcriptional stage for every cell by fitting the RNA velocity patterns.
Moreover, the derived transcriptional stage can be related to cell-cycle stages thanks to known gene markers. In this way, DeepCycle permits the users to determine the cell-cycle progression state of every cell from scRNA-seq information and identify genes engaged with the cell cycle.
Critically, the effectiveness of the technique was widely demonstrated in cell models from various organisms at various developmental stages. Given the variability in the cell cycle signatures among cell models, characterizing the cell cycle gradually works in RNA information-dependent exclusively upon gene markers lacks generalizability.
Later on, the use of gene markers should be supplanted by the adoption of techniques depending on dynamic features of gene expression, ready to accommodate changes in the cell cycle regulation.
At long last, the scientists believe that DeepCycle would turn into a fundamental tool for established researchers to additionally examine the cell cycle in a wide scope of frameworks without the requirement for cell synchronization or genetic tagging and complement the experimental methodologies that have been utilized in the past to unwind the regulation of the cell cycle.
This makes this methodology particularly appropriate for studying the interplay of the cell cycle with pluripotency and cell reprogramming. In addition, the comparison among typical and cancer tissues might prompt the discovery of cell-cycle dysregulated components in cancers and, maybe, potential targets for drug development.
Article Source: Riba, A., Oravecz, A., Durik, M. et al. Cell cycle gene regulation dynamics revealed by RNA velocity and deep-learning. Nat Commun 13, 2865 (2022). https://doi.org/10.1038/s41467-022-30545-8
Learn More:
Top Bioinformatics Books ↗
Learn more to get deeper insights into the field of bioinformatics.
Top Free Online Bioinformatics Courses ↗
Freely available courses to learn each and every aspect of bioinformatics.
Latest Bioinformatics Breakthroughs ↗
Stay updated with the latest discoveries in the field of bioinformatics.
Tanveen Kaur is a consulting intern at CBIRT, currently, she's pursuing post-graduation in Biotechnology from Shoolini University, Himachal Pradesh. Her interests primarily lay in researching the new advancements in the world of biotechnology and bioinformatics, having a dream of being one of the best researchers.
Nice work