Scientists from the University of California Irvine introduced SpaceFlow, which, by the utilization of spatially regularized deep graph networks, combines expression similarity with geographical information to provide spatially-consistent low-dimensional embeddings. Applications of SpaceFlow show evolving lineage in data on heart development and tumor-immune interactions in data on human breast cancer.
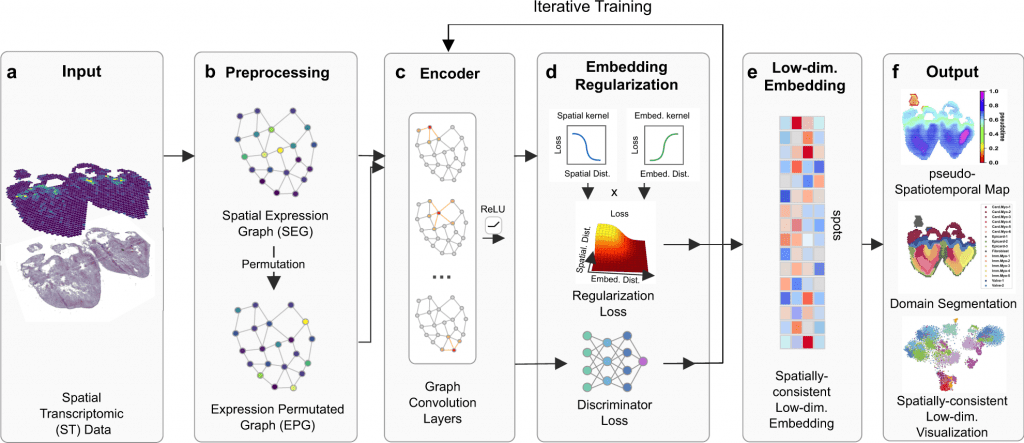
Image Source: https://doi.org/10.1038/s41467-022-31739-w
The simultaneous integration of cell transcriptome similarity and their spatial locations presents a significant barrier in the analysis of spatial transcriptomic datasets.
In this study, the scientists define their advanced tool SpaceFlow, in order to decipher the spatiotemporal patterns of the cells, the scientists presented a pseudo-spatiotemporal map based on the embedding, which combines the pseudotime idea with the geographical positions of the cells.
SpaceFlow has been depicted to provide a strong domain segmentation and uncover biologically significant spatiotemporal patterns by comparing with several existing approaches on various spatial transcriptomic datasets at both spot and single-cell resolutions.
The utilities of SpaceFlow show shifting lineage in data on heart development and tumor-immune interactions in data on human breast cancer. In order to analyze spatial transcriptome data, this study offers a customizable deep learning framework to incorporate spatiotemporal information.
Capturing Whole Transcriptomes with Spatial Barcoding-Based Methodologies
To understand important biological phenomena ranging from disease to embryonic development, it is essential to understand the spatiotemporal pattern of gene expression.
The widely used nonspatial single-cell RNA-sequencing approach is unable to quantify the gene expression using spatial information, but recent developments in spatially resolved transcriptomics (ST) technology offer new means to do so.
The bulk of contemporary ST technologies can be divided into two groups:
- Those that use spatial barcoding and
- In situ hybridization (ISH), with various levels of resolution and gene throughput.
By approximately 100–1000 and 10,000 genes, respectively, Multiplexed Error-Robust Fluorescence ISH (MERFISH) and Sequential Fluorescence ISH (seqFISH) are two ISH-based approaches that can detect target transcripts at the subcellular resolution.
With a variety of spatial spot resolutions, such as Visium in 55m, Slide-seq in 10m, and spatiotemporal enhanced resolution ‘omics sequencing (Stereo-seq) at nanometer (subcellular) resolution, spatial barcoding-based approaches can capture the whole transcriptome.
Requirement for New Computational Methods
The presence of spatial information in ST data necessitates the development of algorithms that natively manage the high-dimensional features in space as opposed to nonspatial technologies like scRNA-seq.
Previous research has focused chiefly on high-dimensional spatially aware analyses of image data.
Spatial transcriptomic data can be abstracted as high-dimensional images by treating each gene as a separate channel in an image.
However, new computational techniques created especially for transcriptome data are needed to reveal the biological relationships between genes in tissue.
Development of Methods for Identification of Spatial Domains in ST Data
In recasting the pertinent objectives in a spatial manner, several methods created for nonspatial transcriptomic data, such as scRNA-seq or bulk spatial transcriptomics data, may offer insights into building approaches for ST data at single-cell resolution.
As an illustration, it is possible to think of the discovery of spatially variable genes in ST data as the spatial expansion of the highly variable genes in scRNA-seq data.
Similar to cell clustering in scRNA-seq data analysis, techniques have been developed to identify spatial domains in ST data, which use spatial information to construct spatially coherent regions.
Markov random fields are used by Giotto, BayesSpace, and SC-MEB to simulate the associated gene expression in adjacent cells. Prior to clustering, stLearn performs spatial smoothing using morphological information.
Tissue domains are divided via graph partitioning by MULTILAYER. MERINGUE uses a weighted graph that combines geographical and transcriptional similarity to carry out graph-based clustering.
Deep auto-encoder networks are constructed using SpaGCN, SEDR, SCAN-IT, stMVC, and STAGATE to segment domains through embedding clustering and learn low-dimensional embeddings of both gene expression and spatial information.
The spatial retained graph autoencoder used by RESEPT to train a three-dimensional embedding from ST data treats the embedding like a three-dimensional image and uses a convolutional neural network to detect domains.
Requirement for Computational Tools for Integrative Reconstruction of Fine-resolution Spatiotemporal Trajectories
In the processing of scRNA-seq data, cell clustering is the ST equivalent of the domain segmentation techniques discussed above.
Contrary to discrete clustering, continuous pseudotime, which can describe developmental trajectories, is a powerful technique in scRNA-seq.
Many developmental systems, including regeneration and cancer progression, have spatially ordered dynamics.
Thus, the ST data offer a chance to concurrently disclose the development’s spatial and temporal features.
Although scRNA-seq pseudotime algorithms can be simply applied to ST data, the resulting trajectory might have gaps in space. Although stLearn filters connections between clusters inferred by scRNA-seq trajectory inference methods using a spatial distance cutoff and combines spatial distance with nonspatial pseudotime by simple average, the resulting connections are constrained by the initial pseudotime trajectories inferred without using spatial information.
Accordingly, there is a need for computational tools for the integrative reconstruction of fine-resolution spatiotemporal trajectories from ST data that are continuous in both time and space.
Various Computational Approaches in Utilization for ST Data
The computation of spatiotemporal trajectories can be seen as a problem of creating spatially aware embeddings of ST data because pseudotime trajectories are often derived using a low-dimensional embedding of transcriptome data.
There are several methods for computing spatially aware embeddings, including dual embedding, hierarchical SNE, and hierarchical UMAP.
In addition, deep graph neural network-based approaches, including DeepWalk, Variational Graph Auto-Encoder (VGAE), Graph2Gauss, and Deep Graph Infomax (DGI), have been used for ST data due to their flexibility to model and learn complex salient spatial dependencies between genes and cells. However, these methods are computationally more expensive.
Development of SpaceFlow
In this study, the scientists explained their developed framework to use ST data to show continuous temporal correlations with spatial context.
They constructed a pseudo-Spatiotemporal Map (pSM), which represented a spatially coherent pseudotime ordering of cells that encodes biological relationships between cells, along with a region segmentation using a DGI framework and spatial regularisation intended to capture both local and global structural patterns.
In order to demonstrate competitive performance on benchmarks, they compareed SpaceFlow with five other methods on six ST datasets.
The researchers then used SpaceFlow to reveal evolving cell lineage structures, spatiotemporal patterns, cell-cell communications, tumor-immune interfaces, and spatial dynamics of cancer progression.
The Endpoint
The scientists explained SpaceFlow in this study, which
- Converts the ST data into low-dimensional embeddings that indicate the geographic proximity of cells as well as expression similarity,
- A pseudo-Spatiotemporal Map (PSM) created from the embeddings incorporates the spatiotemporal associations of cells or spots in the ST data, and
- Identifies spatial domains with consistent expression patterns, clear boundaries, and less noise.
When compared to expert annotations, SpaceFlow outperforms alternative approaches in segmentation performance. Additionally, the pseudo-spatiotemporal patterns in tissue are revealed by the pSM using spatially consistent embeddings.
The pSM, which is not apparent from nonspatial pseudotime, displays layered patterns in the DLPFC and Stereo-seq data that are in line with the maturational sequences of the mouse olfactory bulb and the human cortex, respectively.
When applied to developmental data from the chicken heart, the pSM reveals changing lineage structures and reveals the dynamics in the spatiotemporal relationships of cells across various developmental stages, aiding in the understanding of changes in functional and structural organization in tissue development.
Using SpaceFlow to analyze ST data from human breast cancer, the scientists demonstrated that it has the ability to pinpoint tumor-immune interfaces and the dynamics of cancer growth, offering tools for investigating tumor evolution and interactions with the tumor microenvironment.
Although spatial proximity and similarity in gene expression are frequently associated60, this association is not always present.
Pseudotime techniques for scRNA-seq data, including Monocle and Slingshot, can result in spatially disorganized developmental trajectories. Based on the integrated use of spatial data and gene expression, the pSM created here may produce spatially contiguous trajectories.
Particularly, the low-dimensional embedding is spatially constrained by SpaceFlow’s spatial regularisation, resulting in an embedding that is continuous in both space and time.
Smoother domain segmentation borders and spatiotemporal maps are produced as a result of the low-dimensional spatial constraint, which also reduces noise in the high-dimensional gene expression data.
On ST data with fewer than 10,000 cells, SpaceFlow typically takes less than 5 minutes to train on a GPU. The calculation of the spatial regularisation loss for model optimization, which is quadratic to the number of cells or spots, determines a major portion of the computing cost of training.
The scientists calculated this regularisation loss over a random subset of cell-cell pairs to speed up model training.
The training can scale linearly with the number of cells or spots in the subset with a fixed number of cell pairs, and it has been demonstrated that this does not affect the result.
When a cell population higher than 10,000 is detected in the current implementation, the training will automatically transition to the approximated regularisation technique. For numbers of cells/spots ranging from 3000 to 50,000 on GeForce RTX 2080 Ti GPU, training time with this method varies from 30 seconds to 3 minutes.
Future research could investigate potential substitutes for choosing random subsets, like density-based subsampling, which might provide a more precise estimate of the regularisation loss.
SpaceFlow is a versatile framework that can include supplementary characteristics concerning cell connection among cells in spatial or single-cell omics data in addition to spatial regularisation.
For instance, it can be utilized immediately with spatial graph input based on 3D coordinates for 3D ST data. The framework for geographically resolved epigenetic data could be modified in the future to include suitable preprocessing procedures, such as peak calling on spatially resolved chromatin modification data.
The robustness of the SpaceFlow embeddings could be increased by using additional non-genomic data modalities, such as local texture features from histology images or expert domain annotation priors.
Various regularisation terms indicate different prior knowledge about the organization of the tissue within the SpaceFlow framework, and their integration may improve the performance of the outcome.
Additionally, the low-dimensional embeddings consistent with RNA velocity might be derived using the directed connection matrix inferred by RNA velocity as a constraint, enhancing the representation of developmental trajectory.
Overall, SpaceFlow offers an excellent tool and a solid framework for incorporating previous knowledge or spatial limitations into ST data processing for the inference of spatiotemporal patterns of cells in tissues.
Article Source: Ren, H., Walker, B.L., Cang, Z. et al. Identifying multicellular spatiotemporal organization of cells with SpaceFlow. Nat Commun 13, 4076 (2022). https://doi.org/10.1038/s41467-022-31739-w
Learn More About Bioinformatics:
Top Bioinformatics Books ↗
Learn more to get deeper insights into the field of bioinformatics.
Top Free Online Bioinformatics Courses ↗
Freely available courses to learn each and every aspect of bioinformatics.
Latest Bioinformatics Breakthroughs ↗
Stay updated with the latest discoveries in the field of bioinformatics.
Tanveen Kaur is a consulting intern at CBIRT, currently, she's pursuing post-graduation in Biotechnology from Shoolini University, Himachal Pradesh. Her interests primarily lay in researching the new advancements in the world of biotechnology and bioinformatics, having a dream of being one of the best researchers.