As we approach 2025, the integration of artificial intelligence (AI) into bioinformatics is set to transform the field dramatically. Bioinformaticians must stay abreast of several key trends that will shape research methodologies, data analysis, drug discovery, and personalized medicine. Here are the top AI trends to watch that will significantly impact bioinformatics in the coming year.
#1 Quantum AI: Solving Biology’s Most Complex Puzzles
Quantum AI represents a groundbreaking convergence of quantum computing and artificial intelligence. This innovative field leverages the principles of quantum mechanics to solve complex problems that traditional AI struggles with. For instance, pharmaceutical companies like Roche are collaborating with quantum computing firms to utilize quantum simulations for drug discovery, enabling more accurate modeling of molecular interactions than classical computers can achieve. Additionally, algorithms such as the Quantum Support Vector Machine (QSVM) and Quantum Neural Networks (QNN) are being developed to enhance machine learning models, potentially leading to faster and more precise predictions in healthcare.
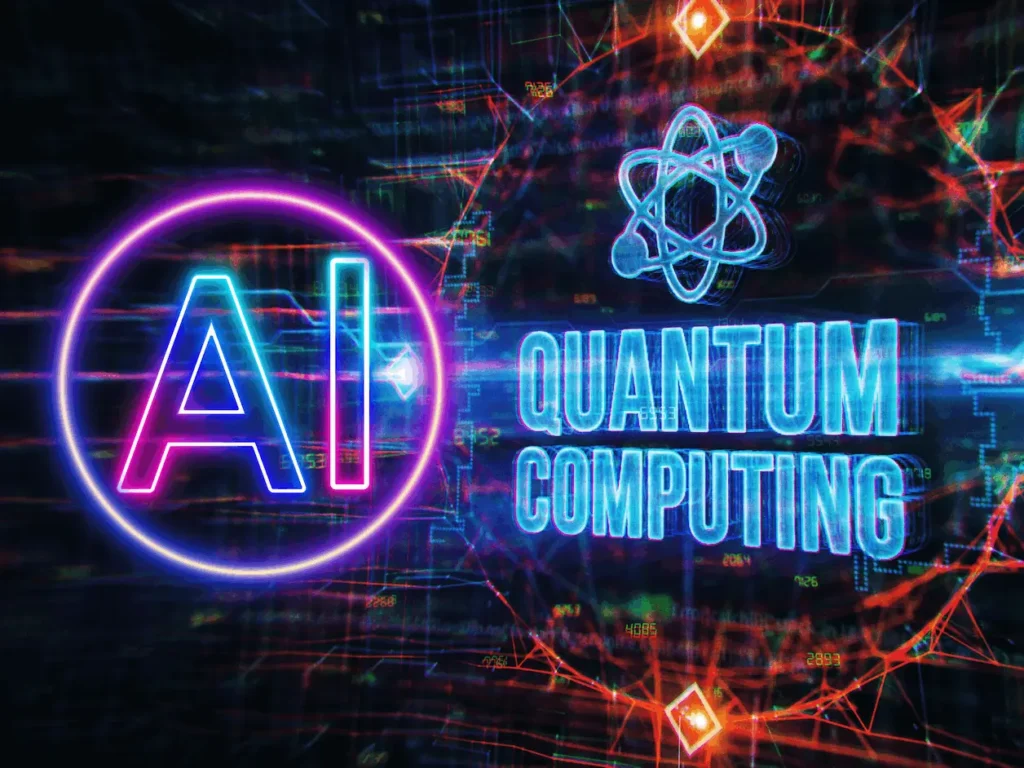
#2 Generative AI in Drug Discovery: From Months to Minutes
Generative AI is rapidly evolving from a niche technology into a critical tool across various sectors, including healthcare. In bioinformatics, generative models can create synthetic datasets for training purposes and generate hypotheses for biological experiments. For example, researchers at Stanford University have developed generative models that can predict protein structures based on genetic sequences, significantly speeding up the process of understanding protein functions and interactions.
Generative models, particularly Variational Autoencoders (VAEs) and Generative Adversarial Networks (GANs), are being utilized to design novel drug-like molecules with specific properties. For instance, researchers have employed GANs to generate molecules that exhibit high binding affinity to target proteins while minimizing toxicity. This approach accelerates the drug discovery process by enabling the rapid screening of potential candidates. Example: Insilico Medicine uses GANs to design novel compounds for drug development, significantly shortening the time required for lead discovery.
Google DeepMind’s AlphaFold has been enhanced with generative capabilities to predict protein structures and design new proteins that can bind effectively to target molecules.
#3 Multimodal AI: The Universal Translator of Biological Data
Imagine an AI that can seamlessly integrate genomic sequences, protein structures, clinical records, and medical imaging into a single, coherent narrative. Multimodal AI models are breaking down the traditional silos of biological research, creating unprecedented insights that were impossible just years ago. This approach allows for a more comprehensive understanding of patient health by leveraging diverse data sources.
Microsoft Research has developed multimodal generative AI frameworks to create high-fidelity patient embeddings using diverse patient data. BiomedJourney uses multimodal patient data for disease progression prediction. NVIDIA BioNeMo provides customizable AI models for various biomolecular tasks by integrating multimodal data such as protein sequences and molecular docking simulations (e.g., DiffDock). It facilitates the prediction of 3D protein structures and enables the generation of novel molecules tailored for specific properties. Evolutionary Scale Modeling (ESM) utilizes multimodal approaches by combining sequence information with structural predictions to improve understanding of protein function and drug design processes. MONAI (Medical Open Network for AI) focuses on medical imaging but also incorporates multimodal capabilities by integrating imaging data with clinical records and genomic information to enhance diagnostic accuracy and treatment planning.
#4 Explainable AI (XAI): Bringing Transparency to Complex Algorithms
As AI systems become more complex, the need for transparency in their decision-making processes grows. Explainable AI aims to make AI outputs understandable to humans. A notable example is the use of XAI techniques in clinical settings where machine learning models assist in diagnosing diseases from genomic data. For instance, IBM’s Watson Health employs XAI principles to provide insights into its diagnostic recommendations, allowing healthcare professionals to understand the rationale behind its suggestions and build trust in its outputs.
#5 Edge AI: Healthcare’s New Frontier
Edge AI refers to deploying AI algorithms on local devices rather than relying on centralized cloud computing. This trend is particularly relevant in bioinformatics for real-time data processing at the source. For example, wearable health devices equipped with Edge AI can analyze biometric data instantly, providing immediate feedback to users regarding their health status. Companies like Fitbit are integrating Edge AI capabilities to enhance user experience by offering real-time health insights without relying on cloud connections.
The Human Element: AI as a Collaborator, Not a Replacement
Let’s be crystal clear: AI isn’t here to replace bioinformaticians. It’s here to supercharge human creativity and scientific intuition. The most successful researchers in 2025 will be those who learn to dance with AI, not compete against it.
Are You Ready for the Future?
The landscape of bioinformatics is evolving swiftly with the advent of advanced artificial intelligence technologies. Bioinformaticians must be proactive in understanding these trends to remain at the forefront of research innovation. By embracing these developments and their real-world applications, professionals in the field can enhance their analytical capabilities and contribute significantly to advancements in healthcare and biotechnology.
FAQs
Quantum AI leverages quantum computing to solve complex biological problems more efficiently than traditional methods. It can enhance drug discovery processes by enabling more accurate simulations of molecular interactions and accelerating the analysis of large genomic datasets.
Generative AI can create synthetic datasets for training purposes and generate hypotheses for biological experiments. It is used to design novel drug-like molecules and predict protein structures, significantly speeding up the understanding of protein functions and interactions.
Explainable AI (XAI) is crucial because it provides transparency in the decision-making processes of AI systems. This transparency helps bioinformaticians trust and understand AI-generated insights, which is particularly important in clinical settings where decisions can impact patient care.
Edge AI refers to deploying AI algorithms on local devices for real-time data processing rather than relying on cloud computing. In bioinformatics, this allows for immediate analysis of biometric data from wearable devices, enhancing user experience and enabling timely health insights.
Multimodal AI integrates multiple data types — such as imaging, genomic sequences, and electronic health records — to improve clinical decision-making. Examples include NVIDIA BioNeMo for biomolecular tasks, Evolutionary Scale Modeling (ESM) for protein function understanding, and MONAI for medical imaging integration.
Bioinformaticians should stay informed about advancements in AI technologies through continuous education and training. Collaborating with interdisciplinary teams and adopting new tools will also help them leverage these trends effectively in their research and practice.
Story Sources:
- Nałęcz-Charkiewicz K, Charkiewicz K, Nowak RM. Quantum computing in bioinformatics: a systematic review mapping. Brief Bioinform. 2024 Jul 25;25(5):bbae391. doi: 10.1093/bib/bbae391. PMID: 39140856; PMCID: PMC11323091.
- Kösoglu-Kind, B., Loredo, R., Grossi, M. et al. A biological sequence comparison algorithm using quantum computers. Sci Rep 13, 14552 (2023). https://doi.org/10.1038/s41598-023-41086-5
- Generative modeling for protein structures
- CQC, Roche Partner to Use Quantum Algorithms to Tackle Drug Discovery for Alzheimer’s Disease
- Multimodal Generative AI: the Next Frontier in Precision Health
Follow Us!
Learn More:
Dr. Tamanna Anwar is a Scientist and Co-founder of the Centre of Bioinformatics Research and Technology (CBIRT). She is a passionate bioinformatics scientist and a visionary entrepreneur. Dr. Tamanna has worked as a Young Scientist at Jawaharlal Nehru University, New Delhi. She has also worked as a Postdoctoral Fellow at the University of Saskatchewan, Canada. She has several scientific research publications in high-impact research journals. Her latest endeavor is the development of a platform that acts as a one-stop solution for all bioinformatics related information as well as developing a bioinformatics news portal to report cutting-edge bioinformatics breakthroughs.